Question
Part A- The data have information from box scores from all 82 regular season basketball games played by the Miami Heat NBA team in the
Part A- The data have information from box scores from all 82 regular season basketball games played by the Miami Heat NBA team in the 2010-11 season. Multiple regression output is shown for predicting the number of points scored by the Miami Heat in a game based on the number of rebounds, steals, blocks, and assists in the game.
How might we proceed if we want to try to improve this model?
- Remove the variable Rebounds from the model.
- Remove the Constant from the model.
- Remove the variable Steals from the model.
- Remove the variable Blocks from the model.
- Remove the variable Assists from the model.
Part B- A quantitatively savvy, young couple is interested in purchasing a home in northern New York. They collected data on 48 houses that had recently sold in the area. They want to predict the selling price of homes (in thousands of dollars) based on the size of the home (in square feet).
The regression equation is Price (in thousands) = 17.1 + 0.0643 Size (sq. ft.)
Predictor | Coef | SE Coef | T | P |
Constant | 17.06 | 24.59 | 0.69 | 0.491 |
Size (sq. ft.) | 0.06427 | 0.01224 | 5.25 | 0.000 |
S = 48.5733 R-Sq = 37.5% R-Sq(adj) = 36.1%
Predicted Values for New Observations
Size (sq. ft.) | Fit | SE Fit | 95% CI | 95% PI |
2000 | 145.61 | 7.07 | (131.38, 159.83) | (46.80, 244.41) |
Use the equation of the least squares line to predict the selling price of a home that is 1,742 square feet in size.
A. $143,773
B. $129,111
C. $115,582
D. $299,000
E. $201.873
Part C- A dataset shows the number of capital letters that a student can write in 15 seconds using the non-dominant hand (X) versus the same thing for the dominant hand (Y). The data is for 63 students. Here is computer output:
Model Summary
S | R-sq | R-sq(adj) | R-sq(pred) |
5.05128 | 35.69% | 34.64% | 31.49% |
Coefficients
Term | Coef | SE Coef | T-Value | P-Value | VIF |
Constant | 22.69 | 2.08 | 10.92 | 0.000 | |
nondom | 0.876 | 0.151 | 5.82 | 0.000 | 1.00 |
Regression Equation
dom | = | 22.69 + 0.876 nondom |
Using the information in the Coefficients table above, make a decision on the hypothesis test to see if we have a statistically significant linear relationship, in this case. The correct response also includes a description of what that means.
- Reject the null hypothesis, this is not a statistically significant linear relationship.
- Reject the null hypothesis, this is a statistically significant linear relationship.
- Fail to reject the null hypothesis, this is not a statistically significant linear relationship.
- Fail to reject the null hypothesis, this is a statistically significant linear relationship.
Step by Step Solution
There are 3 Steps involved in it
Step: 1
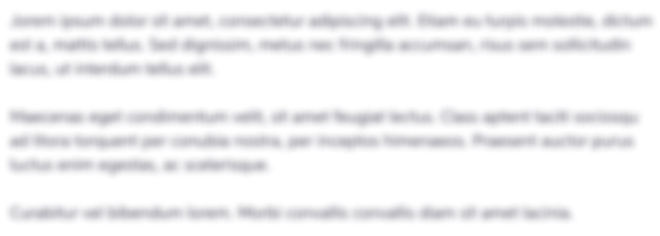
Get Instant Access with AI-Powered Solutions
See step-by-step solutions with expert insights and AI powered tools for academic success
Step: 2

Step: 3

Ace Your Homework with AI
Get the answers you need in no time with our AI-driven, step-by-step assistance
Get Started