Please answer using R code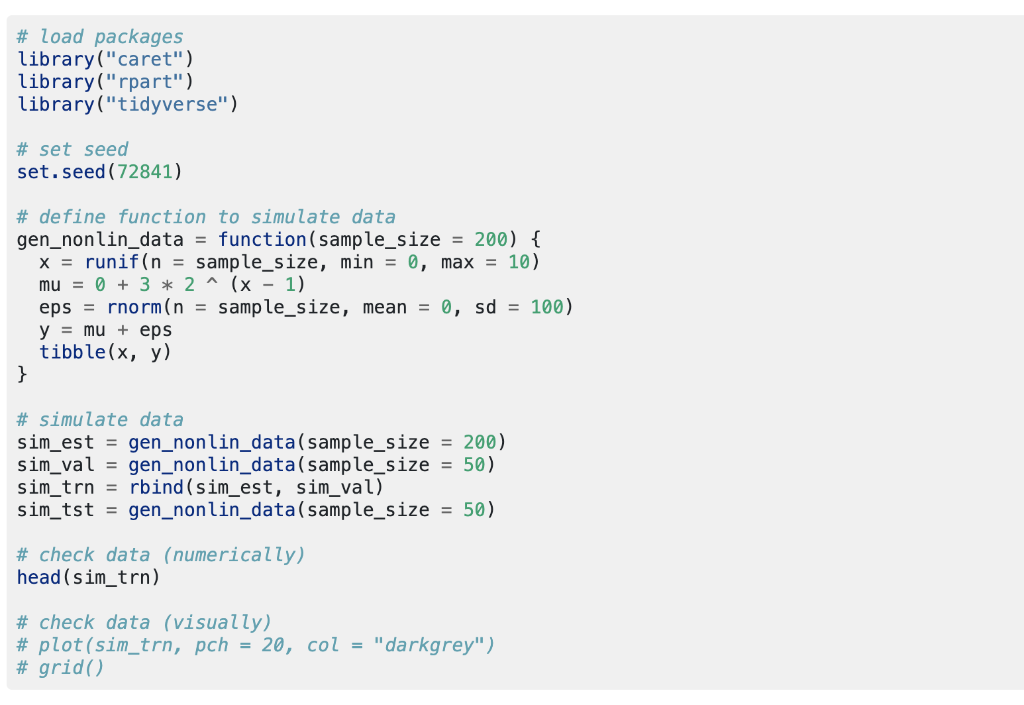
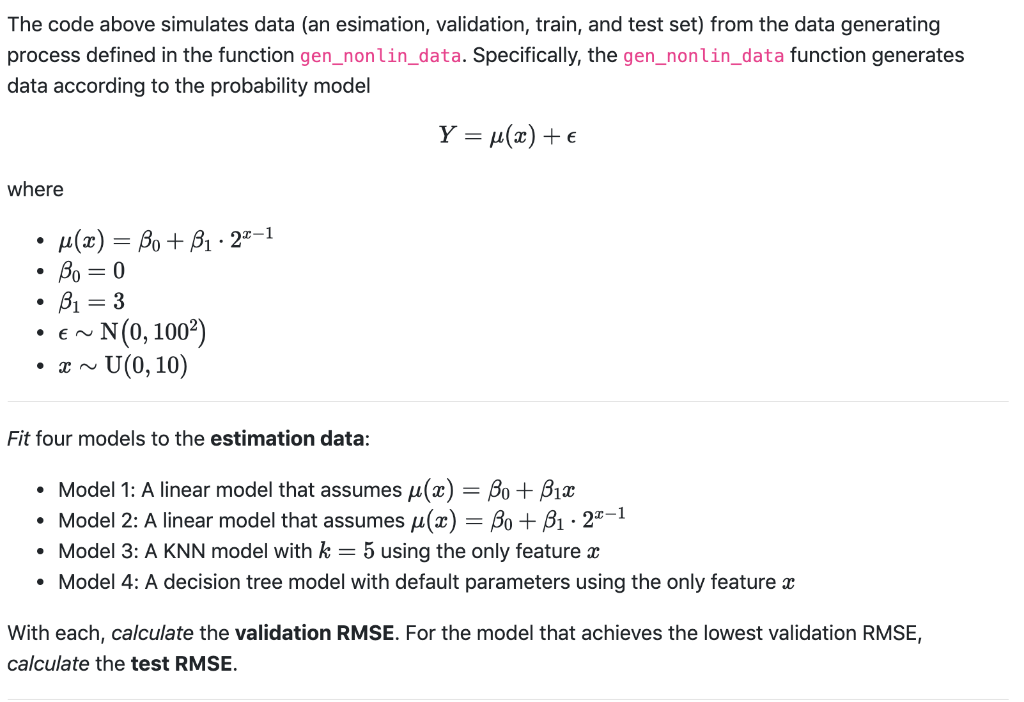
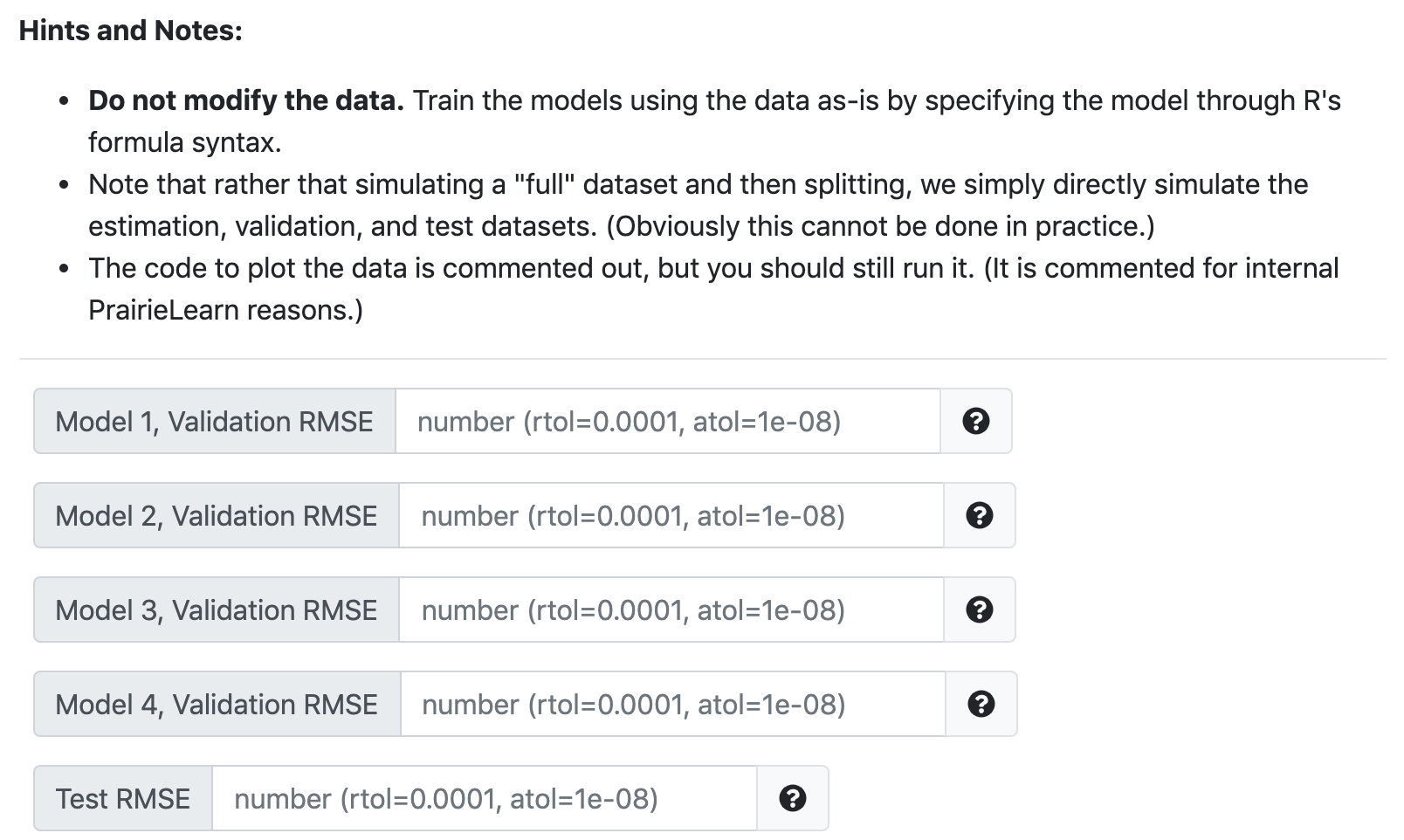
# load packages library("caret") library("rpart") library("tidyverse") # set seed set.seed ( 72841) # define function to simulate data gen_nonlin_data = function( sample_size = 200) { x = runif(n = sample_size, min = 0, max = 10) mu = 0 + 3 * 2 ^ (x - 1) eps = rnorm(n = sample_size, mean = 0, sd = 100) y = mu + eps tibble(x, y) } # simulate data sim_est = gen_nonlin_data(sample_size = 200) sim_val = gen_nonlin_data(sample_size = 50) sim_trn = rbind(sim_est, sim_val) sim_tst = gen_nonlin_data(sample_size = 50) # check data (numerically) head(sim_trn) # check data (visually) # plot(sim_trn, pch = 20, col = "darkgrey") # grid() The code above simulates data (an esimation, validation, train, and test set) from the data generating process defined in the function gen_nonlin_data. Specifically, the gen_nonlin_data function generates data according to the probability model Y = u(x) + where M(x) = Bo +31: 22-1 Bo=0 Bu = 3 N(0, 100) U(0, 10) EN Fit four models to the estimation data: Model 1: A linear model that assumes u(x) = Bo + B1x Model 2: A linear model that assumes u(x) = Bo + B1 22-1 Model 3: A KNN model with k = 5 using the only feature x Model 4: A decision tree model with default parameters using the only feature x . With each, calculate the validation RMSE. For the model that achieves the lowest validation RMSE, calculate the test RMSE. Hints and Notes: Do not modify the data. Train the models using the data as-is by specifying the model through R's formula syntax. Note that rather that simulating a "full" dataset and then splitting, we simply directly simulate the estimation, validation, and test datasets. (Obviously this cannot be done in practice.) The code to plot the data is commented out, but you should still run it. (It is commented for internal PrairieLearn reasons.) Model 1, Validation RMSE number (rtol=0.0001, atol=1e-08) Model 2, Validation RMSE number (rtol=0.0001, atol=1e-08) e Model 3, Validation RMSE number (rtol=0.0001, atol=1e-08) Model 4, Validation RMSE number (rtol=0.0001, atol=1e-08) Test RMSE number (rtol=0.0001, atol=1e-08) ? # load packages library("caret") library("rpart") library("tidyverse") # set seed set.seed ( 72841) # define function to simulate data gen_nonlin_data = function( sample_size = 200) { x = runif(n = sample_size, min = 0, max = 10) mu = 0 + 3 * 2 ^ (x - 1) eps = rnorm(n = sample_size, mean = 0, sd = 100) y = mu + eps tibble(x, y) } # simulate data sim_est = gen_nonlin_data(sample_size = 200) sim_val = gen_nonlin_data(sample_size = 50) sim_trn = rbind(sim_est, sim_val) sim_tst = gen_nonlin_data(sample_size = 50) # check data (numerically) head(sim_trn) # check data (visually) # plot(sim_trn, pch = 20, col = "darkgrey") # grid() The code above simulates data (an esimation, validation, train, and test set) from the data generating process defined in the function gen_nonlin_data. Specifically, the gen_nonlin_data function generates data according to the probability model Y = u(x) + where M(x) = Bo +31: 22-1 Bo=0 Bu = 3 N(0, 100) U(0, 10) EN Fit four models to the estimation data: Model 1: A linear model that assumes u(x) = Bo + B1x Model 2: A linear model that assumes u(x) = Bo + B1 22-1 Model 3: A KNN model with k = 5 using the only feature x Model 4: A decision tree model with default parameters using the only feature x . With each, calculate the validation RMSE. For the model that achieves the lowest validation RMSE, calculate the test RMSE. Hints and Notes: Do not modify the data. Train the models using the data as-is by specifying the model through R's formula syntax. Note that rather that simulating a "full" dataset and then splitting, we simply directly simulate the estimation, validation, and test datasets. (Obviously this cannot be done in practice.) The code to plot the data is commented out, but you should still run it. (It is commented for internal PrairieLearn reasons.) Model 1, Validation RMSE number (rtol=0.0001, atol=1e-08) Model 2, Validation RMSE number (rtol=0.0001, atol=1e-08) e Model 3, Validation RMSE number (rtol=0.0001, atol=1e-08) Model 4, Validation RMSE number (rtol=0.0001, atol=1e-08) Test RMSE number (rtol=0.0001, atol=1e-08)