Question
Please find the classification of correlation type for the data and come to a conclusion The data used is about civil aviation of Canadian air
Please find the classification of correlation type for the data and come to a conclusion
The data used is about civil aviation of Canadian air carriers.
Two data sources where used and they where matched to each other using the year.
Data source for Income:
https://www150.statcan.gc.ca/t1/tbl1/en/tv.action?pid=2310003401
Data source for passengers and goods
https://www150.statcan.gc.ca/t1/tbl1/en/tv.action?pid=2310003301
Compiled Data from two data source
(The maximum data available in the given site was from 2012 to 2020)
Year | Passengers (x1000) | Goods (x1000) | Revenue |
2012 | 66867 | 747275 | 20343071 |
2013 | 67991 | 720872 | 20816397 |
2014 | 72236 | 736764 | 22309270 |
2015 | 76216 | 696491 | 22832738 |
2016 | 81872 | 758425 | 23230889 |
2017 | 88416 | 881191 | 25548635 |
2018 | 93337 | 969457 | 27970817 |
2019 | 94132 | 979161 | 29493379 |
2020 | 28437 | 936903 | 12223233 |
Thedependent variableis theRevenue.This is selected as the main dependent variable is because the final objective of any aviation company is to maximise it's revenue. Also, the revenue is depended on other factors.
TheIndependent VariablesarePassengersandGoods. It is the basic understanding that the number of passengers and goods transported by a carrier an independent factor. Even though marketing and other activities can have some influence in improving or reducing these counts, at the end it is the customer who decides the counts of this. Also, the revenue of a carrier is very highly depended on the number of passengers and goods it transport.
Passengers (x1000) | Goods (x1000) | Revenue | |
Mean | 74389.33 | 825171 | 22752047.67 |
Median | 76216 | 758425 | 22832738 |
Variance | 402329912.5 | 13241135863 | 25167463074589 |
Standard Deviation | 20058.16 | 115070.13 | 5016718.36 |
Max | 94132 | 979161 | 29493379 |
Min | 28437 | 696491 | 12223233 |
Standard deviation gives the measure of spread of data from the mean. we can see this in a better way if we find 2*SD as a percentage of the total range.
Passengers (x1000) | Goods (x1000) | Revenue | |
2*SD | 40116.32 | 230140.26 | 10033436.72 |
2*SD/(Max-Min) (%) | 61.06 | 81.42 | 58.1 |
Analysis on central tendency
- Passengers
- The mean and median are similar, suggesting a higher central tendency
- The standard deviation and the 2SD/range suggest that the spread of passengers is not too less nor too much.
- This suggest that the passenger distribution is cantered around the mean of 74389.33 with a reasonable spread
- Goods
- The goods have a slightly different median and mean.
- The standard deviation is also quite high suggesting a very wide spread in the goods count.
- From the difference in mean and median and the very high spread it can be said that the goods is almost uniformly distributed. That is the central tendency is less.
- Revenue
- The mean and median of revenue is very similar
- The standard deviation of the revenue a bit too high
- The revenue tend to have a good central tendency with a high spread.
Explanation:
Correlation | Passengers | Goods |
Revenue | 0.981623104 | 0.2506799405 |
The correlation of Revenue with Passenger is close to positive 1. This suggest a strong positive correlation. This means the higher the number of passenger the higher the revenue will get.
The correlation of revenue with Goods is very week positive. This suggest that the change in goods wont have a significant effect in revenue. The slight effect that the goods number have is, when goods increases the revenue increases slightly.
Regression Analysis
Passenger and Revenue
Regression Statistics | |
Multiple R | 0.981623104 |
R Square | 0.9635839183 |
Adjusted R Square | 0.9583816209 |
Standard Error | 1023439.797 |
Observations | 9 |
ANOVA | |||||
df | SS | MS | F | Significance F | |
Regression | 1 | 194007701464798 | 194007701464798 | 185.2227673 | 0.000002720850255 |
Residual | 7 | 7332003131912 | 1047429018845 | ||
Total | 8 | 201339704596710 |
Coefficients | Standard Error | |
Intercept | 4488548.173 | 1384635.247 |
Passengers (x1000) | 245.5123426 | 18.03956854 |
This means the equation of revenue if revenue( y) and passenger( x) will be
y=245.51x+4488548.17
Here the intercept is 4488548.173. This is the revenue even when there is no passengers. The coefficient of passenger that is 245.51 is the average increase in revenue per 1000 passenger.
Goods
Regression Statistics | |
Multiple R | 0.2506799405 |
R Square | 0.06284043257 |
Adjusted R Square | -0.07103950563 |
Standard Error | 5191853.928 |
Observations | 9 |
ANOVA | |||||
df | SS | MS | F | Significance F | |
Regression | 1 | 12652274130936 | 12652274130936 | 0.4693790079 | 0.5153113164 |
Residual | 7 | 188687430465774 | 26955347209396 | ||
Total | 8 | 201339704596710 |
Coefficients | Standard Error | |
Intercept | 13733831.55 | 13276397.87 |
Goods (x1000) | 10.92890579 | 15.95198934 |
This means the equation of revenue if revenue( y) and goods( x) will be
y=10.93x+13733831.55
Here the intercept is 13733831.55 .This is the revenue even when there is no goods. The coefficient of goods that is 10.93 is the average increase in revenue per 1000 goods.
Passenger | ||
Observation | Predicted Revenue | Absolute Residual |
1 | 20905221.99 | 562150.9879 |
2 | 21181177.86 | 364780.8611 |
3 | 22223377.76 | 85892.24447 |
4 | 23200516.88 | 367778.8792 |
5 | 24589134.69 | 1358245.689 |
6 | 26195767.46 | 647132.4593 |
7 | 27403933.7 | 566883.3026 |
8 | 27599116.01 | 1894262.99 |
9 | 11470182.66 | 753050.3395 |
Goods | ||
Observation | Predicted Revenue | Absolute Residual |
1 | 21900729.62 | 1557658.621 |
2 | 21612173.72 | 795776.722 |
3 | 21785855.89 | 523414.1073 |
4 | 21345716.07 | 1487021.93 |
5 | 22022586.92 | 1208302.079 |
6 | 23364284.97 | 2184350.031 |
7 | 24328935.77 | 3641881.233 |
8 | 24434989.87 | 5058389.131 |
9 | 23973156.17 | 11749923.17 |
The highest absolute residual points in Passenger regression is the 8th observation corresponding to year 2019 and in case of goods regression it is 9th observation corresponding to 2020.
let us remove both of them and perform a new regression (regression statistics for this will only be given in the link of google sheet provided as this platform cannot support too much text here)
New Regression after removing the high residual entry
Passenger
New Equation: y=230.38x+5340168.80
Goods
New Equation: y=27x+2163309.77
Both the regression improved after removing these points.
From the analysis we can conclude that the passenger count have the highest influence on the revenue with a high positive correlation value of 0.981623104 while the goods count have very little influence on revenue. with a very week correlation of 0.2506799405.
Link to the sheets with calculations
Link1: https://docs.google.com/spreadsheets/d/1NycpvIEaCE6oSU0A4y38HPwBHDZJz7UaPkqJ8vpqTXM/edit?usp=sharing
Link2: https://docs.google.com/spreadsheets/d/1J_SznEL8_vjJxGbSddktKNYYyXF8gmf9h6t3Au0oKjg/edit?usp=sharing
Link 3:
https://docs.google.com/spreadsheets/d/13W49cMwuVCfrJ4eQW_m_K7h8y5PfHUXgWi0Gk9DiBxg/edit?usp=sharing
Step by Step Solution
There are 3 Steps involved in it
Step: 1
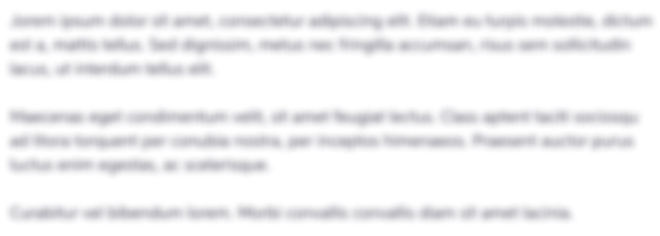
Get Instant Access to Expert-Tailored Solutions
See step-by-step solutions with expert insights and AI powered tools for academic success
Step: 2

Step: 3

Ace Your Homework with AI
Get the answers you need in no time with our AI-driven, step-by-step assistance
Get Started