Answered step by step
Verified Expert Solution
Question
1 Approved Answer
Please show steps. Thanks 2. Table 1 is a summary of the predictive attributes of a car evaluation dataset. The dataset has totally 1296 records.
Please show steps. Thanks
2. Table 1 is a summary of the predictive attributes of a car evaluation dataset. The dataset has totally 1296 records. The class attribute is acceptability of car, which has four class categories: unacceptable, acceptable, good, and very good. Table 2 summarizes the frequency counts of each value of each predictive attribute with respect to each class category. a) (20 Points) Calculate the information gain of each predictive attribute if it is used as the first split attribute Table 1 Summary of Predictive Attributes of Car Evaluation Dataset Predictive Attribute Meaning Value Buying purchasing price categorical (very high, high, med, low) Maint maintenance price categorical (very high, high, med, low) doors number of doors categorical (2,3,4,5 or more) persons capacity in terms of persons to carry categorical (2, 4, more) lug_boot the size of luggage boot categorical (small, med, big) safety estimated safety of the car categorical (low, med, high) Table 2 Frequency counts of each value of each predictive attribute with respect to each class category Class: acceptability Predictive Attribute unacceptable acceptable good very good very high 269 high 246 buying med 202 low maintenance very high high med low doors 5 or more 249 225 222 212 439 228 241 persons more 143 small med lug_boot big 289 low safety med high 199 156 2. Table 1 is a summary of the predictive attributes of a car evaluation dataset. The dataset has totally 1296 records. The class attribute is acceptability of car, which has four class categories: unacceptable, acceptable, good, and very good. Table 2 summarizes the frequency counts of each value of each predictive attribute with respect to each class category. a) (20 Points) Calculate the information gain of each predictive attribute if it is used as the first split attribute Table 1 Summary of Predictive Attributes of Car Evaluation Dataset Predictive Attribute Meaning Value Buying purchasing price categorical (very high, high, med, low) Maint maintenance price categorical (very high, high, med, low) doors number of doors categorical (2,3,4,5 or more) persons capacity in terms of persons to carry categorical (2, 4, more) lug_boot the size of luggage boot categorical (small, med, big) safety estimated safety of the car categorical (low, med, high) Table 2 Frequency counts of each value of each predictive attribute with respect to each class category Class: acceptability Predictive Attribute unacceptable acceptable good very good very high 269 high 246 buying med 202 low maintenance very high high med low doors 5 or more 249 225 222 212 439 228 241 persons more 143 small med lug_boot big 289 low safety med high 199 156
Please show steps.
Thanks



Step by Step Solution
There are 3 Steps involved in it
Step: 1
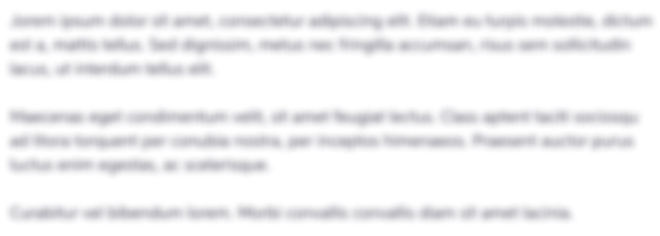
Get Instant Access with AI-Powered Solutions
See step-by-step solutions with expert insights and AI powered tools for academic success
Step: 2

Step: 3

Ace Your Homework with AI
Get the answers you need in no time with our AI-driven, step-by-step assistance
Get Started