Answered step by step
Verified Expert Solution
Question
1 Approved Answer
Please use jupyter notebook application departmen status agesalary count sales sales senior 31..3546k..50k 30 junior 26.3026k..30k40 sales junior 31.3531k..35k40 systemsjunior 21..2546k..50k20 systemssenio31..3566k..70k5 systemsjunior 26..3046k..50k3 systems
Please use jupyter notebook application
Step by Step Solution
There are 3 Steps involved in it
Step: 1
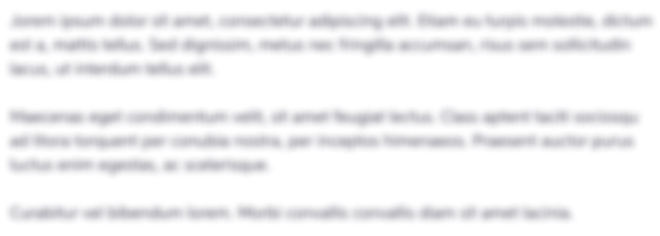
Get Instant Access to Expert-Tailored Solutions
See step-by-step solutions with expert insights and AI powered tools for academic success
Step: 2

Step: 3

Ace Your Homework with AI
Get the answers you need in no time with our AI-driven, step-by-step assistance
Get Started