Answered step by step
Verified Expert Solution
Question
1 Approved Answer
Problem Description You are tasked with developing a Q - learning agent to solve a grid world environment using reinforcement learning and Python. The grid
Problem Description You are tasked with developing a Qlearning agent to solve a grid world environment using reinforcement learning and Python. The grid world is represented as a x grid, and the agent must navigate through it avoiding obstacles, and reach the terminal state to receive a reward. Grid World Configuration and Rules The grid world is a x matrix bounded by borders. The agent starts from cell second row, first column The agent has four possible actions: North action code: South action code: East action code: West action code: The agent receives a reward of if it reaches the terminal state cell blue cell There is a special jump from cell to cell with a reward of The agent is blocked by obstacles black cells QLearning Approach Qlearning is a modelfree reinforcement learning algorithm that learns an actionvalue function Qvalues for each stateaction pair. Heres how you can approach this task: Initialization: Initialize the Qvalues for all stateaction pairs to arbitrary values eg zeros Set the learning rate alpha and discount factor gamma Exploration and Exploitation: Exploration: The agent explores different actions to discover the environment. Use an exploration strategy egepsigreedy to choose actions randomly with some probability. Exploitation: The agent exploits the learned Qvalues to choose the best action based on the current state. QValue Update: Update the Qvalues using the Qlearning update rule:QsaQsaalpha rsagamma amaxQsaQsa where: s is the current state. a is the chosen action. s is the next state after taking action ars a is the immediate reward for taking action a in state salpha is the learning rate. gamma is the discount factor. Training the Agent: Run episodes where the agent interacts with the environment. Update Qvalues based on observed rewards and transitions. Continue until convergence or a maximum number of episodes. Policy Extraction: Extract the policy optimal action for each state from the learned Qvalues. Use the policy to navigate the agent through the grid world. Remember to handle special cases eg the jump from cell to appropriately in your implementation.
Step by Step Solution
There are 3 Steps involved in it
Step: 1
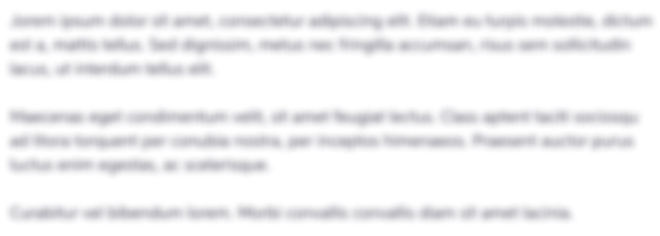
Get Instant Access to Expert-Tailored Solutions
See step-by-step solutions with expert insights and AI powered tools for academic success
Step: 2

Step: 3

Ace Your Homework with AI
Get the answers you need in no time with our AI-driven, step-by-step assistance
Get Started