Answered step by step
Verified Expert Solution
Question
1 Approved Answer
Q2.4 Using Nested Cross validation, find the best hyperparameter Use the Decision Tree Classifier class to build a decision tree inside of a 5-fold cross
Q2.4 Using Nested Cross validation, find the best hyperparameter Use the Decision Tree Classifier class to build a decision tree inside of a 5-fold cross validation loop. The partitions you alread- loop. In the inside loop you should use 4-fold cross validation (so you don't have to partition again) to find the best value for m the Gini Index as your impurity measure. Calculate the mean accuracy across the 4 folds of your inner loop for all the candidat values, and print the value. Use the array np.array([0.1,0.25,0.3,0.4]) as the candidates for the best hyperparameter. min_impurity decrease values give the same highest accuracy), choose the lowest min_impurity decrease value. For each inner loop, select the best min_impurity decrease and train the outer fold training data on using that value. For each of the 5 executions of the inner loop, your output should look something like this: Testing 0.10 min impurity decrease Average accuracy over 4 folds is 0.95 Testing 0.25 min impurity decrease Average accuracy over 4 folds is 0.86 Testing 0.30 min impurity decrease Average accuracy over 4 folds is 0.63 Testing 0.40 min impurity decrease Average accuracy over 4 folds is 0.27 Best min impurity decrease is 0.1 possible_min_impurity_decrease = np.array([0.1,0.25,0.3, 0.4]) Cross validation, find the best hyperparameter Elass to build a decision tree inside of a 5-fold cross validation loop. The partitions you already created in 2.2 will be the outer use 4-fold cross validation (so you don't have to partition again) to find the best value for min_impurity_decrease. Use easure. Calculate the mean accuracy across the 4 folds of your inner loop for all the candidate min_impurity decrease he array np.array([0.1,0.25,0.3,0.4]) as the candidates for the best hyperparameter. If there is a tie (two Es give the same highest accuracy), choose the lowest min impurity_decrease value. st min_impurity decrease and train the outer fold training data on using that value. e inner loop, your output should look something like this: ty decrease er 4 folds is 0.95 ty decrease er 4 folds is 0.86 ty decrease er 4 folds is 0.63 ty decrease er 4 folds is 0.27 -ase is 0.1 ease = np.array([0.1,0.25,0.3,0.4]) possible ain_impurity_decrease - np.array([0.1,0.25,0.3,0.4]) merge the folds def serge_folds(curr_fold); for 1 in range(1, k1): what format should the merged data be in * -df_X[df X['K Assignment')- curr_fold) y - s_y[df_x['K Assignment') curr_fold) Wreturn the merged training data (both x and y) return xy Outer Loop outer_acc - np.array(11) for k in dict k_df X.keys(): for pos_nin_impurity in possible_win_impurity decrease: # Inner Loop cross validation code here (use 4 folds, where the fold does not include for each min (npurity decrease value se decision tree to get accuracy #use gint index for impurity measure gini_ind - tree. DecisionTreeclassifier criterion - "gial', max_depth - k) giniind.fit (dict_k_of_x[k], dict_k syk]) calculate the accuracy based on the decision tree * Calculate the neon accuracy across the 4 folds of your inner loop for all the candidate was impurity decrease values, this acc -get_acc() total acc this acc nean_ace - total acc/4 print('Testing pos_min_Inpurity.'nin inpurity decrease") print("Average accuracy over 4 folds is, mean acc) choose the best impurity decrease value based on the highest avg if min impurity decrease > mean acc: min_impurity decrease - mean_acc train tree using best in impurity decrease & 4 other folds training predictions_gini - gins_Ind.predict(possible_min_impurity_decrease[k]) wuse test data to see how well the tree does (1) outer accuracy atculation outer acc - np.append(outer acc, this acc) . make sure and calculate thisce in your loop print('best sin impurity decrease is', win_impurity decrease) Q2.4 Using Nested Cross validation, find the best hyperparameter Use the Decision Tree Classifier class to build a decision tree inside of a 5-fold cross validation loop. The partitions you alread- loop. In the inside loop you should use 4-fold cross validation (so you don't have to partition again) to find the best value for m the Gini Index as your impurity measure. Calculate the mean accuracy across the 4 folds of your inner loop for all the candidat values, and print the value. Use the array np.array([0.1,0.25,0.3,0.4]) as the candidates for the best hyperparameter. min_impurity decrease values give the same highest accuracy), choose the lowest min_impurity decrease value. For each inner loop, select the best min_impurity decrease and train the outer fold training data on using that value. For each of the 5 executions of the inner loop, your output should look something like this: Testing 0.10 min impurity decrease Average accuracy over 4 folds is 0.95 Testing 0.25 min impurity decrease Average accuracy over 4 folds is 0.86 Testing 0.30 min impurity decrease Average accuracy over 4 folds is 0.63 Testing 0.40 min impurity decrease Average accuracy over 4 folds is 0.27 Best min impurity decrease is 0.1 possible_min_impurity_decrease = np.array([0.1,0.25,0.3, 0.4]) Cross validation, find the best hyperparameter Elass to build a decision tree inside of a 5-fold cross validation loop. The partitions you already created in 2.2 will be the outer use 4-fold cross validation (so you don't have to partition again) to find the best value for min_impurity_decrease. Use easure. Calculate the mean accuracy across the 4 folds of your inner loop for all the candidate min_impurity decrease he array np.array([0.1,0.25,0.3,0.4]) as the candidates for the best hyperparameter. If there is a tie (two Es give the same highest accuracy), choose the lowest min impurity_decrease value. st min_impurity decrease and train the outer fold training data on using that value. e inner loop, your output should look something like this: ty decrease er 4 folds is 0.95 ty decrease er 4 folds is 0.86 ty decrease er 4 folds is 0.63 ty decrease er 4 folds is 0.27 -ase is 0.1 ease = np.array([0.1,0.25,0.3,0.4]) possible ain_impurity_decrease - np.array([0.1,0.25,0.3,0.4]) merge the folds def serge_folds(curr_fold); for 1 in range(1, k1): what format should the merged data be in * -df_X[df X['K Assignment')- curr_fold) y - s_y[df_x['K Assignment') curr_fold) Wreturn the merged training data (both x and y) return xy Outer Loop outer_acc - np.array(11) for k in dict k_df X.keys(): for pos_nin_impurity in possible_win_impurity decrease: # Inner Loop cross validation code here (use 4 folds, where the fold does not include for each min (npurity decrease value se decision tree to get accuracy #use gint index for impurity measure gini_ind - tree. DecisionTreeclassifier criterion - "gial', max_depth - k) giniind.fit (dict_k_of_x[k], dict_k syk]) calculate the accuracy based on the decision tree * Calculate the neon accuracy across the 4 folds of your inner loop for all the candidate was impurity decrease values, this acc -get_acc() total acc this acc nean_ace - total acc/4 print('Testing pos_min_Inpurity.'nin inpurity decrease") print("Average accuracy over 4 folds is, mean acc) choose the best impurity decrease value based on the highest avg if min impurity decrease > mean acc: min_impurity decrease - mean_acc train tree using best in impurity decrease & 4 other folds training predictions_gini - gins_Ind.predict(possible_min_impurity_decrease[k]) wuse test data to see how well the tree does (1) outer accuracy atculation outer acc - np.append(outer acc, this acc) . make sure and calculate thisce in your loop print('best sin impurity decrease is', win_impurity decrease)
Step by Step Solution
There are 3 Steps involved in it
Step: 1
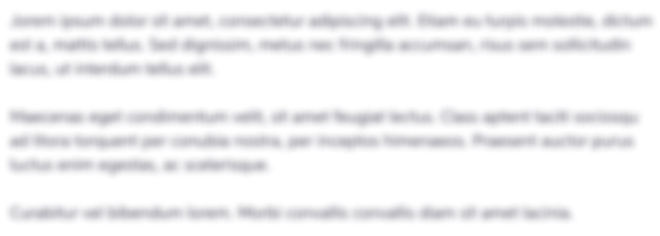
Get Instant Access to Expert-Tailored Solutions
See step-by-step solutions with expert insights and AI powered tools for academic success
Step: 2

Step: 3

Ace Your Homework with AI
Get the answers you need in no time with our AI-driven, step-by-step assistance
Get Started