Question
Question 1 [100 Marks]You decide to work as an academic staff in a university. Other than research ability,academic administrators pay attention to teaching quality in
Question 1 [100 Marks]You decide to work as an academic staff in a university. Other than research ability,academic administrators pay attention to teaching quality in setting salaries. You wouldlike to know how some ascriptive characteristics, such as beauty, affect the instructor'sratings by students. You are given a dataset containing professor characteristics for 463courses for the academic years 20002002 at the University of Texas at Austin. Theresponse variable is teaching evaluation scores (eval) and the predictors are ratings ofthe instructor's physical appearance measured by a score (beauty), age (age), numberof students that participated the evaluation (student), number of students enrolled inthe course (allstudents), whether the instructor is male or female (gender), whetherthe instructor is from a minority group (minority), whether the instructor is on tenuretrack (tenure), and whether the instructor is a native English speaker (native).In this assignment, we would like to use some of these variables to try and build amultiple regression model with eval as the response variable. Use R to further analysethe "teach" data (available on Wattle) and answer the following questions:(a) [6 marks] First identify which variables are numeric in this dataset and fit a multiple linear regression (MLR) model with eval as the response variable and all othernumeric variables as predictors. Present the main residual plot of the residualsagainst the fitted values for this model. Are there are any obvious problems withunderlying assumptions?(b) [10 marks] It is not very difficult to see that eval is always positive (ranges from0 to 5), so it would be worth trying to transform the variable such as the logtransformation. Now fit a MLR model with ln(eval) as the response variable, stillusing all the other numeric variables (not log transformed) as explanatory variables.Again present the main residual plot of the residuals against the fitted values forthis new model. Comment on this new residual plot. Then, test whether this modelis significant.(c) [12 marks] What are the estimated coefficients of the MLR model in part (b) andthe standard errors associated with these coefficients? Interpret the values of eachof the estimated coefficients with regards to model specification. Construct 95%Bonferroni joint confidence intervals for all the slope parameters. Comment on thet-test results in the summary output.(d) [12 marks] Produce both a scatterplot matrix and a correlation matrix for thepredictors included in the model and comment on any important relationshipsbetween the variables. Do you see a problem with this MLR model as in part(b)? Conduct a diagnostic check quantitatively to determine the severity of thisparticular problem. What could be done to solve this problem?(e) [12 marks] You have now discussed this problem with the administrators and theysuggest only to include age and beauty as potential predictors in the model. However, you doubt the importance of the variable age. You are not sure what kind ofmarginal relationship is between age and the response ln(eval), given that beautyis already included in the model. Generate an appropriate plot to visually check thisrelationship and comment on the plot. Then conduct a partial F-test to determinewhether age is a significant addition to a model that already includes beauty.(f) [8 marks] The administrators remind you that a native English speaker and a nonnative English speaker tend to have a different eval. Therefore, you want toknow how does the variable native affect the response ln(eval). Conduct a testof whether a native English speaker has higher eval than a non-native Englishspeaker by fitting a simple linear regression model. Then provide a 95% confidenceinterval on the slope coefficient and interpret this interval.(g) [6 marks] Finally, given above findings, you decide to fit a MLR model with ln(eval)as the response variable and with beauty and native as predictor. Conduct a t-testfor beauty in this model.(h) [16 marks] Using the model in part (g), produce a plot of externally studentizedresiduals against fitted values, a normal QQ plot, a leverage plot, a Cook's distanceplot and a number of DFBETAs plots for all the slope coefficients in your model.Comment on the model assumptions and unusual points.(i) [8 marks] Generate a scatter plot of eval (in its original scale) against beauty,using different color for native and non-native speaking instructors. Use the modelfrom part (g) to predict the expected eval for both native and non-native speakinginstructors over the full range of possible beauty measurements and include theseon your plot as two different curves (using different color or line types). Includeappropriate titles, axis labels, a legend and a brief discussion of your plot.(j) [10 marks] With the model in part (g), we now consider adding the interactionterm between beauty and native. Before adding the interation, generate a scatterplot of ln(eval) (in log scale) against beauty, using different color for native andnon-native speakers. Add fitted lines (using the model in part (g)) for native andnon-native speakers in a different color (or a different line type). Comment on theplot whether there is a visible interaction. Then add the interation into the modelin part (g) and test whether the interaction is significant.
Step by Step Solution
There are 3 Steps involved in it
Step: 1
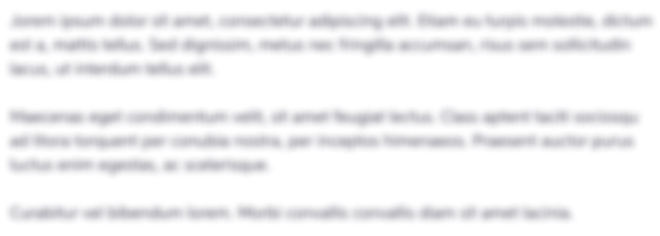
Get Instant Access to Expert-Tailored Solutions
See step-by-step solutions with expert insights and AI powered tools for academic success
Step: 2

Step: 3

Ace Your Homework with AI
Get the answers you need in no time with our AI-driven, step-by-step assistance
Get Started