Question
Q_wc = np.array([[1,0.3],[0.3,1]]); q = np.array([0,0]); Q_sic = np.array([[1,0.85],[0.85,1]]); q = np.array([0,0]); Q_ic = np.array([[1,0.99],[0.99,1]]); q = np.array([0,0]); PartA Consider the quadratic functions f_wc, f_sic
Q_wc = np.array([[1,0.3],[0.3,1]]); q = np.array([0,0]); Q_sic = np.array([[1,0.85],[0.85,1]]); q = np.array([0,0]); Q_ic = np.array([[1,0.99],[0.99,1]]); q = np.array([0,0]);
PartA Consider the quadratic functions f_wc, f_sic and f_ic defined by the quadratic matrices above. For each of these, say whether they are -smooth and/or -stronglyconvex, and if so, compute the value of thecondition number, k=/ for each function.
PartB Compute the best fixed step size for gradient descent, and the best parameters for accelerated gradient descent. For each function, plot the error (_t)() as a function of the number of iterations. For each function, plot these on the same plot so you can compare -- so you should have 3 plots total.
Problem 1: Gradient Descent and Acceleration In this problem you will explore the impact of ill-conditioning on gradient descent, and will then see how acceleration can improve the situation. This accelerated gradient descent as the condition number (ratio of largest to smallest eigenvalues of the Hessian) increases. This is a "toy" problem, but is still instructive regarding the performance of these two algorithms. You will work with the following simple function: f(x)=21xQx, where Q is a 2 by 2 matrix, as defined belowStep by Step Solution
There are 3 Steps involved in it
Step: 1
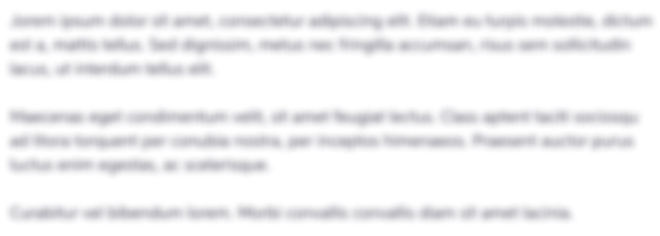
Get Instant Access to Expert-Tailored Solutions
See step-by-step solutions with expert insights and AI powered tools for academic success
Step: 2

Step: 3

Ace Your Homework with AI
Get the answers you need in no time with our AI-driven, step-by-step assistance
Get Started