Answered step by step
Verified Expert Solution
Question
1 Approved Answer
RESEARCH DESIGN We have already discussed potential problems with measurement: reliability, internal validity, and external validity. Internal validity and external validity can also apply to
RESEARCH DESIGN We have already discussed potential problems with measurement: reliability, internal validity, and external validity. Internal validity and external validity can also apply to designs, i.e., how we test our hypotheses in the real world. Just as with measurements, it is virtually impossible to create a test situation (design) totally free of internal and external validity problems. Before I elaborate, let's make the distinction clear. IV stands for hypothesized independent variable; DV is the hypothesized outcome or dependent variable. The distinction is much the same as we made for measurement problems: INTERNAL VALIDITYis the change in the IV the cause of the change in the DV or is it something else? DESIGN: EXTERNAL VALIDITYIV causes DV, but perhaps only given certain other conditions, only for a certain subpopulationhow generalizable are our results? The best way to determine whether potential validity problems exist is to first look at what a perfect experimental design entails. If anything is missing, then we know that our conclusions might be suspect (i.e., the test doesn't really confirm our hypothesis). A true or classical experimental design can be mapped out as follows. Test R: Y1t Control R: Y1c X Y2t ~X Y2c X is the treatment or independent variable. It needs to vary between the test and control (comparison) groups. It can, as in the example, be the presence (X) or absence (~X) of an independent stimulus or treatment, or different levels of that treatment. In medical research, for example, the \"treatment\" is either a drug (given only to the test group) or different levels of a drug (in either instance, variation exists between the two groups). You might already be thinking of a problem. Individuals might get better just because they take a pill (a psychological response), regardless of whether or not the compound in it actually causes the benefit (a physiological response). This is why in most medical research a \"placebo\" is given to the control groupa pill with the same shape, color and taste as the real drug but without the tested medicinal properties. By doing this, the effects of the medicine, as opposed to just taking the pill, can be isolated. Y is the outcome or dependent variable. In our design we measure it for both groups (t=test, c=control or comparison) and before (time=1) and after (time=2) the treatment is administered (or, for our research as an example, before and after a policy is implemented). As you all learned when we discussed hypothesis formation, a comparison must be madethus we need at least two groups to compare. The trick, and the hardest condition in our research, is to make sure that the two groups are exactly the same on every important variable except for the difference on X. Remember that, in discussing measurement problems, we decided that any tested group needed to be a random or equiprobable sample of the target population. How can we accomplish this with designs? One method is by a matching procedure. We try to figure out every possible alternative cause of a difference in outcome. With medical research this might be health, age, gender, race, etc. Although this is often the method employed when the test and control groups are small, it is not preferred. First, it's very difficult to match on every posited alternate variable (each group must have the same age, health, gender, race, etc. breakdown). More importantly, however, is that we can't know for sure whether we equally parceled out individuals or cases on every variable that might bias our experiment. We are not deities, and therefore can't be sure that other important properties might exist that are important in determining the usefulness of a treatment (drug/policy) but that we are not even aware exist. What if there is a human property called \"zenotone\" which influences the usefulness of a drug? This might sound silly, but remember, we didn't even know about DNA and its difference among individuals a half century ago. Yet it is critical in understanding both the implications of medical treatments as well as providing excellent data for determining guilt in certain crimes. How can we possibly equalize out the effects of \"zenotone\" when we can't measure it because we don't know it exists? Just as with measurement, we can do so by randomly assigning individuals into the treatment and control group. If done properly and with a large enough original sample, each group will have the same proportion of individuals with every \"zenotone\" level. In order to do so, each case in the experiment must have an exactly equal chance (50/50)of being selected for either the test (drug is given) or control (placebo is given) group. If done perfectly, then a \"premeasurement\" or \"before measurement,\" Y1t and Y1c is not even necessarythey should break down the same for each group. We often do the premeasurement, however, as a way of testing whether or not we were successful in our random assignment process. Two other conditions, often not mentioned in the political science literature should also be met in order for us to have a true experiment: 1. The experiment should be \"double blind.\" This means that whether or not a group is being given the treatment (drug) should not be known to those taking the drug or placebo (otherwise different psychological reactions might occur) AND to those measuring outcomes (otherwise it might influence how they perceive those outcomes). Every medical practitioner wants to find a cure, especially if their continued funding depends upon it. 2. No \"crosscontamination.\" This means that there can be no \"spill over\" effects from one group to another. Usually we don't concern ourselves with this because cross contamination will tend to reduce the probability that we will see the hypothesized differences. Those on a placebo might get a psychological boost just by seeing their friends get better. If the test group still shows even more improvement than the control, then we can be even more certain that the drug works as intended. On the other hand, what if the \"placebo\" group gets depressed because they realize that they are not getting better as they notice some of their friends are? This might tend to exaggerate the effects of the drug. At this point, you should all realize the difficulty of conducting a true experiment in political science. Most of what we study has already occurred, with individuals or states or countries deciding on their own whether or not they will be part of a cosmic test or control group. Even if we are looking at the present or future, we can't force some states, e.g., to try a new education program and others not to. They will choose on their own short of any financial inducements from the federal government. Even field experiments like those employed by Gerber and Green might suffer from a crosscontamination problem and (external validity) might not be generalizable outside of a particular town for a particular election at a particular point in time. So, how do we even begin to do research? We go back to our Law and Order scenario. First, we try to examine the possible confounding elements in our designthe alternate explanations. Can we find ways to isolate them? Let's go back to our \"Spock\" example (the baby doctor, not the pointy eared alien). If we think that radicalism increased because of the existence of an unpopular war (Vietnam) rather than the prior use of permissive child rearing practices, then we should try to find periods when unpopular wars did not follow more permissive child rearing practices, as well as peaceful periods that did. Of course, this is not always possible. Another, often times unavoidable problem comes with some public policy equivalent of cross contamination. If we try out a new educational method for some students in a school and not others, the latter might either learn from the former (and thus make it more difficult for us to see a measurable difference) or get ticked off by what they see as a comparative disadvantage (and the difference might get exaggerated). Solution? Try to find two school districts separated by hundreds of miles that are as similar as possible on every known characteristic that can affect learning. Employ the new teaching method in one but not the other. Problem? How do we guarantee that the two are exactly the same on every important characteristic? What if they have different proportional levels of the unknown property \"zenotone\"? The best we can hope for is to do the best we can do, control for the most obvious alternate explanations, and measure and test in as many ways as possible. Each test, as with each type of measurement, will have a potential internal or external validity problem. We can't control for all of them but, if each test has a different type of validity problem yet the results always come out as anticipated, then we are much more confident in our conclusion (although never fully sure). Again, this is called \"triangulation\" or \"triangulization.\" What's Missing Can Often Point Out What is potentially suspect: Let's look at the following examples that fall short of a classical experiment: I. Single group longitudinal studyno separate randomly assigned control; same group is compared against itself over time: Test R: Y1t Control R: Y1c X Y2t ~X Y2c Potential problems with design (the list is not exhaustivesee readings and following set of examples): INTERNAL VALIDITY: 1. Historyother events could have occurred between times 1 and 2 that might have caused the change. The longer the time period between these two points, the more likely history may pose a potential problem. 2. Test sensitizationparticularly in surveys, individuals might recall from the first measurement (Y1t) what they got right and what they got wrong. The original measurement is what really causes the change. 3. Hawthorne effectthe group in question may be responding to being watched, not the treatment (X) itself. Students might study harder, e.g., to please the teacher (yeah, right). The new teaching method itself may be of limited usefulness. 4. Experimental mortalityoriginally coined as a phrase to describe what happens to results over a long longitudinal study when some people actually die off (perhaps those who were most ill). This can also be applied to selective withdrawal from the test. What if those who are not getting better after administration of a drug are more likely to drop out than those who are? The results at time 2 might be higher only because of the selfselection out of the experiment. Grades at the end of a quarter will always be, on \"average\" better than grades at the beginning. Did students actually learn? Perhaps. But the group being measured is not the same after as before the class was conducted. Students who fail a first exam often drop out, leaving behind better qualified students. We would need to look at individual scores to see if those who remained actually improved. But then we might not be able to generalize the benefits of the teaching method to all students. 5. Instrumentationdoes the way we measure change between the two points in time. Does it become more or less accurate. As much as we would like to think otherwise, public officials might want to be able to demonstrate that the programs for which they received funds had the intended effects if only to make political points or guarantee ongoing funding. Example: many social scientists believe that the much touted drop in crime in NYC under the Giuliani mayoral administration (and to a lesser extent, the current Bloomberg administration) had as much to do with the aging of the NYC population (older individuals are less likely to commit a vast variety of crimes than younger people) and economic changes (less crime during periods of economic expansion). Now there is also evidence that the data might have been \"cooked\" to show a decrease in crime greater than that which actually occurred. See: (http://www.nytimes.com/2010/02/09/nyregion/09mayor.html?scp=2&sq=crime%20nyc&st=cse) Sometimes the instrumentation change might be inadvertent. The reported incidence of domestic abuse often goes up after programs to counsel women about domestic abuse have been instituted. Do the programs lead to more abuse, or does the reporting become more accurate when women feel safer in reporting problems and police officials are more sensitive to them? 6. Regression artifactfrom the statistical term \"regression to the mean.\" Are the measurements at the two points in time unusual (say unusually high at one point, unusually low at another)? Usually any type of measurement will fluctuate on a day by day basis. Is the drop consistent or just part of a sawtooth pattern? EXTERNAL VALIDITY: 1. selectionexperimentation interactionis there anything unique about the group being tested that might make them more likely to benefit from the treatment than any group chosen at random? We call this an \"external validity\" problem because the program, drug, etc. does work, but only for certain types of subjects. The problem is with subject generalizability. 2. Testsensitizationmight the premeasurement have affected the subjects ability or willingness to change? For example, if I give an exam at the beginning of a course, students might be sensitized to what information they are deficient on. They will then pay more attention when the class turns to that information. The class does help, but might not have without the initial testing (again, situational generalizability) II. . Independent but Noncomparable group comparison without premeasurement: Test R: Y1t Control R: Y1c X Y2t ~X Y2c Internal Validity's Main Problem: 1. Spuriousnessmight another variable (Z) be causing both which group assigns itself to the treatment and the outcome? We have no way of knowing if the differences always existed without a premeasurement or observation. For all we know, the test group measurement might have gone down while the \"control's\" went up but with the hypothesized difference still observable. X Z Y III. Independent but Noncomparable group comparison with premeasurement: Test R: Y1t Control R: Y1c X Y2t ~X Y2c Even if the premeasurement is the same, selfselection into test and control might still pose a problem. All of the problems in example I could still be lurking. Let us say that one group of students decides to take a Political science class during an election year and one doesn't. Let us also imagine that they start of with the same level of knowledge but only the PoliSci group improves measurably. These problems, and more, might still be influencing the results: 1. SelectionHistoryNon PoliSci students might be less interested in watching the news (politics). PoliSci students are learning from that, not the class. 2. SelectionHawthorne/Instrumentation?why should non PoliSci students care about my feelings? 3. SelectionTestingwhy should they care to remember? 4. SelectionExperimental Mortality - why would non PoliSci students drop out? 5. Etc. Example: School funding and educational achievement: The amount spent per pupil by schools in some education districts is much higher than in others. The higher the amount spent, the better students tend to perform on standardized exams, as reported by the school boards in the community. We can therefore conclude that spending money improves the overall quality of student education. DESIGN: Test: R: Y1t X Y2t Control: R: Y1c ~X Y2c Independent but nonrandom/equivalent control; no premeasurement Four Potential Problems: 1. Big one: (self)selection biasSES of communities that spend more might have other reasons for higher scores (more parents with college educations and high incomes). This can produce a spurious relationship where the SES status of a community influences both the amount of money parents can and are willing to spend on their schools and how well off their children are even without that spending. 2. measurement internal validityare standardized exams useful in measuring \"educational quality\"? 3. instrumentationmight the better funded school boards be padding the results to demonstrate that money did work? 4. maybe selectionhistory interaction : money might have also been spent in those communities on libraries, tutoring, etc.not just class per pupil ratios. This is called \"selectionhistory\" because although all communities go through history at the same time, different events might not be affecting both equally. IV = school spending per pupil DV=educational quality (or standardized scores as measured) U of A: education districts
Step by Step Solution
There are 3 Steps involved in it
Step: 1
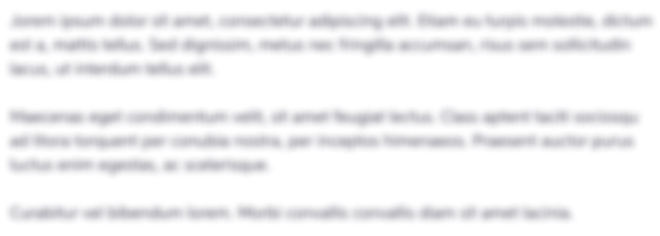
Get Instant Access to Expert-Tailored Solutions
See step-by-step solutions with expert insights and AI powered tools for academic success
Step: 2

Step: 3

Ace Your Homework with AI
Get the answers you need in no time with our AI-driven, step-by-step assistance
Get Started