Answered step by step
Verified Expert Solution
Question
...
1 Approved Answer
RYLEE SMITH Investment manager Rylee Smith was thinking about the meeting she had just held with her team at Glitz Investments, an investment firm based
RYLEE SMITH Investment manager Rylee Smith was thinking about the meeting she had just held with her team at Glitz Investments, an investment firm based in the United States that focused on the entertainment industry. Specifically, Smith was pondering the comments that her fellow investment manager had made: Were forgoing a lucrative opportunity by not investing in the east. Some international movie industries are seeing high growth rates. It will be a mistake to not shift our investment focus to that region. Specifically, Ive gathered some information on Bollywoodthe Indian movie industry and Rylee, I think you should look over it. Weve got some potential movies lined up, we just have to choose one if we decide to give this project a go. Smith knew that investing in the Indian film industry for the first time would be a risk for Glitz Investments. However, such a move, if executed properly, could become a critical turning point for the company. Another colleague who had attended the meeting agreed. This could be a great opportunity for us, stated the colleague. Capitalizing on these trends could provide us with access to an untapped market. From the research that was given to her, Smith understood that if Glitz Investments wanted to expand its investment focus geographically, Bollywood would be the perfect place to begin. The main challenge was choosing the right movies as investment vehicles. Although it was an attractive industry for investment, many movie projects across the world resulted in failure. Smith decided that she had to gain a better understanding of the unique features of the Indian movie industry. Smith studied the report that the analysts at the firm had compiled for her (see Exhibits 1 and 2). She noticed that the team had collected data only for movies with a budget of 100 million1 or higher, which was consistent with the firms policy to invest in movies with a minimum level of production quality. She wondered if she could determine a quantitative relationship between box office performance and the factors that the analysts had identified. Could Glitz Investments selectively choose movies based on Smiths analysis? Half an hour later, she had created a couple of log linear regression models (see Exhibits 3 and 4). It was time to share some conclusions with her boss, Carl Irving. Smith reached for her phone and called Irving. CARL IRVING On the other end of the phone line, Smiths boss listened carefully to her conclusions before replying, This is good Rylee, but I want something more. It shows how we can improve our average return but I want an analysis that guarantees or at least improves the odds that we strike a jackpot Bollywood movie. Smith shook her head to herself and spoke into her phone: If there was such a thing, Carl, then everyone would be using that magic formula to guarantee sky-high ROIs [returns on investment]. Irving replied with his own assessment of the situation: Of course, thats a given. But come on, in a world where analytics is providing so many different ways to look at data, surely something as straightforward as log linear regression cannot be the only analytical technique we can use. I want more refined analysis and I want it by the end of this week. I mean, look at the trends of the Bollywood market. The 1B Club of box office earnings is going to be superseded by a new 2 billion benchmark. Thats what I want to identifya 2 billion movie that sets the precedent for forthcoming blockbusters. Nothing else. And without warning, Smith heard the phone click on the other end. What was she going to do? And how was she going to further dissect the analysis she had conducted? Smith felt frustrated, but as she logged into Facebook and started to mindlessly go through her newsfeed, something caught her eye. A former classmate from her master of business administration (MBA) studies had posted a new status. More important than the Facebook status was the name of the classmate that caught her attention. Ian Thomas had been widely known as the analytics wizard of the MBA program. If anyone could expertly interpret the Bollywood data, it was him. Without hesitating, Smith picked up her phone and called Thomas. IAN THOMAS Thomas agreed to meet with Smith two days later at a nearby coffee shop. After exchanging pleasantries, the two former classmates got down to business. Okay so show me what youve got, stated Thomas. Smith reached for her notes and started explaining the situation: Definitely. Heres what Ive done already. I performed a log linear regression between the net gross of Bollywood movies over the past five years and the following factors: budget, number of screens, and star factor. While I found significant relationships between these factors and the net gross, this information is not enough. My boss wants more insight on this information. The thing is that he wants to invest in a movie that is not only going to be a success but a game changer for the industryone that has the potential to break all the Indian box office records. I just dont know what else to do with this information to answer his question. Frankly, Im not sure if it can be answered at all. Hmmm. Lets see. Do you remember the concept we learned at schoolquantile regression? asked Thomas. Oh yeah. I do remember that concept. Quantile regression helps us model the relationship between an independent variable X and the specific percentiles or quantiles of the response variable Y. I also recall that quantile regression is especially useful to identify relationships in markets where outcomes are highly uncertain, as is the case with many cultural industries. My word, it will be perfect for our Bollywood data. Exactly. Averages dont mean much in the film industry because producers and investors care only about blockbusters. No wonder your boss is so antsy about hitting the jackpot. Well hes antsy in general, so dont make excuses for him. Do you want to apply quantile regression to the data? asked Smith. Yeah, definitely, confirmed Thomas. Smith and Thomas got to work. A few hours later, they had the output from their analysis in front of them (see Exhibit 4). Okay, Thomas started, now that we have performed further analysis using quantile regression, its time to understand what all these numbers mean. Hopefully, by the end of today, youll be able to give your boss some insights. EXHIBIT 1: INDIAN FILM INDUSTRY ANALYSIS REPORT The Bollywood Report Based in Mumbai, the Indian movie industry, known widely as Bollywood, is one of the fastest growing movie industries in the world. In 2013, the value of the entire industry was estimated at 125 billion. Each year, box office milestones are created by Bollywood moviesnot only in India, but across the world. The domestic film industry market was expected to grow at an annual compound annual growth rate (CAGR) of 11.4 per cent until 2018, while the overseas market was projected to grow at a CAGR of 8.9 per cent. Two other significant revenue streams for Bollywood movie production companies are cable and satellite television rights and ancillary revenue streams. Cable and satellite television rights were expected to grow at an annual rate of 10.7 per cent, whereas ancillary revenue streams were expected to grow at a rate of 24.7 per cent. The 1B Club In 2008, the Indian movie industry released a blockbuster movie that earned a staggering 1.14 billion in domestic revenues. This marked the creation of the coveted 1B Club, which consisted of movies that earned over 1 billion domestically. Year over year, an increasing number of movies recorded earnings above that benchmark. Currently, 25 movies hold a place in the 1B Club. Analysts predict that the 1B Club will eventually be replaced by a 2B Club, as more movies break box office records set by predecessors. Hollywoods Interest in Bollywood Foreign investment in the Indian movie industry is not new. Many Hollywood production companies have already entered Bollywood, contributing to this lucrative industry. For example, 20th Century Fox Film Corporation entered the industry as a joint venture and later acquired the leading Indian media and entertainment company Star India Private Limited to distribute and produce over 25 Indian movies. Similarly, Viacom started a joint venture with the India-based media company Network 18. Disney has a majority stake in UTV Software Communications, one of the top Indian movie production companies. Source: KPMG, The Stage Is Set: FICCI-KPMG Indian Media and Entertainment Industry Report, 2014, accessed May 31, 2019, https://assets.kpmg/content/dam/kpmg/pdf/2014/03/FICCI-Frames-2014-The-stage-is-set-Report-2014.pdf; Nyay Bhushan, Disney Acquires Controlling Stake in Indias UTV, The Hollywood Reporter, February 1, 2012, accessed May 31, 2019, www.hollywoodreporter.com/news/disney-acquires-controlling-stake-indias-286342. EXHIBIT 2: INDIAN FILM INDUSTRY FINANCIAL ANALYSIS REPORT Movie Name Total Net Gross (INR) Budget (INR) Screens Star Dhoom 3 2,612,100,000 1,500,000,000 3,800 1 Ra. One 1,139,475,000 1,300,000,000 2,900 1 Chennai Express 2,076,900,000 1,100,000,000 3,600 1 Once Upon a Time in Mumbai Dobaara! 584,900,000 1,000,000,000 2,300 1 Aatma 83,450,000 110,000,000 1,300 0 Mickey Virus 78,050,000 110,000,000 1,000 0 Rabba Main Kya Karoon 10,575,000 110,000,000 400 0 London Paris New York 63,800,000 105,000,000 550 0 EXHIBIT 3: LOG REGRESSION OUTPUTS Log Regression 1: Predicting the mean log(Box Office) using Star, log(Budget) and log(Screens) Loge (Box office) = Intercept + (Coefficient1) (Star) + (Coefficent2) (Loge Budget) + (Coefficient3) (Loge Screens) Coefficients Estimate Standard Error t value Pr(>|t|) (Intercept) 5.7687 1.9197 3.005 0.002885 ** Star 0.3943 0.1115 3.537 0.000471 *** Budget 0.1482 0.1215 1.220 0.223479 Screens 1.4706 0.1026 14.334 < 2e-16 *** Significance codes: 0 *** 0.001 ** 0.01 * 0.05 . 0.1 1 Residual standard error: 0.7682 on 293 degrees of freedom Multiple R-squared: 0.6959, Adjusted R-squared: 0.6928 F-statistic: 223.5 on 3 and 293 DF, p-value: < 2.2e-16 Log Regression 2: Predicting the mean log(Box Office) using Star and log(Screens) Loge (Box office) = Intercept + (Coefficient1) (Star) + (Coefficient2) (Loge Screens) Coefficients Estimate Standard Error t value Pr(>|t|) (Intercept) 8.03146 0.49504 16.224 < 2e-16 *** Star 0.44233 0.10439 4.237 3.03e-05 *** Screens 1.55758 0.07384 21.095 < 2e-16 *** Significance codes: 0 *** 0.001 ** 0.01 * 0.05 . 0.1 1 Residual standard error: 0.7688 on 294 degrees of freedom Multiple R-squared: 0.6944, Adjusted R-squared: 0.6923 F-statistic: 334 on 2 and 294 DF, p-value: < 2.2e-16 XHIBIT 4: QUANTILE REGRESSION OUTPUTS Quantile Regression 1: Predicting the 10th percentile of log(Box Office) using Star, log(Budget), and log(Screens) Coefficients Value Standard Error t value Pr(>|t|) (Intercept) 1.11150 2.93688 0.37846 0.70536 Star 0.60504 0.14961 4.04415 0.00007 Budget 0.50483 0.19516 2.58669 0.01017 Screens 1.32462 0.16840 7.86597 0.00000 Quantile Regression 2: Predicting the 25th percentile of log(Box Office) using Star, log(Budget), and log(Screens) Coefficients Value Standard Error t value Pr(>|t|) (Intercept) 1.77625 2.09388 0.84831 0.39696 Star 0.52088 0.12253 4.25117 0.00003 Budget 0.30617 0.13925 2.19864 0.02869 Screens 1.52156 0.13155 11.5622 0.00000 Quantile Regression 3: Predicting the 50th percentile of log(Box Office) using Star, log(Budget), and log(Screens) Coefficients Value Standard Error t value Pr(>|t|) (Intercept) 7.13596 2.53504 2.81493 0.00521 Star 0.37228 0.14405 2.58433 0.01024 Budget 0.02935 0.15518 0.18911 0.85014 Screens 1.60742 0.11144 14.42373 0.00000 Quantile Regression 4: Predicting the 50th percentile of log(Box Office) using Star and log(Screens) Coefficients Value Standard Error t value Pr(>|t|) (Intercept) 7.67060 0.67662 11.3361 0.00000 Star 0.41186 0.12539 3.28468 0.00114 Screens 1.60951 0.10029 16.04832 0.00000 Quantile Regression 5: Predicting the 75th percentile of log(Box Office) using Star, log(Budget), and log(Screens) Coefficients Value Standard Error t value Pr(>|t|) (Intercept) 8.25137 0.70655 11.67844 0.00000 Star 0.25278 0.09772 2.58690 0.01017 Budget 0.04560 0.05858 0.77844 0.43694 Screens 1.48199 0.13629 10.87396 0.00000 EXHIBIT 4 (CONTINUED) Quantile Regression 6: Predicting the 75th percentile of log(Box Office) using Star and log(Screens) Coefficients Value Standard Error t value Pr(>|t|) (Intercept) 8.78914 0.71381 12.31308 0.00000 Star 0.24419 0.11584 2.10804 0.03587 Screens 1.53469 0.10157 15.11001 0.00000 Quantile Regression 7: Predicting the 90th percentile of log(Box Office) using Star, log(Budget), and log(Screens) Coefficients Value Standard Error t value Pr(>|t|) (Intercept) 8.68576 1.10943 7.82901 0.00000 Star 0.14172 0.07807 1.81527 0.07050 Budget 0.14964 0.08446 1.77180 0.07747 Screens 1.18726 0.14779 8.03332 0.00000 Quantile Regression 8: Predicting the 90th percentile of log(Box Office) using Star and log(Screens) Coefficients Value Standard Error t value Pr(>|t|) (Intercept) 10.96996 0.73463 14.93263 0.00000 Star 0.18845 0.13059 1.44299 0.15009 Screens 1.27688 0.10444 12.22618 0.00000 Quantile Regression 9: Predicting the 90th percentile of log(Box Office) using log(Budget) and log(Screens) Coefficients Value Standard Error t value Pr(>|t|) (Intercept) 7.43025 1.32027 5.62784 0.00000 Budget 0.19819 0.10532 1.88187 0.06084 Screens 1.23842 0.15100 8.20143 0.00000 Quantile Regression 10: Predicting the 90th percentile of log(Box Office) using Star and log(Screens) Value Standard Error t value Pr(>|t|) (Intercept) 10.96996 0.73463 14.93263 0.00000 Star 0.18845 0.13059 1.44299 0.15009 Screens 1.27688 0.10444 12.22618 0.00000 Quantile Regression 11: Predicting the 90th percentile of log(Box Office) using log(Screens) Coefficients Value Standard Error t value Pr(>|t|) (Intercept) 9.66798 0.85738 11.27622 0.00000 Screens 1.47248 0.11578 12.71833 0.00000 Quantile Regression 12: Predicting the 90th percentile of log(Box Office) using log(Budget) Coefficients Value Standard Error t value Pr(>|t|) (Intercept) -1.15978 1.59321 0.72795 0.46722 Budget 1.09204 0.07921 13.78673 0.00000 Quantile Regression 13: Predicting the 90th percentile of log(Box Office) using Star Value Standard Error t value Pr(>t) (Intercept) 19.95097 0.09972 200.06023 0.00000 Star 0.96062 0.17897 5.36738 0.00000 Note: All quantile regressions were done using the statistical software and programming language R. Source: Created by authors. 1. Why was Irving not satisfied with Smiths initial analysis using log linear regression? How did Smith address the weakness in her initial analysis? (5 Marks) 2. What insights would you provide to Irving, based on smiths revised analysis using quantiles regression? (5 Marks) 3. What investment strategy would you recommend to Irving? (5 Marks)
RYLEE SMITH
Investment manager Rylee Smith was thinking about the meeting she had just held with her team at Glitz Investments, an investment firm based in the United States that focused on the entertainment industry. Specifically, Smith was pondering the comments that her fellow investment manager had made:
Were forgoing a lucrative opportunity by not investing in the east. Some international movie industries are seeing high growth rates. It will be a mistake to not shift our investment focus to that region. Specifically, Ive gathered some information on Bollywoodthe Indian movie industry and Rylee, I think you should look over it. Weve got some potential movies lined up, we just have to choose one if we decide to give this project a go.
Smith knew that investing in the Indian film industry for the first time would be a risk for Glitz Investments. However, such a move, if executed properly, could become a critical turning point for the company. Another colleague who had attended the meeting agreed. This could be a great opportunity for us, stated the colleague. Capitalizing on these trends could provide us with access to an untapped market.
From the research that was given to her, Smith understood that if Glitz Investments wanted to expand its investment focus geographically, Bollywood would be the perfect place to begin. The main challenge was choosing the right movies as investment vehicles. Although it was an attractive industry for investment, many movie projects across the world resulted in failure. Smith decided that she had to gain a better understanding of the unique features of the Indian movie industry.
Smith studied the report that the analysts at the firm had compiled for her (see Exhibits 1 and 2). She noticed that the team had collected data only for movies with a budget of 100 million1 or higher, which was consistent with the firms policy to invest in movies with a minimum level of production quality. She wondered if she could determine a quantitative relationship between box office performance and the factors that the analysts had identified. Could Glitz Investments selectively choose movies based on Smiths analysis? Half an hour later, she had created a couple of log linear regression models (see Exhibits 3 and 4). It was time to share some conclusions with her boss, Carl Irving. Smith reached for her phone and called Irving.
CARL IRVING
On the other end of the phone line, Smiths boss listened carefully to her conclusions before replying, This is good Rylee, but I want something more. It shows how we can improve our average return but I want an analysis that guarantees or at least improves the odds that we strike a jackpot Bollywood movie.
Smith shook her head to herself and spoke into her phone: If there was such a thing, Carl, then everyone would be using that magic formula to guarantee sky-high ROIs [returns on investment].
Irving replied with his own assessment of the situation:
Of course, thats a given. But come on, in a world where analytics is providing so many different ways to look at data, surely something as straightforward as log linear regression cannot be the only analytical technique we can use. I want more refined analysis and I want it by the end of this week. I mean, look at the trends of the Bollywood market. The 1B Club of box office earnings is going to be superseded by a new 2 billion benchmark. Thats what I want to identifya 2 billion movie that sets the precedent for forthcoming blockbusters. Nothing else.
And without warning, Smith heard the phone click on the other end. What was she going to do? And how was she going to further dissect the analysis she had conducted?
Smith felt frustrated, but as she logged into Facebook and started to mindlessly go through her newsfeed, something caught her eye. A former classmate from her master of business administration (MBA) studies had posted a new status. More important than the Facebook status was the name of the classmate that caught her attention. Ian Thomas had been widely known as the analytics wizard of the MBA program. If anyone could expertly interpret the Bollywood data, it was him. Without hesitating, Smith picked up her phone and called Thomas.
IAN THOMAS
Thomas agreed to meet with Smith two days later at a nearby coffee shop. After exchanging pleasantries, the two former classmates got down to business.
Okay so show me what youve got, stated Thomas.
Smith reached for her notes and started explaining the situation:
Definitely. Heres what Ive done already. I performed a log linear regression between the net gross of Bollywood movies over the past five years and the following factors: budget, number of screens, and star factor. While I found significant relationships between these factors and the net gross, this information is not enough. My boss wants more insight on this information. The thing is that he wants to invest in a movie that is not only going to be a success but a game changer for the industryone that has the potential to break all the Indian box office records. I just dont know what else to do with this information to answer his question. Frankly, Im not sure if it can be answered at all.
Hmmm. Lets see. Do you remember the concept we learned at schoolquantile regression? asked Thomas.
Oh yeah. I do remember that concept. Quantile regression helps us model the relationship between an independent variable X and the specific percentiles or quantiles of the response variable Y. I also recall that quantile regression is especially useful to identify relationships in markets where outcomes are highly uncertain, as is the case with many cultural industries. My word, it will be perfect for our Bollywood data.
Exactly. Averages dont mean much in the film industry because producers and investors care only about blockbusters. No wonder your boss is so antsy about hitting the jackpot.
Well hes antsy in general, so dont make excuses for him. Do you want to apply quantile regression to the data? asked Smith.
Yeah, definitely, confirmed Thomas.
Smith and Thomas got to work. A few hours later, they had the output from their analysis in front of them (see Exhibit 4). Okay, Thomas started, now that we have performed further analysis using quantile regression, its time to understand what all these numbers mean. Hopefully, by the end of today, youll be able to give your boss some insights.
EXHIBIT 1: INDIAN FILM INDUSTRY ANALYSIS REPORT
The Bollywood Report
Based in Mumbai, the Indian movie industry, known widely as Bollywood, is one of the fastest growing movie industries in the world. In 2013, the value of the entire industry was estimated at 125 billion. Each year, box office milestones are created by Bollywood moviesnot only in India, but across the world. The domestic film industry market was expected to grow at an annual compound annual growth rate (CAGR) of 11.4 per cent until 2018, while the overseas market was projected to grow at a CAGR of 8.9 per cent. Two other significant revenue streams for Bollywood movie production companies are cable and satellite television rights and ancillary revenue streams. Cable and satellite television rights were expected to grow at an annual rate of
10.7 per cent, whereas ancillary revenue streams were expected to grow at a rate of 24.7 per cent.
The 1B Club
In 2008, the Indian movie industry released a blockbuster movie that earned a staggering 1.14 billion in domestic revenues. This marked the creation of the coveted 1B Club, which consisted of movies that earned over 1 billion domestically. Year over year, an increasing number of movies recorded earnings above that benchmark. Currently, 25 movies hold a place in the 1B Club. Analysts predict that the 1B Club will eventually be replaced by a 2B Club, as more movies break box office records set by predecessors.
Hollywoods Interest in Bollywood
Foreign investment in the Indian movie industry is not new. Many Hollywood production companies have already entered Bollywood, contributing to this lucrative industry. For example, 20th Century Fox Film Corporation entered the industry as a joint venture and later acquired the leading Indian media and entertainment company Star India Private Limited to distribute and produce over 25 Indian movies. Similarly, Viacom started a joint venture with the India-based media company Network 18. Disney has a majority stake in UTV Software Communications, one of the top Indian movie production companies.
Source: KPMG, The Stage Is Set: FICCI-KPMG Indian Media and Entertainment Industry Report, 2014, accessed May 31, 2019, https://assets.kpmg/content/dam/kpmg/pdf/2014/03/FICCI-Frames-2014-The-stage-is-set-Report-2014.pdf; Nyay Bhushan, Disney Acquires Controlling Stake in Indias UTV, The Hollywood Reporter, February 1, 2012, accessed May 31, 2019, www.hollywoodreporter.com/news/disney-acquires-controlling-stake-indias-286342.
EXHIBIT 2: INDIAN FILM INDUSTRY FINANCIAL ANALYSIS REPORT
Movie Name Total Net Gross (INR)
Budget (INR)
Screens
Star
Dhoom 3 2,612,100,000 1,500,000,000 3,800 1
Ra. One 1,139,475,000 1,300,000,000 2,900 1
Chennai Express 2,076,900,000 1,100,000,000 3,600 1
Once Upon a Time in Mumbai Dobaara! 584,900,000 1,000,000,000 2,300 1
Aatma 83,450,000 110,000,000 1,300 0
Mickey Virus 78,050,000 110,000,000 1,000 0
Rabba Main Kya Karoon 10,575,000 110,000,000 400 0
London Paris New York 63,800,000 105,000,000 550 0
EXHIBIT 3: LOG REGRESSION OUTPUTS
Log Regression 1: Predicting the mean log(Box Office) using Star, log(Budget) and log(Screens) Loge (Box office) = Intercept + (Coefficient1) (Star) + (Coefficent2) (Loge Budget)
+ (Coefficient3) (Loge Screens)
Coefficients
Estimate Standard Error t value Pr(>|t|)
(Intercept) 5.7687 1.9197 3.005 0.002885
**
Star 0.3943 0.1115 3.537 0.000471
***
Budget 0.1482 0.1215 1.220 0.223479
Screens 1.4706 0.1026 14.334 < 2e-16 ***
Significance codes: 0 *** 0.001 ** 0.01 * 0.05 . 0.1 1 Residual standard error: 0.7682 on 293 degrees of freedom
Multiple R-squared: 0.6959, Adjusted R-squared: 0.6928
F-statistic: 223.5 on 3 and 293 DF, p-value: < 2.2e-16
Log Regression 2: Predicting the mean log(Box Office) using Star and log(Screens) Loge (Box office) = Intercept + (Coefficient1) (Star) + (Coefficient2) (Loge Screens)
Coefficients
Estimate Standard Error t value Pr(>|t|)
(Intercept) 8.03146 0.49504 16.224 < 2e-16 ***
Star 0.44233 0.10439 4.237 3.03e-05
***
Screens 1.55758 0.07384 21.095 < 2e-16 ***
Significance codes: 0 *** 0.001 ** 0.01 * 0.05 . 0.1 1 Residual standard error: 0.7688 on 294 degrees of freedom
Multiple R-squared: 0.6944, Adjusted R-squared: 0.6923
F-statistic: 334 on 2 and 294 DF, p-value: < 2.2e-16
XHIBIT 4: QUANTILE REGRESSION OUTPUTS
Quantile Regression 1: Predicting the 10th percentile of log(Box Office) using Star, log(Budget), and log(Screens) Coefficients
Value Standard Error t value Pr(>|t|)
(Intercept) 1.11150 2.93688 0.37846 0.70536
Star 0.60504 0.14961 4.04415 0.00007
Budget 0.50483 0.19516 2.58669 0.01017
Screens 1.32462 0.16840 7.86597 0.00000
Quantile Regression 2: Predicting the 25th percentile of log(Box Office) using Star, log(Budget), and log(Screens) Coefficients
Value Standard Error t value Pr(>|t|)
(Intercept) 1.77625 2.09388 0.84831 0.39696
Star 0.52088 0.12253 4.25117 0.00003
Budget 0.30617 0.13925 2.19864 0.02869
Screens 1.52156 0.13155 11.5622 0.00000
Quantile Regression 3: Predicting the 50th percentile of log(Box Office) using Star, log(Budget), and log(Screens) Coefficients
Value Standard Error t value Pr(>|t|)
(Intercept) 7.13596 2.53504 2.81493 0.00521
Star 0.37228 0.14405 2.58433 0.01024
Budget 0.02935 0.15518 0.18911 0.85014
Screens 1.60742 0.11144 14.42373 0.00000
Quantile Regression 4: Predicting the 50th percentile of log(Box Office) using Star and log(Screens) Coefficients
Value Standard Error t value Pr(>|t|)
(Intercept) 7.67060 0.67662 11.3361 0.00000
Star 0.41186 0.12539 3.28468 0.00114
Screens 1.60951 0.10029 16.04832 0.00000
Quantile Regression 5: Predicting the 75th percentile of log(Box Office) using Star, log(Budget), and log(Screens) Coefficients
Value Standard Error t value Pr(>|t|)
(Intercept) 8.25137 0.70655 11.67844 0.00000
Star 0.25278 0.09772 2.58690 0.01017
Budget 0.04560 0.05858 0.77844 0.43694
Screens 1.48199 0.13629 10.87396 0.00000
EXHIBIT 4 (CONTINUED)
Quantile Regression 6: Predicting the 75th percentile of log(Box Office) using Star and log(Screens) Coefficients
Value Standard Error t value Pr(>|t|)
(Intercept) 8.78914 0.71381 12.31308 0.00000
Star 0.24419 0.11584 2.10804 0.03587
Screens 1.53469 0.10157 15.11001 0.00000
Quantile Regression 7: Predicting the 90th percentile of log(Box Office) using Star, log(Budget), and log(Screens) Coefficients
Value Standard Error t value Pr(>|t|)
(Intercept) 8.68576 1.10943 7.82901 0.00000
Star 0.14172 0.07807 1.81527 0.07050
Budget 0.14964 0.08446 1.77180 0.07747
Screens 1.18726 0.14779 8.03332 0.00000
Quantile Regression 8: Predicting the 90th percentile of log(Box Office) using Star and log(Screens) Coefficients
Value Standard Error t value Pr(>|t|)
(Intercept) 10.96996 0.73463 14.93263 0.00000
Star 0.18845 0.13059 1.44299 0.15009
Screens 1.27688 0.10444 12.22618 0.00000
Quantile Regression 9: Predicting the 90th percentile of log(Box Office) using log(Budget) and log(Screens)
Coefficients
Value Standard Error t value Pr(>|t|)
(Intercept) 7.43025 1.32027 5.62784 0.00000
Budget 0.19819 0.10532 1.88187 0.06084
Screens 1.23842 0.15100 8.20143 0.00000
Quantile Regression 10: Predicting the 90th percentile of log(Box Office) using Star and log(Screens)
Value Standard Error t value Pr(>|t|)
(Intercept) 10.96996 0.73463 14.93263 0.00000
Star 0.18845 0.13059 1.44299 0.15009
Screens 1.27688 0.10444 12.22618 0.00000
Quantile Regression 11: Predicting the 90th percentile of log(Box Office) using log(Screens) Coefficients
Value Standard Error t value Pr(>|t|)
(Intercept) 9.66798 0.85738 11.27622 0.00000
Screens 1.47248 0.11578 12.71833 0.00000
Quantile Regression 12: Predicting the 90th percentile of log(Box Office) using log(Budget) Coefficients
Value Standard Error t value Pr(>|t|)
(Intercept) -1.15978 1.59321 0.72795 0.46722
Budget 1.09204 0.07921 13.78673 0.00000
Quantile Regression 13: Predicting the 90th percentile of log(Box Office) using Star
Value Standard Error t value Pr(>t)
(Intercept) 19.95097 0.09972 200.06023 0.00000
Star 0.96062 0.17897 5.36738 0.00000
Note: All quantile regressions were done using the statistical software and programming language R. Source: Created by authors.
1. Why was Irving not satisfied with Smiths initial analysis using log linear regression? How did Smith
address the weakness in her initial analysis? (5 Marks)
2. What insights would you provide to Irving, based on smiths revised analysis using quantiles
regression? (5 Marks)
3. What investment strategy would you recommend to Irving? (5 Marks)
Step by Step Solution
There are 3 Steps involved in it
Step: 1
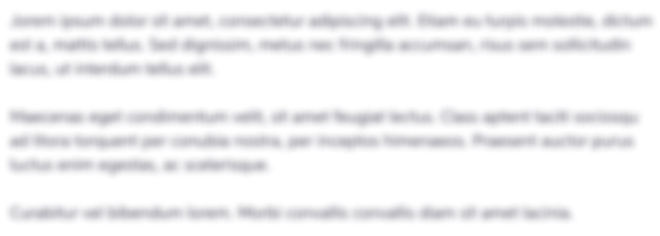
Get Instant Access with AI-Powered Solutions
See step-by-step solutions with expert insights and AI powered tools for academic success
Step: 2

Step: 3

Ace Your Homework with AI
Get the answers you need in no time with our AI-driven, step-by-step assistance
Get Started