Question
se the k-means algorithm and Euclidean distance to cluster the following 8 examples into 3 clusters: A1=(4,10), A2=(5,8), A3=(1,7), A4=(4,7), A5=(6,5), A6=(6,10), A7=(8,5), A8=(4,9). Suppose
se the k-means algorithm and Euclidean distance to cluster the following 8 examples into 3 clusters: A1=(4,10), A2=(5,8), A3=(1,7), A4=(4,7), A5=(6,5), A6=(6,10), A7=(8,5), A8=(4,9). Suppose that the initial seeds (centres of each cluster) are A3, A5 and A7. Run the k-means algorithm for 1 epoch only. In particular: 1. Fill the distance matrix based on the Euclidean distance of the points given above: A1 A2 A3 A4 A5 A6 A7 A8 A1 0 A2 0 A3 0 A4 0 A5 0 A6 0 A7 0 A8 0 2. Calculate the cluster assignment at the end of the first epoch: a. The new cluster assignment (i.e. contents of each cluster) b. The centroids of the new clusters 3. How many more iterations are needed to converge? Show cluster assignments and updated centroids for each of the remaining epochs.
Step by Step Solution
There are 3 Steps involved in it
Step: 1
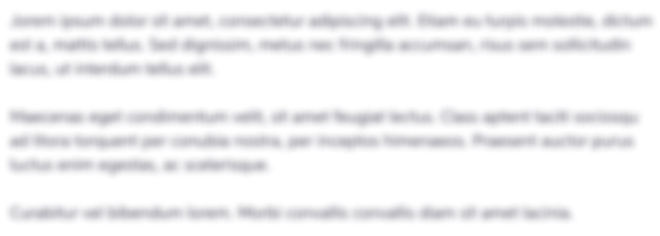
Get Instant Access to Expert-Tailored Solutions
See step-by-step solutions with expert insights and AI powered tools for academic success
Step: 2

Step: 3

Ace Your Homework with AI
Get the answers you need in no time with our AI-driven, step-by-step assistance
Get Started