Question
Some designers of products think they have a firm grasp on the requirements and preferences of their target market from the very beginning. It goes
Some designers of products think they have a firm grasp on the requirements and preferences of their target market from the very beginning.
It goes without saying that this kind of thinking frequently results in poor design choices.
An example of a cognitive bias that might significantly harm the outcome is the false-consensus effect.
One of usability's most painful lessons is that "you are not the user," according to Jakob Nielsen, who summed up the danger of this prejudice.
Most of the time, designers are not their intended audience.
Designers frequently develop things based on their own beliefs when there is no data to back up those assumptions regarding specific situations.
Data provides designers with insight, and that insight can assist in guiding product design in the proper direction.
Different ways of using data are represented by data-driven and data-informed design.
Data is essential for data-driven design since the team centers it on all of its design choices and uses it as a main input.
Every possible solution to an issue is weighed against the team's data while debating a particular design choice.
By using data as a reference, the product team is implying that they use data to guide their decisions.
In other words, the product team incorporates qualitative feedback and other inputs, such as design intuition, with statistics as a source of information.
When product teams desire to find answers to the "What" and "How many" queries (i.e., "What's happening?" and "How many users [do something]? "), the data-driven method works effectively.
When a team wishes to optimize a particular component of the product, this strategy is helpful.
For instance, if a product team wants to lower the bounce rate on a landing page, numerical indicators like average time on page or time-to-load might assist them in identifying the challenges that customers are encountering.
More data collection isn't the only goal of data-driven design.
In order to example a better product, data-driven design entails gathering information that can reveal insights into user behavior.
Therefore, it's crucial to first comprehend business objectives as well as consumer wants.
Spend time and energy on user research, then choose a set of KPIs that align with your corporate objectives.
A large amount of data must normally be collected for a data-driven method to provide a statistically significant outcome.
Because they won't have adequate data, small enterprises and startups might not be a good fit for this method.
It's crucial to use data-driven strategies sparingly.
Some businesses try to over-optimize straightforward elements of their products, which frequently results in frustrating design rather than a positive user experience.
Google's experimentation with 41 colors of blue is a particularly remarkable example.
Always keep in mind the objectives of your tests and your motivations.
A/B testing is the process of comparing the performance of two versions of a product after being presented to various users.
A/B testing necessitates altering one particular design element, usually a minor adjustment like the color of a call-to-action button.
Teams who conduct A/B testing often gauge conversion rates when it comes to measuring performance (e.g., for a landing page, this might be the number of new sign-ups).
I strongly suggest reading 37Signals' A/B testing case study on homepage design if you'd like to see some examples of how A/B testing operates for real products.
Step by Step Solution
There are 3 Steps involved in it
Step: 1
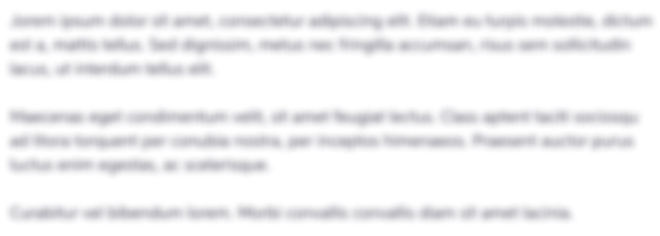
Get Instant Access to Expert-Tailored Solutions
See step-by-step solutions with expert insights and AI powered tools for academic success
Step: 2

Step: 3

Ace Your Homework with AI
Get the answers you need in no time with our AI-driven, step-by-step assistance
Get Started