Question
Summary. In new research, the authors study the use of dynamic pricing and targeted discounts, in which they asked if (and how) biases might arise
Summary.In new research, the authors study the use of dynamic pricing and targeted discounts, in which they asked if (and how) biases might arise if the prices consumers pay are decided by an algorithm.Suppose your company wants to use historical data to train an algorithm to identify customers who are most...more
Tweet
Post
Share
Save
Buy Copies
In theory, marketing personalization should be a win-win proposition for both companies and customers. By delivering just the right mix of communications, recommendations, and promotions all tailored to each individual's particular tastes marketing technologies can result in uniquely satisfying consumer experiences.
While ham-handed attempts at personalization can give the practicea bad rap, targeting technologies are becoming more sophisticated every day. New advancements in machine learning and big data are making personalization more relevant, less intrusive, and less annoying to consumers. However, along with these developments come a hidden risk: the ability of automated systems to perpetuate harmful biases.
In new research, we studied the use of dynamic pricing and targeted discounts, in which we asked if (and how) biases might arise if the prices consumers pay are decided by an algorithm. A cautionary tale of this type of personalized marketing practice is that of the Princeton Review. In 2015, it was revealed that the test-prep company wascharging customers in different ZIP codes different prices, with discrepancies between some areas reaching hundreds of dollars, despite the fact that all of its tutoring sessions took place via teleconference. In the short term, this type of dynamic pricing may have seemed like an easy win for boosting revenues. Butresearch has consistently shownthat consumers view it as inherently unfair,leading to lower trust and repurchasing intentions. What's more, Princeton Review's bias had a racial element:a highly publicized follow-up investigationby journalists at ProPublica demonstrated how the company's system was, on average, systematically charging Asian families higher prices than non-Asians.
INSIGHT CENTER
AI and Bias
Building fair and equitable machine learning systems.
Even the largest of tech companies and algorithmic experts have found it challenging to deliver highly personalized services while avoiding discrimination. Severalstudieshave shown that ads for high-paying job opportunities on platforms such as Facebook and Google are served disproportionately to men. And, just this year,Facebook was suedand found to be in violation of the Fair Housing Act for allowing real estate advertisers to target users by protected classes, including race, gender, age, and more.
What's going on with personalization algorithms and why are they so difficult to wrangle? In today's environment with marketing automation software and automatic retargeting, A/B testing platforms that dynamically optimize user experiences over time, and ad platforms that automatically select audience segments more important business decisions are being made automatically without human oversight. And while the data that marketers use to segment their customers are not inherently demographic, these variables are often correlated with social characteristics.
To understand how this works, suppose your company wants to use historical data to train an algorithm to identify customers who are most receptive to price discounts. If the customer profiles you feed into the algorithm contain attributes that correlate with demographic characteristics, the algorithm is highly likely to end up making different recommendations for different groups. Consider, for example, how often cities and neighborhoods are divided by ethnic and social classes and how often a user's browsing data may be correlated with their geographic location (e.g., through their IP address or search history). What if users in white neighborhoods responded strongest to your marketing efforts in the last quarter? Or perhaps users in high-income areas were most sensitive to price discounts. (This is known to happen in some circumstances not because high-income customers can't afford full prices but because they shop more frequently online andknow to wait for price drops.) An algorithm trained on such historical data would even without knowing the race or income of customers learn to offer more discounts to the white, affluent ones.
To investigate this phenomenon, we looked at dozens of large-scale e-commerce pricing experiments to analyze how people around the United States responded to different price promotions. By using a customer's IP address as an approximation of their location, we were able to match each user to a US Census tract and use public data to get an idea of the average income in their area. Analyzing the results of millions of website visits, we confirmed that, as in the hypothetical example above, people in wealthy areas responded more strongly to e-commerce discounts than those in poorer ones and, since dynamic pricing algorithms are designed to offer deals to users most likely to respond them, marketing campaigns would probably systematically offerlowerprices tohigherincome individuals going forward.
What can your company can do to minimize these socially undesirable outcomes?One possibility for algorithmic risk-mitigationis formal oversight for your company's internal systems. Such "AI audits" are likely to be complicated processes, involving assessments of accuracy, fairness, interpretability, and robustness of all consequential algorithmic decisions at your organization.
While this sounds costly in the short term, it may turn out to be beneficial for many companies in the long term.Because "fairness" and "bias" are difficult to universally define, getting into the habit of having more than one set of eyes looking for algorithmic inequities in your systems increases the chances you catch rogue code before it ships. Given the social, technical, and legal complexities associated with algorithmic fairness, it will likely become routine to have a team of trained internal or outside experts try to find blind spots and vulnerabilities in any business processes that rely on automated decision making.
As advancements in machine learning continue to shape our economy and concerns about wealth inequality and social justice increase, corporate leaders must be aware of the ways in which automated decisions can cause harm to both their customers and their organizations. It is more important than ever to consider how your automated marketing campaigns might discriminate against social and ethnic groups. Managers who anticipate these risks and act accordingly will be those who set their companies up for long-term success.
Read more onMarketingor related topicsPricingandTechnology
Step by Step Solution
There are 3 Steps involved in it
Step: 1
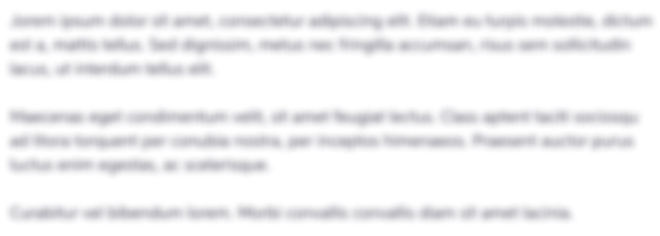
Get Instant Access to Expert-Tailored Solutions
See step-by-step solutions with expert insights and AI powered tools for academic success
Step: 2

Step: 3

Ace Your Homework with AI
Get the answers you need in no time with our AI-driven, step-by-step assistance
Get Started