Answered step by step
Verified Expert Solution
Question
1 Approved Answer
The data files are at the following links, since I'm unable to upload the file types here. ARFF - https://www.dropbox.com/s/zzrrynsizt07ffc/deception_data_converted_arff.arff?dl=0 CSV- https://www.dropbox.com/s/0wment04x9xwu8l/deception_data_converted_final.csv?dl=0 Scenario: Some people
The data files are at the following links, since I'm unable to upload the file types here.
ARFF - https://www.dropbox.com/s/zzrrynsizt07ffc/deception_data_converted_arff.arff?dl=0
CSV- https://www.dropbox.com/s/0wment04x9xwu8l/deception_data_converted_final.csv?dl=0
Scenario: Some people claimed that machine learning algorithms can figure out whether a person is lying or not. Do you believe that? To test this claim, we have collected a collection of customer reviews, some are true some are fake, and you are going to test how good multinomia SVMs can be for fake review detection. l NB and This data set also has sentiment label for each review. You will also MNB and SVMs performance in sentiment classification. For both tasks, try tune parameters and report results in a table like Parameter Precision in Recall in category category category II category II Overall Precision in Recall in Explain your initial parameter tuning strategy (which parameter to tune, to what option, and theoretical foundation for your choice). Does your strategy help you get better results? Compare performance difference in sentiment classification and lie detection, and tell us which task is harder, and try to explain why. For each task, use GainRatio and Chi2 to rank the features and list top20 features from each method. Based on these top features, can you understand what patterns the classifiers have learned from the data? Scenario: Some people claimed that machine learning algorithms can figure out whether a person is lying or not. Do you believe that? To test this claim, we have collected a collection of customer reviews, some are true some are fake, and you are going to test how good multinomia SVMs can be for fake review detection. l NB and This data set also has sentiment label for each review. You will also MNB and SVMs performance in sentiment classification. For both tasks, try tune parameters and report results in a table like Parameter Precision in Recall in category category category II category II Overall Precision in Recall in Explain your initial parameter tuning strategy (which parameter to tune, to what option, and theoretical foundation for your choice). Does your strategy help you get better results? Compare performance difference in sentiment classification and lie detection, and tell us which task is harder, and try to explain why. For each task, use GainRatio and Chi2 to rank the features and list top20 features from each method. Based on these top features, can you understand what patterns the classifiers have learned from the dataStep by Step Solution
There are 3 Steps involved in it
Step: 1
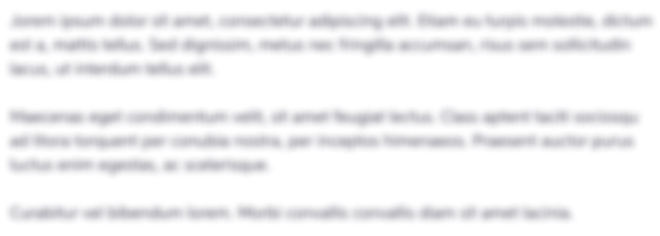
Get Instant Access to Expert-Tailored Solutions
See step-by-step solutions with expert insights and AI powered tools for academic success
Step: 2

Step: 3

Ace Your Homework with AI
Get the answers you need in no time with our AI-driven, step-by-step assistance
Get Started