Question
The python code below implements an autoencoder. Please modify the code to experiement with sampling and clamping with the MNIST data and denoising autoencoder. Some
The python code below implements an autoencoder. Please modify the code to experiement with sampling and clamping with the MNIST data and denoising autoencoder. Some instructions are also pasted below to help get you started:
Instructions:
Code:
# denoising autoencoder of Geron import tensorflow as tf
n_inputs = 28 * 28 n_hidden1 = 300 n_hidden2 = 150 # codings n_hidden3 = n_hidden1 n_outputs = n_inputs
learning_rate = 0.01
noise_level = 1.0
X = tf.placeholder(tf.float32, shape=[None, n_inputs]) X_noisy = X + noise_level * tf.random_normal(tf.shape(X))
hidden1 = tf.layers.dense(X_noisy, n_hidden1, activation=tf.nn.relu, name="hidden1") hidden2 = tf.layers.dense(hidden1, n_hidden2, activation=tf.nn.relu, # not shown in the book name="hidden2") # not shown hidden3 = tf.layers.dense(hidden2, n_hidden3, activation=tf.nn.relu, # not shown name="hidden3") # not shown outputs = tf.layers.dense(hidden3, n_outputs, name="outputs") # not shown
reconstruction_loss = tf.reduce_mean(tf.square(outputs - X)) # MSE
optimizer = tf.train.AdamOptimizer(learning_rate) training_op = optimizer.minimize(reconstruction_loss) init = tf.global_variables_initializer()
from tensorflow.examples.tutorials.mnist import input_data mnist = input_data.read_data_sets("/tmp/data/")
n_epochs = 10 batch_size = 150
import sys
with tf.Session() as sess: init.run() for epoch in range(n_epochs): n_batches = mnist.train.num_examples // batch_size for iteration in range(n_batches): print(" {}%".format(100 * iteration // n_batches), end="") sys.stdout.flush() X_batch, y_batch = mnist.train.next_batch(batch_size) sess.run(training_op, feed_dict={X: X_batch}) loss_train = reconstruction_loss.eval(feed_dict={X: X_batch}) print(" {}".format(epoch), "Train MSE:", loss_train)
X_test - mnist.test.images[:n_test_digits] CO Markov chain: initialize state that is in the shape of X_test with random noise state outputs.eval(feed_dict-(X: state]) state state +noise level* tf.random_normal(tf.shape(X_test)) state = outputs.eval fee Clamping: need to assign the right half of X_test to state X_test - mnist.test.images[:n_test_digits] CO Markov chain: initialize state that is in the shape of X_test with random noise state outputs.eval(feed_dict-(X: state]) state state +noise level* tf.random_normal(tf.shape(X_test)) state = outputs.eval fee Clamping: need to assign the right half of X_test to stateStep by Step Solution
There are 3 Steps involved in it
Step: 1
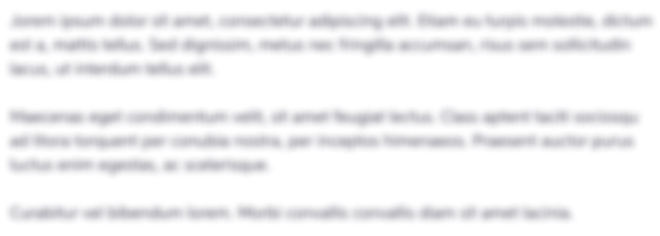
Get Instant Access to Expert-Tailored Solutions
See step-by-step solutions with expert insights and AI powered tools for academic success
Step: 2

Step: 3

Ace Your Homework with AI
Get the answers you need in no time with our AI-driven, step-by-step assistance
Get Started