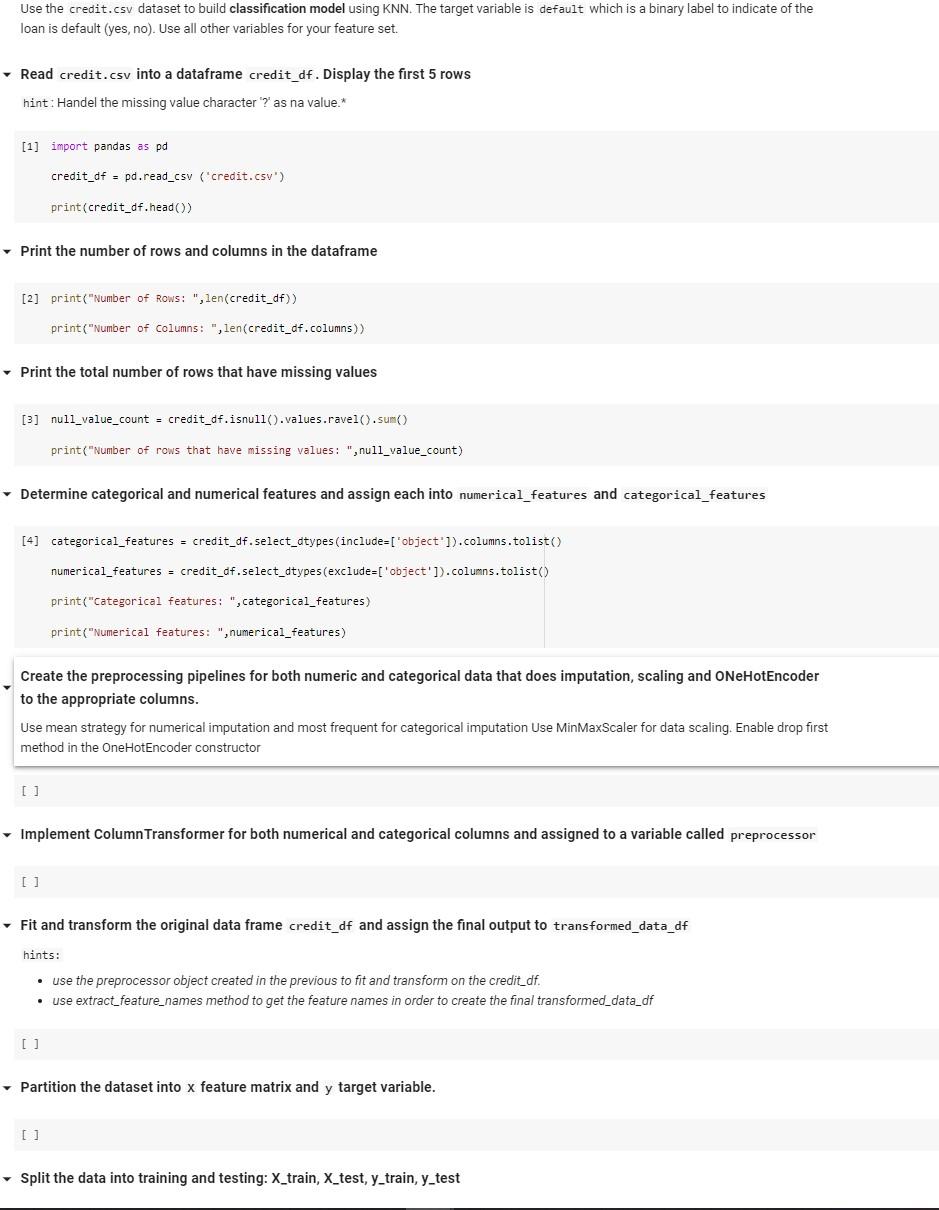
Use the credit.csv dataset to build classification model using KNN. The target variable is default which is a binary label to indicate of the loan is default (yes, no). Use all other variables for your feature set. Read credit.csv into a dataframe credit_df. Display the first 5 rows hint: Handel the missing value character'? as na value. * [1] import pandas as pd credit_df = pd.read_csv ('credit.csv") print(credit_df.head() Print the number of rows and columns in the dataframe [2] print("Number of Rows: ", len(credit_df)) print("Number of Columns: ", len(credit_df.columns)) Print the total number of rows that have missing values [3] null_value_count = credit_df.isnull().values.ravel().sum() print("Number of rows that have missing values: ", null_value_count) Determine categorical and numerical features and assign each into numerical_features and categorical_features [4] categorical_features = credit_df.select_dtypes (include= ['object']).columns.tolisto numerical_features = credit_of.select_dtypes (exclude=['object']).columns.tolist() print("categorical features: ", categorical_features) print("Numerical features: ", numerical_features) Create the preprocessing pipelines for both numeric and categorical data that does imputation, scaling and OneHotEncoder to the appropriate columns. Use mean strategy for numerical imputation and most frequent for categorical imputation Use MinMaxScaler for data scaling Enable drop first method in the OneHotEncoder constructor [] Implement Column Transformer for both numerical and categorical columns and assigned to a variable called preprocessor [] Fit and transform the original data frame credit_df and assign the final output to transformed_data_df hints: use the preprocessor object created in the previous to fit and transform on the credit_df. use extract_feature_names method to get the feature names in order to create the final transformed_data_df [] Partition the dataset into x feature matrix and y target variable. [] Split the data into training and testing: X_train, X_test, y_train, y_test