Question
Video and Image data With the ubiquity of video and image capture devices, visual data are more prevalent than ever. The use of these data
Video and Image data With the ubiquity of video and image capture devices, visual data are more prevalent than ever. The use of these data to augment accounting records is quickly becoming a reality as video and image storage, processing, and analytic techniques mature. Evolving approaches that derive objective information from video increase the feasibility of video-enhanced accounting records, particularly as they relate to internal controls. A recent Metaxas and Zhang (2013) meta-analysis describes algorithms that detect entry into restricted access areas, count objects, analyze scenes, monitor vehicles, and detect emotions. These methods can add important information to accounting records including (1) surveillance footage to reveal when and how many times a restricted-access area is entered, (2) workplace video to track worker productivity (e.g., who is spending too much time around the water cooler), (3) video of inventory to assess real-time quantity changes to measure throughput and identify bottlenecks, and (4) video of the condition of property, plant, and equipment as an additional component to address potential impairment issues. Interview video data could also be a source of accounting information. Analyzing periodic interviews of management and the board of directors to extract content, emotion, and deception would provide nonverbal information about business and audit risks. In fact, these nonverbal components of interviews can be more valuable and relevant than the verbal content. Interview video data, combined with vocalic and linguistic elements, creates a more complete picture of executive intent and potentially gives insights about a companys health. To collect interview data efficiently, the process could be automated. Increasingly sophisticated computer algorithms process and interpret static images. Accurate object detection and semantic segmentation of images help computers automatically categorize and interpret images. Algorithms for object and scene recognition, crowd-sourced training of object detectors, and emotion detection contribute to this process. Similar to video data, these methods could extract valuable business data from images including objects and scenes that appear in company-related images uploaded by customers, the demographic breakdown of product users featured in those images, and the condition and use of company products found in images. Image analyses could generate useful business insights, including but not limited to knowledge concerning product usage tendencies, and accurate assessments of corporate image. Indeed, business disclosures that incorporate supplemental nonfinancial information, including video and images, can even enhance the perception of investment quality. Audio data Audio data related to business activities could also enhance accounting records and the quality of financial information. Potentially important audio sources include quarterly conference calls, shareholder and board of directors meetings, customer calls, internal employee phone calls, microphones placed on company premises for surveillance, and audio from video sources mentioned in the previous section. Supporting the aforementioned assertions, Mayew and Venkatachalam analyzed audio from quarterly earnings calls to detect cognitive dissonance in the speech patterns of chief executive officers. Their research showed a positive relationship between vocal cognitive dissonance markers and the likelihood of restatements attributable to irregularities. In a related study, positive and negative affective states in earnings conference calls significantly correlated with stock returns. Recently, vocalic measures have been included as independent variables in assessing trust, as well as the interactional synchrony between deceptive interviewers and interviewees. Other applications of audio analysis include crime detection, event identification in audio streams, and mood detection. With these audio data streams, Big Data can provide additional evidence to support accounting records. For example, audio interviews with construction engineers during the construction phase of plant assets offer additional evidence of their value and estimated period of benefit. This would help future accountants understand the basis for valuing these long-term assets and assist them in addressing potential impairment issues. Also, enhanced analysis of audio data from customer phone calls could yield additional customer satisfaction and product quality information and ultimately improve estimates for warranty liability. Textual data Textual data include nonfinancial or soft documentation. Some significant repositories of text are SEC filings, emails, web pages (including corporate documents found on company websites), news media, and social media. Social media is one of the largest and fastest-growing collections of relevant textual data. For example, the number of Facebook participants, Twittere users, and public blogs as of 2013 was 700 million, 250 million, and 156 million, respectively. Data from these sources will be instrumental in assessing and improving business performance. Email data are already mined to mitigate occupational fraud losses in the United States. These losses reached an estimated $652 billion in 2009 alone, and dissatisfied employees were the primary contributors. U.S. fraud losses continue to climb, rising to an estimated $1 trillion in 2012. In seeking measures for reducing fraud attributable to employees, text mining of email messages identifies disgruntled workers and predicts and addresses organizational fraud risk. More importantly, this method unearths pertinent information not revealed in a traditional fraud audit. Fraud Triangle Analytics (a fraud discovery method) applies the principles of the fraud triangle in analyzing email messages to detect employees with the opportunity, motivation, and rationalization to commit fraud. As companies extract and integrate information from video, audio, and textual data with traditional financial data, they enhance their abilities to evaluate and predict business performance for managerial accounting purposes. For instance, customer satisfaction is an important element of product and company value, and advanced analytic techniques can measure satisfaction levels. Customer feedback on websites and social media (e.g., positive words and phrases), customer telephone complaints and inquiries (e.g., vocal stress and tension), and Internet video reviews posted by customers and bloggers (e.g., frowns and other negative gesturing) could add to a customer satisfaction analysis. Finally, employee satisfaction is measured using similar types of data. Managerial accounting refers to the use of information generated from accounting records to help managers carry out their duties. One significant task for management accountants is to create systems that align organizational goals with the behaviors of management and employees. These behavior-regulating devices are known as management control systems (MCSs). They are distinguished from information and decision support systems that help managers make decisions. Research shows that managers will change their actions to achieve incentives tied to behaviors. Thus, when creating measures and associated incentives, managers must consider whether the incentives are aligned with corporate goals. One MCS that aligns metrics with company goals is the Balanced Scorecard (BSC). This system identifies financial and nonfinancial measures for behaviors that best fit with business objectives. How statistics from organizational units compare with the identified measures and associated goals constitutes management performance. As a result, the BSC and other MCSs identify positive (negative) management behaviors and then reward management for doing (avoiding) them. Big Data can play a role in MCSs by discovering behaviors correlated with specific goal outcomes, which would prompt the creation of corresponding performance measures. For example, the BSC collects data in four areas: financial, customer, internal business process, and learning and growth. Within each area, Big Data can identify new behaviors that influence respective goal outcomes. For instance, web use while at work may be tied to learning and growth goals, internal emails may correlate with the effectiveness of internal business processes as well as customer service quality, and customer service quality may be related to vocalic cues mined from customer service calls. In general, Big Data analyses can facilitate the discovery of important measures to be incorporated in MCSs. Companies can use metadata, such as the amount of time spent on a telephone, to track productivity. In sales, greater phone use might indicate higher productivity, whereas the converse could be true in manufacturing. Employee computers can also be monitored to generate activity logs that contain data on web use, click streams, and time spent using productivity software such as MS Excelt. Companies can also monitor employee telephone calls, emails, and in-office behaviors. Furthermore, companies can track what employees do with company resources away from the office including vehicles, cell phones, and P-Cards. Big Data could convert MCSs into comprehensive monitoring and control systems (CMCSs). A possible drawback of CMCSs, or increased employee monitoring in general, involves backlash attributable to economic, legal, and ethical issues. Also, excessive tracking could suppress employee creativity and/or motivation. If employers constantly micromanage employees, then they may fear showing originality or initiative. Hopwood demonstrated that monitoring through performance evaluations could be detrimental to morale and productivity if the wrong constructs are measured. For example, in the minds of managers, profitbased measures were much more fair than budget-oriented indicators. Indeed unfair (as perceived by managers) performance measures can cause negative behaviors including management avoiding difficult problems, making decisions that inflate profits (or reduce costs) in the short term, and causing harm to the company in exchange for short-term gains. The goal of monitoring and performance evaluations should be to improve productivity. Consequently, academic research has focused on identifying measures that will properly motivate workers. Big Data may hold the key to discovering new motivational measures and identifying harmful ones. Association rule learning is a technique for discovering correlations in very large datasets, such as relationships between good management performance and variables previously not considered. For example, the tone of emails and phone conversations made on company equipment could be indicators of employee morale. The number of emails sent by managers could be a proxy for productivity, and video-captured body language of customers could be a proxy for customer satisfaction. The more data that is captured, the greater the opportunity to discover useful knowledge. Budgeting represents one area of management control that has branched out from traditional data sources and embraced new methods of control. Traditional budgeting activities have been criticized for being too inwardly focused, stifling creativity and hampering competitive edge in an information economy. Because of the problems linked with budgeting, many companies are doing away with traditional methods and implementing so-called beyond budgeting techniques. Beyond budgeting is the use of alternative sources of information for operational planning, performance evaluation, communication of goals, and strategy formation. It is enabled by new information streams, such as those available in enterprise resource planning (ERP) systems. Big Data, including additional streams of data outside ERP systems (e.g., climate, satellite, census, labor, and macroeconomic data) could be used to enhance beyond budgeting practices. Companies could also use internally generated data, including the nontraditional accounting data discussed above. Big Data could aid management accounting and MCSs in particular. Before this can be done, however, data repositories must be transformed into actionable information. This middle layer between data and packaged information is where the roles of statisticians and data analysts (also known as data scientists) lie. For management accountants to use Big Data, those with expertise must first understand, mine, transform, and analyze the data. While accumulating data has proven to be easy and sometimes incidental to other activities, management cannot reap the benefits without additional resources. In the United States, financial accounting is mainly concerned with preparing financial statements in accordance with generally accepted accounting principles (GAAP) and subsequent dissemination of information to external stakeholder groups. This branch of accounting is distinguished from managerial accounting in that it provides financial information produced in alignment with a set of standards (e.g., U.S. GAAP in the United States) to stakeholders outside of the organization. Big Data could significantly influence the future of financial reporting and the evolution of generally accepted accounting principles. The forms of Big Data discussed in this paper (i.e., video, images, audio, and text) complement traditional financial information and can provide improved transparency and usefulness for decision making. For instance, relative to fixed assets, ERP systems can augment records with video clips and other types of multimedia. In this way, the user obtains a more comprehensive view of each assets condition, features, and characteristics. This improved transparency not only assists in meeting stakeholder needs, but also provides benefits to auditors addressing pertinent assertions about fixed assets. For example, an auditor seeking to test the existence assertion relative to fixed assets would face a less-challenging task if each asset record were complemented with pertinent audio, video, and textual information. Using Big Data technologies, these data can also be analyzed in the aggregate via data mining to detect outliers or other irregularities, thus assisting auditors, management, and other stakeholders. Balance sheets omit several intangible items of significance because their values are difficult to determine objectively. Such off-balance sheet assets include the customer base, human resources, commitments, product quality, vendor base, company reputation, and so forth. Because these assets differentiate organizations and deliver competitive advantages, information about these items is clearly of value to organizational stakeholders. As of 2004, U.S. company investments in intangible and tangible assets were at comparable levels. However, many countries are experiencing faster investment growth in intangibles relative to physical assets, fostering an increase in the ratio of off-balance sheet to on-balance sheet items. This creates a situation in which traditional financial statements are declining in relevance, thereby becoming progressively less useful for decision making. At the firm level, attempts could first be made to understand the general nature and characteristics of a given intangible of interest. This is precisely where Big Data can help. For example, key indicators associated with a target asset could be accumulated, processed, and analyzed via data-mining algorithms. The resulting information would have immediate value potential and could be subsequently disseminated to stakeholders. In the short run, this information might be largely qualitative and therefore supplement the notes to financial statements. In this way, stakeholders would have some access to increasingly important off-balance sheet intangible signals. Ultimately, as data collection and analytical processing evolves, substantially quantitative valuation methods could be developed for these soft assets, allowing them to appear in the actual financial statements. Big Data could play a vital role in this process. Moreover, it could affect the evolution of accounting practices, thus influencing the manner in which reporting takes place. Financial reporting is adopting a fair value orientation. For more than a decade, the Financial Accounting Standards Board (FASB) and the International Accounting Standards Board (IASB) have been working to converge U.S. GAAP and International Financial Reporting Standards (IFRS). This effort is controversial, especially given the conflicting positions in application of fair value accounting under certain conditions. For example, key differences persist in addressing fair value accounting for property, plant, and equipment. Although IFRS allows for a fair value approach and associated revaluations, U.S. GAAP mandates property, plant, and equipment be reflected at historical cost and prohibits the use of fair values except in cases of impairment. When impairment occurs, U.S. GAAP requires each affected asset be revalued to the impaired lower value. Big Data can help address the disparity between U.S. GAAP and IFRS fair value accounting and help create a global set of accounting standards. One potential method for facilitating this process entails the use of long-lived Internet software agents.1 The function of these agents would be to collect information to assist in the valuation of otherwise hard-to-value assets by using extensive automated Internet search methods running over extended periods of time. The design and implementation of long-lived Internet agents have been examined. These are described generically as software programs that assemble data useful in various decision-making routines. Agents operate continuously or periodically to collect and evaluate designated data from the cloud. As agents retrieve, process, and accumulate information, it is stored for subsequent analyses. Because agents operate frequently and generate all available information related to a phenomenon of interest, the sizes of the corresponding datasets can quickly become excessive. In the fair value accounting (FVA) estimation context, agents search all relevant web-based information to maximize Levels 1 and 2 fair value estimates with objective, observable, and current market data. If each company had this method of access, then agents could function with a standardized process in generating information for making FVA estimates. In extending this idea, data service firms specializing in providing fair value information about assets and liabilities might emerge in the marketplace, similar to current valuation firms that provide appraisal and estimation services. At one extreme, these entities would establish service agreements with companies to provide regular data and/or estimates for all assets and liabilities, and such contracts might also provide for actuarial-oriented assessments in cases where Level 3 assessments are necessary. At the other extreme, these organizations would generate data in a one-time instance for a single asset or liability. In addition, auditors might use these services in confirming the reasonableness of established fair values in a fashion similar to how auditors enlist independent experts in making basket asset valuations. Regardless of how the information acquisition process unfolded via Big Data, subjective assumptions in FVA estimates would be mitigated. For example, consider the impact FVA would have on depreciable assets. Currently, depreciation schedules reflect the change over time in value of such assets based on their estimated useful lives and presumably match the manner in which these resources contribute to revenues. However, various depreciation approaches (i.e., straight-line, accelerated methods, or units of production) are available. Furthermore, selecting a depreciation method is a discretionary accounting decision and varies among organizations. Although straight-line is the most common method, it often does not properly reflect the change in asset value over the benefit period. Vehicles in a truck fleet might be replaced based on years of service or number of miles driven, and the straight-line approach could be selected in either case to recognize the costs of the vehicles over their useful lives. However, this will typically not reflect how vehicle values change over time. In particular, there is a negative relationship between vehicle age and decline in value. Specifically, a new truck naturally incurs the greatest decrease in worth during the initial year and progressively lower decrements in value in subsequent years. A vehicle operated less frequently tends to maintain a relatively higher value, suggesting that a metric based on mere passage of time is not a good estimator of value change in such instances. FVA could resolve this problem by eliminating the need for and use of depreciation schedules that are often arbitrary. With FVA, the value of a vehicle is simply modified in each reporting period in response to relevant marketplace variables. High-quality sensors, exponential increases in storage capacity, and sophisticated algorithms have given rise to Big Data and its increasingly broad applications. However, for each company, the usefulness of the data is limited by quantity, quality, and accessibility. Many organizations cannot apply Big Data techniques simply because the entities cannot overcome a limiting factor, such as lack of data (quantity), irrelevance or data from questionable sources (quality), or insufficient expertise in extracting information (accessibility). Because of this, one of the first action items for businesses that wish to include Big Data in their accounting records is to identify the data, assess their suitability for the task at hand, and decide whether the analyses should be outsourced. Failure to properly perform this process could diminish the quality of the accounting records and underlying confidence in the financial results. Apart from the responsible handling of Big Data, companies that are defendants in lawsuits must also consider the possibility of adverse litigation outcomes, not unlike what currently happens when a plaintiffs expert arrives at a different value for damages. These latter experts would have access to Big Data archives in arriving at potential damages. Accordingly, the e-discovery issue presented via Big Data could lead to potentially increased liability for organizations. The complexity of Big Datas use in computing damages may create confusion on the part of either a judge or a jury in understanding the underlying assumptions for determining amounts to be awarded to plaintiffs. As with any change in the valuation model, this is a legitimate concern and will need to be addressed as the rise of Big Data continues.
What you agree or disagree with? Do you think their perspective is accurate?
Step by Step Solution
There are 3 Steps involved in it
Step: 1
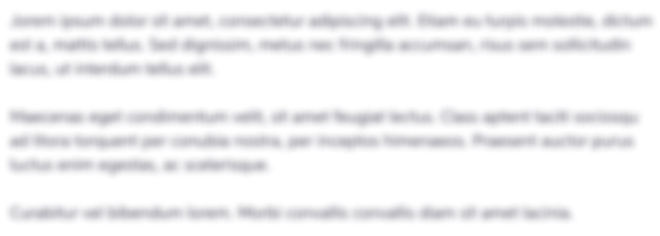
Get Instant Access to Expert-Tailored Solutions
See step-by-step solutions with expert insights and AI powered tools for academic success
Step: 2

Step: 3

Ace Your Homework with AI
Get the answers you need in no time with our AI-driven, step-by-step assistance
Get Started