Answered step by step
Verified Expert Solution
Question
1 Approved Answer
Work individually on this assignment. You are encouraged to collaborate on ideas and strategies pertinent to this assignment. Data for this assignment is focused on
- Work individually on this assignment. You are encouraged to collaborate on ideas and strategies pertinent to this assignment. Data for this assignment is focused on real estate transactions recorded from 1964 to 2016 and can be found in Housing.xlsx. Using your skills in statistical correlation, multiple regression, and R programming, you are interested in the following variables: Sale Price and several other possible predictors.
- If you worked with the Housing dataset in previous week - you are in luck, you likely have already found any issues in the dataset and made the necessary transformations. If not, you will want to take some time looking at the data with all your new skills and identifying if you have any clean up that needs to happen.
- Complete the following:
- Explain any transformations or modifications you made to the dataset.
- Create a linear regression model where "sq_ft_lot" predicts Sale Price.
- Get a summary of your first model and explain your results (i.e., R2, adj. R2, etc.)
- Get the residuals of your model (you can use 'resid' or 'residuals' functions) and plot them. What the does the plot tell you about your predictions?
- Use a qq plot to observe your residuals. Do your residuals meet the normality assumption?
- Now, create a linear regression model that uses multiple predictor variables to predict Sale Price (feel free to derive new predictors from existing ones). Explain why you think each of these variables may add explanatory value to the model.
- Get a summary of your next model and explain your results.
- Get the residuals of your second model (you can use 'resid' or 'residuals' functions) and plot them. What the does the plot tell you about your predictions?
- Use a qq plot to observe your residuals. Do your residuals meet the normality assumption?
- Compare the results (i.e., R2, adj R2, etc) between your first and second model. Does your new model show an improvement over the first? To confirm a 'significant' improvement between the second and first model, use ANOVA to compare them. What are the results?
- After observing both models (specifically, residual normality), provide your thoughts concerning whether the model is biased or not.
- Another important aspect of regression tasks is determining the accuracy of your predictions. For this section, we will look at root mean square error (RMSE), a common accuracy metric for regression models.
- Install the 'Metrics' package in R Studio
- Using the first model, we will make predictions on the dataset using the predict function. An example would look like this (will vary for you based on variable names):
- 'preds <- predict(object = modelName, newdata = dataset)'
- Use the 'rmse' function to get RMSE for the model ('rmse(actual, predicted)')
- What is the RMSE for the first model?
- Perform the same task for the second model. Provide the RMSE for the second model.
- Did the second model's RMSE improve upon the first model? By how much?
Step by Step Solution
There are 3 Steps involved in it
Step: 1
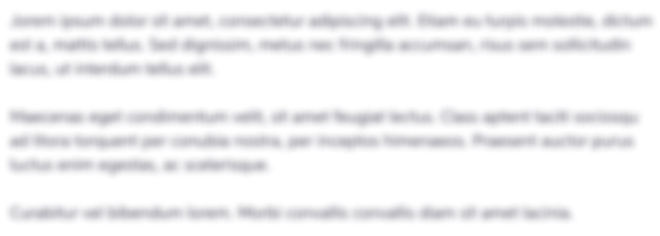
Get Instant Access to Expert-Tailored Solutions
See step-by-step solutions with expert insights and AI powered tools for academic success
Step: 2

Step: 3

Ace Your Homework with AI
Get the answers you need in no time with our AI-driven, step-by-step assistance
Get Started