Question
Write 2 paragraphs about Exchange Rate Pass-Through to Consumer Prices: The Increasing Role of Energy Prices article. No more than 100-word count. Kim, H., Lin,
Write 2 paragraphs about Exchange Rate Pass-Through to Consumer Prices: The Increasing Role of Energy Prices article. No more than 100-word count.
Kim, H., Lin, Y., & Thompson, H. (2021). Exchange Rate Pass-Through to Consumer Prices: The Increasing Role of Energy Prices. Open Economies Review, 32(2), 395-415. https://4x10hup1x-mp01-y-https-doi-org.proxy.lirn.net/10.1007/s11079-020-09601-7
Abstract A number of researchers have found that the rate of exchange rate pass-through (ERPT) to domestic prices has declined substantially over the last few decades. We revisit this claim of a shrinking exchange rate effect on the Consumer Price Index (CPI) in a vector autoregressive (VAR) model for US macroeconomic data under the current floating exchange rate regime. Our VAR approach nests the conventional single equation method and reveals statistically significant evidence of ERPT to the CPI only during later observations, sharply contrasting with previous findings. After confirming structural breaks in ERPT via statistical tests by Hansen (Journal of Economic Perspectives, 15(4), 117-128, 2001) and Qu and Perron (Econometrica, 75(2), 459-502, 2007), we seek the source with disaggregated level CPIs, and pin down a key role of energy prices. US energy imports increased from the 1990s until the recent recession. This market changes magnify the effects of the exchange rate shocks on domestic energy prices, resulting in greater responses of the total CPI via the energy price channel. Keywords Exchange rate pass through Disaggregated CPI inflation Structural break Real exchange rate shock
1 Introduction In an open economy, changes in the exchange rate can substantially influence the Consumer Price Index (CPI). In response to depreciations of the home currency, import prices may rise, triggering increases in the CPI of the home country. In addition, the relative demand for domestic products may grow through the expenditure switching effect when the home currency loses value against the foreign currency, making home products relatively cheaper. Stronger demand for domestic goods then raises home consumer prices. Rates of exchange rate pass-through (ERPT) to the CPI may change over time. Frankel et al. (2012), for instance, provide international evidence that the degree of ERPT to import goods prices, and in turn to the CPI, decreased during the 1990s especially in developing countries. Campa and Goldberg (2005) report similar evidence for 23 high-income OECD countries. Taylor (2000) claims that weaker degree ERPT to the CPI of the US in the 1990s is due to a lower inflationary environment. Gagnon and Ihrig (2004) extended Taylor's claim to 11 industrialized countries. Takhtamanova (2010) presents similar empirical evidence of structural breaks in the rate of ERPT during the 1990s for a set of fourteen OECD countries. We revisit this claim of waning degree ERPT to domestic prices in the 1990s via structural vector autoregressive (VAR) model estimations for US macroeconomic data under the floating exchange rate regime since 1973. In what follows, we provide strong evidence of time-varying ERPT. Employing a trivariate VAR with a fixedsize rolling window scheme, we estimate and report time-varying responses of CPI inflation to a real appreciation shock that confirms qualitatively different dynamics over time. Furthermore, CPI response estimates tend to be statistically significant only when earlier observations are excluded. That is, we report strong evidence of significant ERPT to CPI during the post-1990 era. We then statistically test this possibility of time-varying ERPT using Hansen (2001) sequential structural break test procedure as well as the test by Qu and Perron (2007) in a multivariate framework. We identify structural breaks in the rate of ERPT to an array of disaggregated CPIs in addition to the total CPI. We also estimate and report the ERPT parameters from the structural VAR method that nests the conventional single equation approach, which confirms time-varying ERPT. We seek the source of the structural break by looking at the disaggregated CPI responses to the real exchange rate shock with impulse-response function analysis. We demonstrate that the time-varying responses of the total CPI are driven mainly by the responses of the energy-related CPIs. That is, energy prices play an important role in explaining the structural break. We note that the dependency of US oil consumption on imports exhibits overall a positive trend with a dip in the 1980s, then continued to increase from around 1990 until the recent recession, which may explain the structural breaks via the energy price channel. We confirm this conjecture using a quad-VAR model that uncovers a strong oil price channel that propagates the exchange rate shock to the CPI. The remainder of this paper is organized as follows. Section 2 presents our baseline empirical model. We also explain how our multivariate VAR approach for ERPT 396 H. Kim et al. nests the conventional single equation method. Section 3 provides preliminary analysis of time-varying ERPT as well as formal structural break test results. In Section 4, we implement the impulse-response function analysis that unveils an important role of energy prices in explaining the source of the break. Section 5 concludes. 2 The Empirical Model We employ a recursively identified vector autoregressive (VAR) process of order q to study the dynamic exchange rate effects on consumer prices in the US. Abstracting from deterministic terms, we propose the following model.1 xt = q j=1 Bj xtj + Cut, (1) where xt = [st yt pt] , C denotes a lower-triangular (Choleski factor) matrix, and ut is a vector of mutually orthonormal structural shocks, that is, Eutu t = I. st denotes the real exchange rate, yt is the real GDP, and pt is the Consumer Price Index (CPI). All variables are log transformed and differenced, so positive values of st , for example, denote real appreciations of the US dollar. We are particularly interested in the j -period ahead orthogonalized impulseresponse function (IRF) of domestic CPI inflation (pt) to the structural shock to st that occurs at time t. That is, (j ) = E pt+j |us,t = 1, t1 E pt+j |t1 , (2) where E(|t1) is the conditional expectation operator given the information set t1 at time t 1, and us,t is the 1% shock to st at time t. 2 The j -period ahead level CPI response is obtained by the following cumulative sum. (j ) = j s=0 (s) (3) Econometric inferences from recursively identified VAR models such as (1) may not be robust to alternative VAR ordering. It should be noted, however, that we use a partial identification scheme based on the Proposition 4.1 in Christiano et al. (1999), implying that our empirical analysis does not suffer from this criticism given the fixed location of st in xt as we are interested only in exchange rate pass-through (ERPT) to consumer prices. That is, all IRF estimates to the exchange rate (st) 1We demean the VAR prior to estimations. 2The information set t is adaptive in the sense that j j1, j . Exchange Rate Pass-Through to Consumer Prices... 397 shock including (j ) and (j ) are numerically identical even if one randomly reshuffles the variables next to st in (1).3 Put it differently, the location of pt is irrelevant when we estimate ERPT via the IRF estimations. One may estimate = s,0...s,q y,0...y,q p,1...p,q from the following univariate equation to measure the degree of ERPT. See Takhtamanova (2010) and Campa and Goldberg (2005), among others.4 pt = q j=0 s,jstj + q j=0 y,jytj + q j=1 p,jptj + ut (4) The long-run measure of ERPT is then defined as, ERP T = q j=0 s,j 1 q j=1 p,j , (5) while s,0 in (4) provides information on the short-run ERPT.5 It should be noted that the VAR model in (1) with xt = [st yt pt] nests this conventional measure of ERPT in (5). After we obtain the Choleski factor (C) from the reduced form estimation of (1), we pre-multiply each side of (1) by C1 to recover the structural form VAR: A0xt = q j=1 Aj xtj + ut, (6) where A0 = C1 and Aj = C1Bj . By dividing the third equation of the VAR system (6) by the (3, 3) component of A0, we obtain the following normalized equation that is equivalent to (4). pt = q j=0 s,jstj + q j=0 y,jytj + q j=1 p,jptj + t (7) Note that parameter estimates for = s,0...s,q y,0...y,q p,1...p,q in (7) are not the same as parameters in (4) in finite samples, because is estimated via the straightforward least squares regression for (4), while is estimated from the structural VAR model (1) utilizing a recursive identification scheme with the Choleski decomposition. In addition to the conventional measure of ERPT, q j=0 s,j 1q j=1 p,j , our approach also allows dynamic measures of ERPT at any point of time horizon j that can be obtained via the impulse-response function (j ). 6 3The IRF estimates E yt+j |us,t = 1, t1 E yt+j |t1 are also numerically identical even if yt and pt switch their location. 4Additional control variables can be added in the right hand side of the equation, which can be also easily nested by VAR models following the procedure in the present paper. 5One may estimate the standard error of (5) via the Delta method given the least squares estimation of (4), while our structural VAR approach allows us to estimate it using nonparametric bootstrap replications. 6When the ordering is [st pt yt] , (7) remains the same but y,0 = 0 by construction. 398 H. Kim et al. 3 The Empirics 3.1 Data Descriptions We obtained most data from the Federal Reserve Economic Data (FRED) website. Observations span from 1973:I to 2017:IV during the post-Bretton Woods system. That is, we focus on exchange rate pass-through (ERPT) during the current floating exchange rate regime. yt is the US real Gross Domestic Product (GDPC1), st denotes the real tradeweighted US dollar index with major currencies (TWEXMPA), and pt is the Consumer Price Index (CPI: CPIAUCSL). In addition to the total CPI (CPIAUCSL), we consider an array of disaggregated CPI sub-indices including: Food CPI (CPIUFDSL); Housing CPI (CPIHOSSL); Apparel CPI (CPIAPPSL); Transportation CPI (CPITRNSL); Medical Care CPI (CPIMEDSL); Energy CPI (CPIENGSL); All Items less Energy CPI (CPILEGSL); All Items less Food CPI (CPIULFSL); All Items less Food and Energy CPI (CPILFESL). In what follows, we use these disaggregate level CPIs to search for the source of the structural break in the rate of ERPT to the total CPI. We also use the spot crude oil price (West Texas Intermediate: WTISPLC) in our extended VAR models. We deflated this nominal crude oil price by the US CPI to get the real oil price ropt . We transformed monthly frequency data (st , pt , ropt) to quarterly frequency by taking end of period values to match the quarterly frequency of yt . All data are log differenced to ensure the stationarity of the VAR model (1). 3.2 Time-Varying ERPT We revisit the claim that the degree of ERPT to the CPI has waned during the 1990s. See among others, Frankel et al. (2012), Takhtamanova (2010) , Campa and Goldberg (2005), and Gagnon and Ihrig (2004) for detailed discussion. To investigate such possibility, we estimate the VAR model (1) repeatedly using a fixed-size rolling window scheme described as follows. We employ the rolling-window scheme instead of the recursive scheme because we are particularly interested in detecting structural changes in the data generating process of xt over time. We begin with an estimation for the VAR model (1) using the first T0(< T ) observations, {xt} T0 t=1. After obtaining the first round estimate for (3), we move the sample period window forward by one, adding one new observations at time T0 + 1 (xT0+1) but dropping the oldest ones at time t = 1 (x1). Note that the second round estimate utilizes {xt} T0+1 t=2 that contains the same number of observations T0. We repeat until we obtain the last round IRFs using {xt} T t=T T0+1, totalling T T0 + 1 sets of the IRF estimates. The left panel of Fig. 1 reports the CPI response estimates to a real appreciation shock with a 20-year (T0 = 80 quarters) fixed-size rolling window scheme.7 The range of the x-axis (Date) is from 1992:IV to 2017:IV, where each point indicates the 7We also implemented the same analysis with a 30-year window scheme. Results are qualitatively similar and are available upon requests. Exchange Rate Pass-Through to Consumer Prices... 399 ending period of each rolling window. For example, the IRF estimate on 1992:IV is based on observations between 1973:I and 1992:IV. The y-axis (Year) is the annualized time horizon (j ) of the response function indexed from 0 to 5 years. The z-axis is the level CPI response (j ) to a 1% real appreciation shock. This surface graph reveals dramatic shifts of the CPI responses to the real exchange rate shock over time. Note that CPI positively responds for about one year when the first 20-year long observations were used, while solid negative responses started to appear as we move the sample window forward. In order to highlight these dramatic transitions over time, we dissect this surface graphs at y = 0, 1, 5 (years) of the y-axis from the right to the left, which demonstrates the time-varying responses of the total CPI in the short-, medium-, and long-run. See the right panel of Fig. 1. It should be noted that the responses at impact (y = 0) and in 1 year show a clear negative slope, implying that the US CPI started to respond more negatively to the real exchange rate shock over time. In what follows, we relate this phenomena with greater consumption dependency on foreign oil production in the US. Fig. 1 Time-Varying CPI Responses to the Exchange Rate Shock. Note: We employed a 20-year fixedsize rolling window scheme to estimate time-varying IRFs. That is, we repeat IRF estimations by updating observations but maintaing the same sample size. The first set IRFs are from the sample period from 1973:I to 1992:IV, while the last ones are from 1998:I to 2017:IV. The right graph is obtained by slicing the left graph at fixed points on the year axis, which provides time-varying responses at the impact (0), 1 year, and 3 years, respectively. Responses in blue denote positive values, while green responses are negative ones 400 H. Kim et al. One quick reasoning is as follows. US dollar appreciation may result in decreases in energy prices because world commodities are denominated in dollars. When US dependency on energy imports increases, declining energy prices may result in more influential negative effects on US CPI through cheaper import goods. It should be noted that our inferences based on Fig. 1 can be limited because this surface graph is based on point estimates. To highlight the statistical significance of the response function estimates, we report the responses of the CPI in two subsample periods, 1973:I-1989:IV and 1990:I-2017:IV. As can be seen in Fig. 2, we obtain statistically significant negative responses of the total CPI to the real exchange rate shock only in the post-1990 (1990:I-2017:IV) sample. When we employ the pre-1990 (1973:II-1989:IV) sample period, however, the total CPI responds initially positively then rapidly declines below zero. Furthermore, it comes with a very wide 90% confidence band. These qualitatively different responses imply the presence of time-varying rates of ERPT to the CPI in the US. Fig. 2 CPI Responses to the Exchange Rate Shock from Sub-Samples. Note: We estimate the impulseresponse function of the level CPI to the real exchange rate shock from the trivariate VAR system with the real exchange rate, the real GDP, and the CPI after log-differencing each variable. The pre-1990 sample period is from 1973:II to 1989:IV, while the post-1990 begins in 1990:I and ends in 2017:IV. We set the number of lag to 2. The 90% confidence bands were constructed by taking the 5 and 95 percentiles from 5,000 nonparametric bootstrap simulations using the empirical distribution Exchange Rate Pass-Through to Consumer Prices... 401 3.3 Structural Break in Exchange Rate Pass-Through to CPI Inflation This section statistically evaluates the possibility of the existence of structural breaks using an array of statistical tests under both a univariate and a multivariate equation frameworks. 3.3.1 Univariate Structural Break Test We first implement an array of econometric tests for (7), employing the sequential test procedure suggested by Hansen (2001). Let R = R s,0...R s,q R y,0...R y,q R p,1...R p,q , R = 1, 2 for the following stochastic process. pt = q j=0 R s,jstj + q j=0 R y,jytj + q j=1 R p,jptj + t, R = 1, 2, (8) where = 1, t = 0, 1 = 2, t = 1, T , under the alternative hypothesis HA. We begin the procedure by testing the null hypothesis H0 : 1 = 2, employing the following three test statistics proposed by Andrews (1993) and Andrews and Ploberger (1994) for the full sample (T ), SupFT = sup k1kk2 FT (k) (9) ExpFT = ln 1 k2 k1 + 1 k2 t=k1 exp 1 2 FT (k) AveFT = 1 k2 k1 + 1 k2 t=k1 FT (k), where FT (k) denotes the Lagrange multiplier (LM) test statistics of the null hypothesis of no structural change at each fine grid point k [k1, k2]. 8 We use conventional trimming parameter values, k1 = 0.15T and k2 = 0.85T . Associated p values are obtained by numerical approximations to the asymptotic distributions of the test statistics using the method proposed by Hansen (1997). When the test rejects the null hypothesis of no structural break at time t = 1, we repeat the test for the resulting two sub-sample periods, [0, 1) and 1, T , for the existence of additional structural break date, say t = 2, in each sub-sample. If the test identifies a break in [0, 1), we implement the test for the two sub-sample 8Alternatively, the Wald or the Likelihood Ratio statistics can be used. 402 H. Kim et al. periods, [0, 2) and 2, 1 for another structural break at time t = 3 in each subperiod. If the test fails to identify a break in [0, 1), we stop looking for break dates in that region. We repeat this procedure for the other sub-sample period 1, T until the test fails to reject the null hypothesis. We choose the number of lags by the Akaike Information Criteria (AIC) with a maximum 4 lags. As can be seen in Table 1, we obtained strong evidence of a structural break from the full sample period for all CPIs with exceptions of the CPIs that exclude energy prices, that is, the All less Energy CPI and the All less Food and Energy CPI. For example, the null hypothesis of no structural break was rejected by all three tests (9) for the All item CPI at the 1% significance level, while all three tests fail to reject the null hypothesis even at the 10% significance level for the All less Food and Energy CPI. We report the identified break date from the SupFT test in Table 1 that selects the break date around the late 1980s whenever the test rejects the null hypothesis. The test fails to reject the null hypothesis for the sub-sample periods implied by the chosen break date from the first test at any conventional significance level.9 That is, our structural break tests identified a single statistically significant break date for most CPIs other than the ones that exclude energy prices. These findings imply that the structural break in the total CPI may be mainly due to the break in the Energy CPI. 3.3.2 Multivariate Structural Break Test We next implement structural break tests by Qu and Perron (2007) for a reduced form representation of xt in (1), which employs a quasi-maximum likelihood approach with Gaussian errors for, xt = q j=1 Bj xtj + t, (10) where t is a 3 by 1 vector of error terms. Note that the order of variables doesn't matter because (10) is a reduced form. Note also that this procedure tests the existence of structural breaks in the coefficients of all three equations instead of the single equation similar to (8) with no contemporaneous terms. We first use Qu and Perron (2007) Seq(l + 1|l) test to identify the number of structural breaks as well as estimating the corresponding break dates. We implement this procedure via sequentially testing the null hypothesis of l structural breaks with the alternative hypothesis of l+1 breaks. After pinning down the number of structural breaks with the 5% significance level, we test the null hypothesis of no break against this chosen number of structural breaks using their SupLRT test. Table 2 reports our key results for q = 1 with a maximum 3 breaks. Recall that the univariate test in Table 1 provided evidence of no more than one break. Qu and Perron (2007) procedure, however, identified two or more structural breaks for 7 out 9These results are available upon request. Exchange Rate Pass-Through to Consumer Prices... 403 Table 1 Structural break test results: single equation approach CPI Break Date SupFT ExpFT AveFT All item 1989Q1 41.24 17.51 29.99 Food 1989Q1 31.53 13.24 22.97 Housing 1982Q1 28.59 12.19 20.34 Apparel 1992Q3 32.30 13.20 18.48 Transportation 1988Q4 26.44 10.62 18.99 Medical Care 1989Q4 33.20 13.30 21.83 Energy 1988Q4 21.75 8.73 15.67 All less Energy 1983Q1 26.77 11.17 19.99 All less Food 1988Q3 33.52 14.25 23.92 All less F&E 1983Q1 27.99 11.14 19.16 We follow the structural break test procedure suggested by Hansen (2001) by applying the tests Hansen (1997) sequentially for the sub-sample periods chosen by the break date estimates. *, , and denote a rejection at the 10%, 5%, and 1% significance level, respectively, based on p values were obtained using Hansen's GAUSS codes. The number of lags was chosen by the Akaike Information Criteria (AIC) with a maximum 4 lags Table 2 Structural break test results: multivariate regression approach Break Date Estimates Structural Break Test CPI BD#1 BD#2 #BD#3 Seq(2|1) Seq(3|2) SupLRT All item 1980Q3 1990Q3 2001Q1 24.62 24.33 91.32 Food 1980Q2 N.A. N.A. 12.52 20.66 39.84 Housing 1982Q3 2007Q4 N.A. 27.62 16.59 53.48 Apparel 1992Q4 NA N.A. 18.16 7.89 48.28 Transportation 1980Q4 2001Q1 N.A. 29.41 11.00 37.29 Medical Care 1982Q4 1993Q1 2007Q3 26.61 25.63 85.95 Energy 2001Q1 N.A. N.A. 21.68 21.68 23.81 All less Energy 1982Q3 1990Q4 N.A. 40.70 8.11 48.49 All less Food 1982Q1 2001Q1 N.A. 25.12 11.28 89.79 All less F&E 1982Q3 1992Q3 2001Q3 43.13 24.62 54.13 We implement the structural break test for multivariate regressions by Qu and Perron (2007) with a maximum 3 breaks. We use a bivariate VAR system with log-differenced exchange rate and CPI. We determined the number of breaks using the Seq(l+1|l) test with the 5% signficance level. SupLRT statistics are for the chose number of breaks by the sequential test against the zero break null hypothesis. *, , and denote a rejection at the 10%, 5%, and 1% significance level, respectively, based on critical values that are obtained using Qu's GAUSS codes. The confidence bands for the break date are available upon request 404 H. Kim et al. of 10 CPIs, which makes sense because the test estimates break dates for any of the three equations in (10). The Seq(l+1|l) test rejects H0 : l = 2 and H0 : l = 1 at the 5% significance level for the total CPI, implying that there are three structural breaks under the current specification with a maximum 3 breaks. The SupLRT test rejects the null of no break against three breaks at the 1% significance level. The estimated structural break dates are in 1980:III, 1990:III, and 2001:I. Note that the second break date is roughly coincide with the univariate test result in Table 1. It should be noted that these break dates are based on all three equations for the real exchange rate and the real GDP in addition to the CPI. On the other hand, the Seq(l + 1|l) test fails to reject the null of l = 1 and 2 for the Food CPI, but the SupLRT test rejects the null of no break at the 1% significance level, implying that the test procedure identifies one statistically significant break at 1980:II. As can be seen in Table 2, the test identified a break date around the early 1980s for 8 out of 10 CPIs. Since our observations begin in 1973:I, it is difficult to obtain reliable ERPT estimates for the pre-1980 sample period that provides less than 10-year long quarterly observations for our VAR system. Therefore, we report our statistical inferences on ERPT estimates in the next section mainly based on the two similar length sub-sample periods, the pre-1990 and the post-1990 sample periods. 4 Searching for the Source of the Break 4.1 Time-Varying Degree of ERPT to CPI Confirming the statistical evidence of structural breaks in ERPT to the CPIs, we now seek the source of the structural break by investigating disaggregated level CPI responses to the real exchange rate shock via impulse-response function analysis. As shown in the previous section, the univariate structural break test procedure by Hansen (2001) identified the end of the 1980s as a common statistically significant single break date for most CPIs. The multivariate tests by Qu and Perron (2007) confirmed this break date as well as up to two other break dates. Based on these results, we estimate the degree of ERPT focusing on the two sub-sample periods, the pre-1990 (1973:I-1989:IV) and the post-1990 (1990:I-2017:IV) eras. Also, we considered 1983 as alternative specification of a structural break that allows two sub-sample periods, the pre-1983 and the post-1983, providing10-year long observations for the earlier sub-sample period. We obtained qualitatively similar results from this specification. Results are reported in the abstract. Based on the pre- and the post-1990 sample periods, we report the ERPT parameter estimates from the IRF approach in Table 3, while those from the structural VAR coefficient approach appear in Table 4. 10 Major findings are as follows. 10We obtain the long-run rate of ERPT from the IRF approach by taking the value of (20). j = 20 (quarter) seems to be sufficient for the IRF to become stabilized. Exchange Rate Pass-Through to Consumer Prices... 405 Table 3 ERPT Estimates: IRF Approach xt = q j=1 Bj xtj + Cut, xt = [st yt pt] (j ) = E pt+j |us,t = 1, t1 E pt+j |t1 , (j ) = j s=0 (s) Pre-1990 Post-1990 CPI ERP T CI ERP T CI All item 0.142 [0.637, 0.286] 0.139 [0.209, 0.081] Food 0.125 [0.400, 0.110] 0.152 [0.255, 0.064] Housing 0.019 [0.458, 0.508] 0.064 [0.145, 0.008] Apparel 0.018 [0.213, 0.172] 0.029 [0.084, 0.144] Transportation 0.177 [0.369, 0.791] 0.708 [0.998, 0.475] Medical Care 0.153 [0.026, 0.375] 0.013 [0.138, 0.155] Energy 0.448 [0.670, 1.782] 1.390 [1.980, 0.893] All less Energy 0.036 [0.345, 0.265] 0.065 [0.143, 0.011] All less Food 0.007 [0.469, 0.455] 0.152 [0.230, 0.087] All less F&E 0.028 [0.259, 0.336] 0.014 [0.089, 0.051] We estimate the impulse-response function of the level CPI to the real exchange rate shock from the trivariate VAR system with the real exchange rate, the real GDP, and the CPI after log-differencing each variable. The pre-1990 sample period is from 1973:II to 1989:IV, while the post-1990 begins in 1990:I and ends in 2017:IV. We set the number of lag to 2. The 90% confidence bands were constructed by taking the 5 and 95 percentiles from 5,000 nonparametric bootstrap simulations using the empirical distribution. The long-run effect ERPT is the 20-period ahead response of the level CPI, which is sufficient for the IRFs to get stabilized Table 4 ERPT Estimates: VAR Coefficients Approach xt = q j=1 Bj xtj +Cut, xt = [st yt pt] pt = q j=1 p,j ptj + q j=0 s,j stj + q j=0 y,j ytj + t ERP T = q j=0 s,j 1q j=1 p,j Pre-1990 Post-1990 CPI ERP T CI ERP T CI All item 0.179 [1.485, 0.602] 0.134 [0.192, 0.081] Food 0.137 [0.326, 0.027] 0.169 [0.292, 0.069] Housing 0.032 [0.874, 0.861] 0.063 [0.150, 0.013] Apparel 0.018 [0.168, 0.141] 0.006 [0.094, 0.108] Transportation 0.149 [0.352, 0.608] 0.661 [0.848, 0.479] Medical Care 0.087 [0.011, 0.205] 0.016 [0.145, 0.239] Energy 0.328 [0.582, 1.268] 1.285 [1.693, 0.882] All less Energy 0.031 [0.379, 0.231] 0.068 [0.172, 0.008] All less Food 0.018 [0.896, 0.712] 0.143 [0.206, 0.085] All less F&E 0.026 [0.377, 0.385] 0.009 [0.085, 0.064] We estimate the impulse-response function of the level CPI to the real exchange rate shock from the trivariate VAR system with the real exchange rate, the real GDP, and the CPI after log-differencing each variable. The pre-1990 sample period is from 1973:II to 1989:IV, while the post-1990 begins in 1990:I and ends in 2017:IV. ERPT is constructed using the structural coefficient estimates from the VAR system. We set the number of lag to 2. The 90% confidence bands were constructed by taking the 5 and 95 percentiles from 5,000 nonparametric bootstrap simulations using the empirical distribution 406 H. Kim et al. First, we obtain statistically insignificant estimates for all CPIs during the pre1990 era, while estimates from the post-1990 era are highly significant for 6 out of 10 CPIs regardless of approaches. These findings imply strong and statistically significant evidence of ERPT to CPIs only in the later sample period. Furthermore, all significant ERPT parameter estimates turn out to be negative, implying that real appreciations of the US dollar result in a decrease in CPIs as discussed in the introduction. We obtained qualitatively similar results from sub-sample analysis with 1983 as the structural break. See Tables 5 and 6 in the appendix. Second, we confirm a weaker rate of ERPT to the total CPI during the post-1990 era, consistent with Frankel et al. (2012), Takhtamanova (2010), McCarthy (2007), Campa and Goldberg (2005), Gagnon and Ihrig (2004), and Taylor (2000) who claim that the degree of ERPT became substantially weaker in the 1990s. The ERPT point estimate with the total CPI from the VAR coefficient approach (see Table 4) were 0.179 and 0.134 for the pre- and the post-1990 eras, respectively. Although these point estimates are consistent with the previous works in the current literature, it should be noted that the estimates are statistically significant only for the post-1990 sample period. We obtain similar results from the IRF approach as can be seen in Table 3. Tables 5 and 6 provide similar evidence with an alternative break date in 1983. Putting it all together, our findings cast serious doubt on the validity of previous findings because our structural estimation approaches reveal a high degree of uncertainty in the linkage between the exchange rate and the CPI during the pre-1990 era. Third, ERPT estimates tend to be greater in absolute value for CPIs with food and energy prices during the post-1990 era. ERPT estimates for the Housing, Apparel, and the Medical Care CPIs are not only quantitatively smaller but also statistically insignificant, meaning that the significant and strong rate of ERPT to the total CPI is mainly driven by the strong degree of ERPT to some of its sub-category CPIs such as the Energy CPI, the Transportation CPI, and the Food CPI. It should be noted that the ERPT estimate becomes statistically insignificant and negligibly small when food and energy prices are excluded (All less F&E CPI). Note also that the ERPT estimate of the CPI without Food is greater than that of the CPI without Energy, even though they are both significant, implying a stronger role of the Energy CPI compared with the Food CPI in contributing to ERPT to the total CPI during the post-1990 era. 4.2 Time-Varying Responses of Disaggregated CPIs This subsection further investigates the source of the structural break in ERPT to the total CPI by investigating the IRFs of disaggregated level CPIs to the real exchange rate shock in the pre- and the post-1990 eras. We note that all CPIs for the pre-1990 era in Fig. 3 exhibit overall insignificant responses. We obtained insignificant negative ERPT only to the Food CPI during the pre-1990 era, which implies that the negative responses of the total CPI we observed in Fig. 2 should have been driven solely by ERPT to the Food CPI given virtually negligible responses of other CPIs. On the other hand, we observed statistically significant negative responses of the Food CPI, Transportation CPI, and the Energy CPI Exchange Rate Pass-Through to Consumer Prices... 407 Fig. 3 Disaggregated CPI Responses to the Exchange Rate Shock. Note: We estimate the impulseresponse function of the level CPI to the real exchange rate shock from the trivariate VAR system with the real exchange rate, the real GDP, and the CPI after log-differencing each variable. The pre-1990 sample period is from 1973:II to 1989:IV, while the post-1990 begins in 1990:I and ends in 2017:IV. We set the number of lag to 2. The 90% confidence bands were constructed by taking the 5 and 95 percentiles from 5,000 nonparametric bootstrap simulations using the empirical distribution 408 H. Kim et al. during the post-1990 era. Responses were negligibly weak and overall insignificant for Housing CPI, Apparel CPI, and Medical CPI. Interestingly, as can be seen in Fig. 4, the All less Energy CPI and the All less Food CPI exhibit significantly negative responses during the post-1990 era, while the All less Food and Energy CPI shows no evidence of ERPT during the same sample period. These responses jointly imply that both food and energy prices play an important role in explaining ERPT to the total CPI during the post-1990 era. It should be also noted that the Energy CPI and its related prices such as the Transportation CPI play a key role in explaining the existence of the structural break in ERPT to the total CPI. This is because ERPT to the Food CPI was observed in both sub-sample periods, whereas we observe more significant evidence only for the post1990 sample period. Unlike the Food CPI, the Energy CPI responses are qualitatively different across the two sample periods. That is, we observe negative responses of the Energy CPI only in the post-1990 sample period, resembling responses of the total CPI to the exchange rate shock. To put it differently, we pinned down the structural Fig. 4 Special CPI Responses to the Exchange Rate Shock. Note: We estimate the impulse-response function of the level CPI to the real exchange rate shock from the trivariate VAR system with the real exchange rate, the real GDP, and the CPI after log-differencing each variable. The pre-1990 sample period is from 1973:II to 1989:IV, while the post-1990 begins in 1990:I and ends in 2017:IV. We set the number of lag to 2. The 90% confidence bands were constructed by taking the 5 and 95 percentiles from 5,000 nonparametric bootstrap simulations using the empirical distribution Exchange Rate Pass-Through to Consumer Prices... 409 change in ERPT to the Energy CPI as the source of the structural break in ERPT to the total CPI in the US. 4.3 Role of Energy Prices in Explaining the Break The previous section presented the important role of energy prices in explaining the break in ERPT to the total CPI in the US. To better understand this phenomenon, we report the share of the US petroleum net imports in consumption in Fig. 5. 11 The share exhibits a positive trend until 1978 followed by decreases until the mid 1980s, which seems to correspond to the alternative break date near 1983 estimated via the procedure of Qu and Perron (2007). After that, the share rapidly went up again until the emergence of the US financial crisis in 2006. The import share in consumption averaged 24% during the pre-1990 era before increasing to its peak of 60% in 2006. Since then, it started to decline reflecting: (1) decreased domestic consumption triggered by the US financial crisis followed by the Great Recession, and (2) rapidly increasing domestic production due to hydraulic fracturing. Nonetheless, the import share in the post-1990 of 46% is nearly double its earlier level. The increased integration of the US into the international energy market since 1990 is consistent with stronger exchange rate effects on domestic energy prices. Given that, demonstrating a strong link between energy prices and the total CPI will help understand the emergence of the structural break in the pattern of ERPT via the energy price channel. To investigate this possibility, we report the IRFs from the following quad-variate VAR system. xt = q j=1 Bj xtj + Cut, (11) xt = [st ropt yt pt] , where ropt is the log real oil price. Results are reported in Fig. 6. Note that the location of ropt in xt doesn't matter as long as we are interested in the effect of the exchange rate shock on the other variables. The real oil price responds significantly negatively to the real exchange rate shock during the post-1990 era, while it exhibits insignificant positive responses during the earlier period. The real US GDP responses are overall insignificantly negative in both periods, and are quantitatively negligible. The responses of the total CPI and the Energy CPI are similar to each other and resemble those of the real oil price, confirming our claim that the break in the pattern of ERPT to the total CPI is caused by the break in the response of the oil price to the exchange rate shock. 11We obtained the data from the U.S. Energy Information Administration website. 410 H. Kim et al. Fig. 5 Consumption Dependency on Foreign Petroleum. Note: We obtained the data from the Energy Information Agency (EIA) .EIA uses product supplied as a proxy for U.S. petroleum consumption. The share (%) is the U.S. petroleum net imports divided by domestic consumption As a robustness check, we also implement the structural break test for the total CPI with a quad-variate VAR model as follows. pt = q j=0 R s,j stj +q j=0 R o,j roptj +q j=0 R y,j ytj +q j=1 R p,j ptj + t, R = 1, 2, (12) We applied Hansen (2001) sequential test procedure for this VAR, which rejected the null hypothesis again at least at the 8% significance level, and identifies two break dates, 1993:III and 2006:IV. The first date is roughly consistent with our previous findings from the trivariate model, while the second one corresponds to the beginning of the recent US financial crisis that triggered rapid decreases in the consumption dependency on foreign energy products (see Fig. 5). These findings strengthen our claim of an important role of energy prices in explaining time-varying ERPT to the total CPI in the US.12 12Detailed results are available upon requests. Exchange Rate Pass-Through to Consumer Prices... 411 Fig. 6 IRF Analysis with the Real Oil Price. Note: We estimate the impulse-response function of the level CPI to the real exchange rate shock from the quadvariate VAR system with the real exchange rate, the real oil price, the real GDP, and the CPI. We log-differenced all variables prior to estimations. The pre-1990 sample period is from 1973:II to 1989:IV, while the post-1990 begins in 1990:I and ends in 2017:IV. We set the number of lag to 2. The 90% confidence bands were constructed by taking the 5 and 95 percentiles from 5,000 nonparametric bootstrap simulations using the empirical distribution 5 Concluding Remarks This paper revisits the claim of a decreasing rate of exchange rate pass-through (ERPT) to the CPI, employing a recursively identified vector autoregressive (VAR) model for US macroeconomic data during the current floating exchange rate regime. Our findings sharply contrast with those from earlier works including Frankel et al. (2012), Takhtamanova (2010), and Campa and Goldberg (2005), that mostly employed the univariate regression approach. We also demonstrate that our VAR approach allows richer statistical analyses but is flexible enough to nest the single equation approach. Our findings suggest some evidence of a greater rate of ERPT to the total CPI in the pre-1990 sample period, which is consistent with earlier work. However, our multivariate VAR approach reveals high uncertainty on the ERPT estimation during that period, which makes statistical inferences on ERPT difficult. On the other hand, we report highly significant negative responses of the total CPI to real exchange rate shock during the post-1990 era. These qualitatively different responses across the 412 H. Kim et al. two eras imply the possibility of a structural break in the pattern of ERPT to the CPI in the US. Employing the univariate and multivariate structural break tests, we confirm the existence of a structural break at the end of the 1980s. Then, we seek the source of the structural break by investigating the impulse-response function estimates of the disaggregate level CPIs to the real exchange rate shock. Our empirical results show that energy prices play a key role in explaining the change in the pattern of ERPT to the total CPI. We point out that the energy sector of the US economy became more involved with the international market since the 1990s. Greater interdependence amplifies the effect of exchange rate shocks on domestic energy prices, resulting in greater responses of the total CPI via the energy price channel. Energy prices play a key role in explaining the structural break in ERPT to the total CPI in the US.
Step by Step Solution
There are 3 Steps involved in it
Step: 1
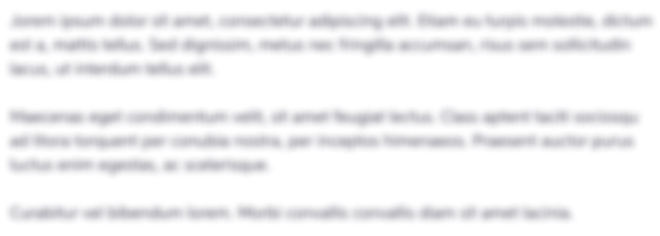
Get Instant Access to Expert-Tailored Solutions
See step-by-step solutions with expert insights and AI powered tools for academic success
Step: 2

Step: 3

Ace Your Homework with AI
Get the answers you need in no time with our AI-driven, step-by-step assistance
Get Started