Answered step by step
Verified Expert Solution
Question
1 Approved Answer
xloadscores, yloadscores = PLSRmodel.x_loadings_, PLSRmodel.y_loadings_ plt.xlabel('Principal compenent 1) plt.ylabel('Principal component 2 ') plt.scatter (xloadscores [:,0],x loadscores [:,1],c=bb, marker='^') plt.scatter(yloadscores [:,0], yloadscores [:,1],c=r, marker='o') plt.annotate('LDH', (yloadscores
Step by Step Solution
There are 3 Steps involved in it
Step: 1
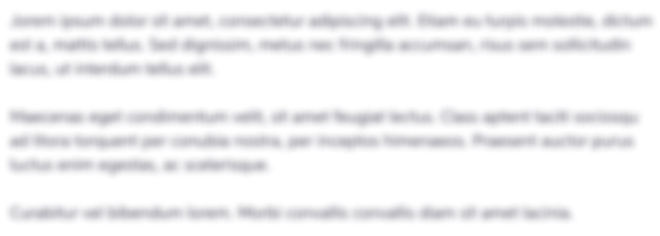
Get Instant Access to Expert-Tailored Solutions
See step-by-step solutions with expert insights and AI powered tools for academic success
Step: 2

Step: 3

Ace Your Homework with AI
Get the answers you need in no time with our AI-driven, step-by-step assistance
Get Started