Question
You would like to build a classifier for an Autism early detection application. Each data point in the dataset represents a patient. Each patient is
You would like to build a classifier for an Autism early detection application. Each data point in the dataset represents a patient. Each patient is described by a set of attributes such as age, sex, ethnicity, communication and development figures, etc. You know from domain knowledge that autism is more prevalent in males than females.
If the dataset you are using to build the classifier is noisy, contains redundant attributes and missing values. If you are considering a decision tree classifier and a k-nearest neighbor classifier, explain how each of these can handle the three mentioned problems:
1. Noise
2. Missing Values
3. Redundant Attributes
Step by Step Solution
There are 3 Steps involved in it
Step: 1
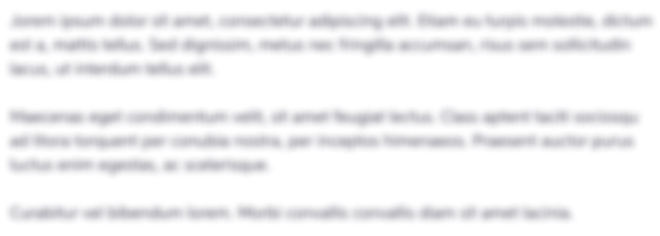
Get Instant Access to Expert-Tailored Solutions
See step-by-step solutions with expert insights and AI powered tools for academic success
Step: 2

Step: 3

Ace Your Homework with AI
Get the answers you need in no time with our AI-driven, step-by-step assistance
Get Started