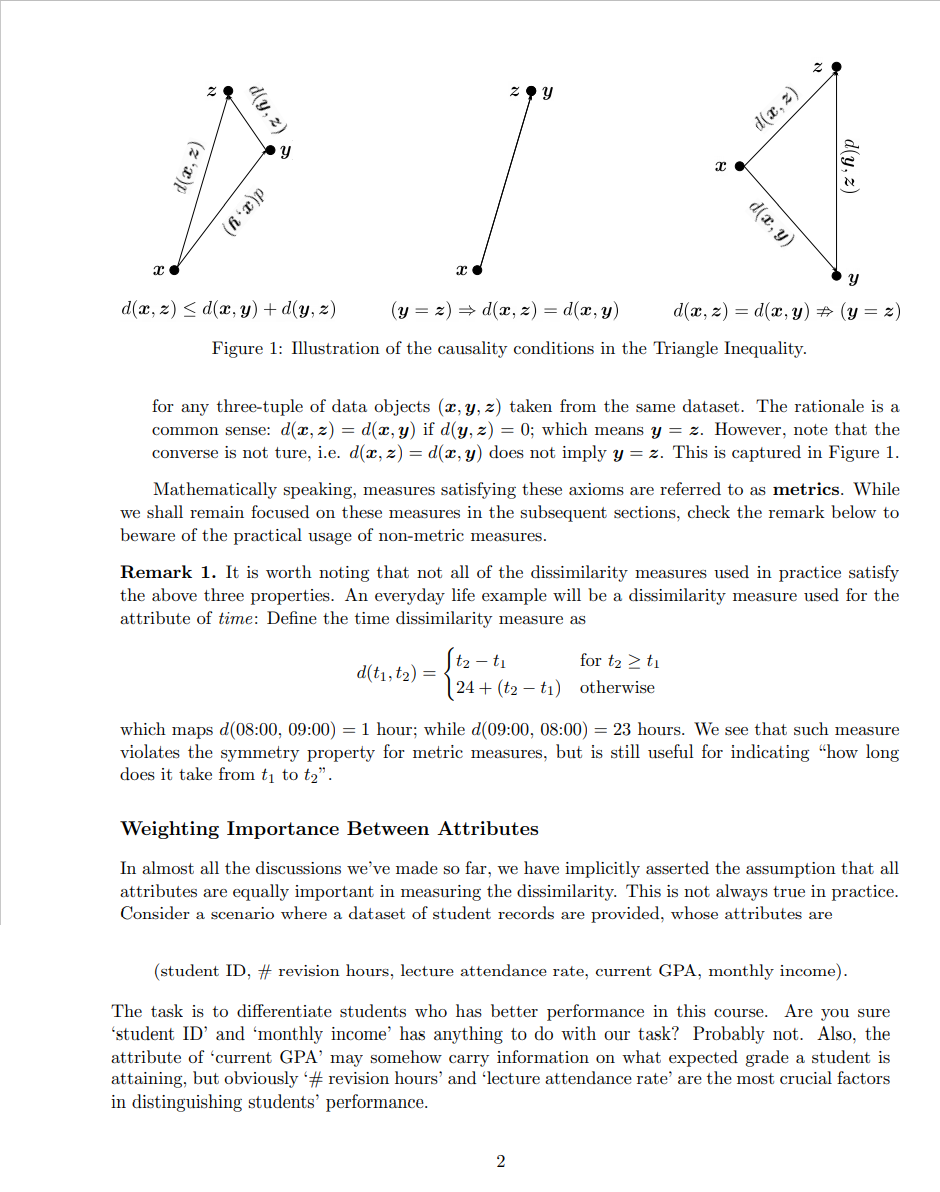
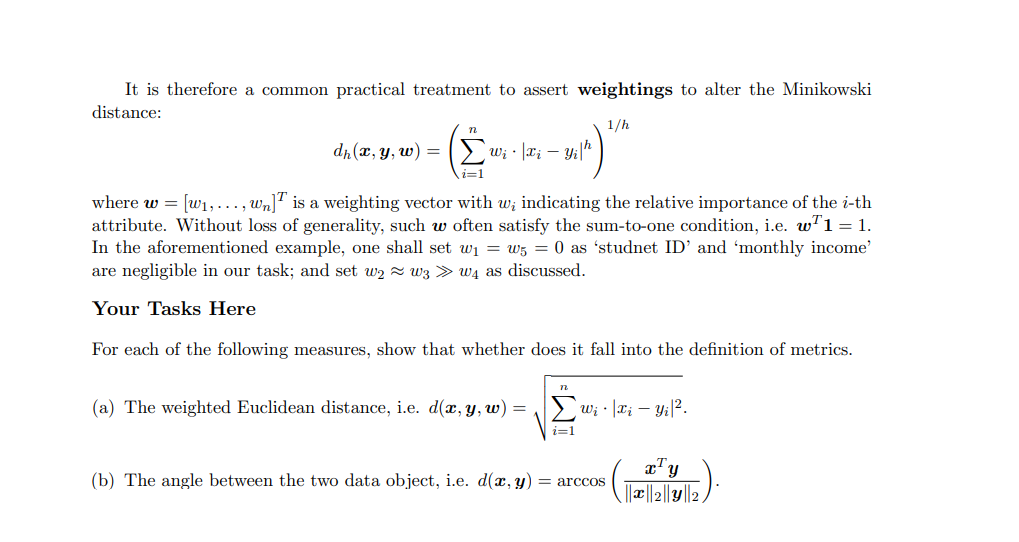
(zip d(z, z) T dy, z) diz,y) (hop C y d(x,z)
t d(t1, t2) = | 24+ (t2 t) otherwise which maps d(08:00, 09:00) = 1 hour; while d(09:00, 08:00) = 23 hours. We see that such measure violates the symmetry property for metric measures, but is still useful for indicating "how long does it take from ti to t2". Weighting Importance Between Attributes In almost all the discussions we've made so far, we have implicitly asserted the assumption that all attributes are equally important in measuring the dissimilarity. This is not always true in practice. Consider a scenario where a dataset of student records are provided, whose attributes are (student ID, # revision hours, lecture attendance rate, current GPA, monthly income). The task is to differentiate students who has better performance in this course. Are you sure 'student ID' and 'monthly income' has anything to do with our task? Probably not. Also, the attribute of current GPA' may somehow carry information on what expected grade a student is attaining, but obviously '# revision hours' and 'lecture attendance rate' are the most crucial factors in distinguishing students' performance. It is therefore a common practical treatment to assert weightings to alter the Minikowski distance: 1/h dn(, y, w) = W; Ii yilh i=1 where w = [w1, ..., Wn] is a weighting vector with w; indicating the relative importance of the i-th attribute. Without loss of generality, such w often satisfy the sum-to-one condition, i.e. WT1=1. In the aforementioned example, one shall set w = w5 = 0 as 'studnet ID' and 'monthly income are negligible in our task; and set w2w3 >> W4 as discussed. Your Tasks Here For each of the following measures, show that whether does it fall into the definition of metrics. 72 (a) The weighted Euclidean distance, i.e. d(x, y, w) = w; |!; yil2 i=1 (b) The angle between the two data object, i.e. d(x, y) = arccOS aty ||2||2||y|2 (zip d(z, z) T dy, z) diz,y) (hop C y d(x,z) t d(t1, t2) = | 24+ (t2 t) otherwise which maps d(08:00, 09:00) = 1 hour; while d(09:00, 08:00) = 23 hours. We see that such measure violates the symmetry property for metric measures, but is still useful for indicating "how long does it take from ti to t2". Weighting Importance Between Attributes In almost all the discussions we've made so far, we have implicitly asserted the assumption that all attributes are equally important in measuring the dissimilarity. This is not always true in practice. Consider a scenario where a dataset of student records are provided, whose attributes are (student ID, # revision hours, lecture attendance rate, current GPA, monthly income). The task is to differentiate students who has better performance in this course. Are you sure 'student ID' and 'monthly income' has anything to do with our task? Probably not. Also, the attribute of current GPA' may somehow carry information on what expected grade a student is attaining, but obviously '# revision hours' and 'lecture attendance rate' are the most crucial factors in distinguishing students' performance. It is therefore a common practical treatment to assert weightings to alter the Minikowski distance: 1/h dn(, y, w) = W; Ii yilh i=1 where w = [w1, ..., Wn] is a weighting vector with w; indicating the relative importance of the i-th attribute. Without loss of generality, such w often satisfy the sum-to-one condition, i.e. WT1=1. In the aforementioned example, one shall set w = w5 = 0 as 'studnet ID' and 'monthly income are negligible in our task; and set w2w3 >> W4 as discussed. Your Tasks Here For each of the following measures, show that whether does it fall into the definition of metrics. 72 (a) The weighted Euclidean distance, i.e. d(x, y, w) = w; |!; yil2 i=1 (b) The angle between the two data object, i.e. d(x, y) = arccOS aty ||2||2||y|2