Go back
Data Driven Clinical Decision Making Using Deep Learning In Imaging(1st Edition)
Authors:
M F Mridha ,Nilanjan Dey

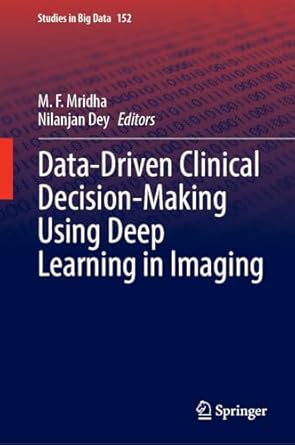
Cover Type:Hardcover
Condition:Used
In Stock
Shipment time
Expected shipping within 2 DaysPopular items with books
Access to 10 Million+ solutions
Free ✝
Ask 10 Questions from expert
200,000+ Expert answers
✝ 7 days-trial
Total Price:
$0
List Price: $199.99
Savings: $199.99(100%)
Book details
ISBN: 9819739659, 978-9819739653
Book publisher: Springer
Get your hands on the best-selling book Data Driven Clinical Decision Making Using Deep Learning In Imaging 1st Edition for free. Feed your curiosity and let your imagination soar with the best stories coming out to you without hefty price tags. Browse SolutionInn to discover a treasure trove of fiction and non-fiction books where every page leads the reader to an undiscovered world. Start your literary adventure right away and also enjoy free shipping of these complimentary books to your door.