Go back
Simulation Based Optimization Parametric Optimization Techniques And Reinforcement Learning(2nd Edition)
Authors:
Abhijit Gosavi

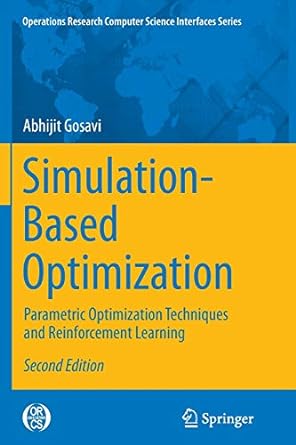
Cover Type:Hardcover
Condition:Used
In Stock
Shipment time
Expected shipping within 2 DaysPopular items with books
Access to 30 Million+ solutions
Free ✝
Ask 50 Questions from expert
AI-Powered Answers
✝ 7 days-trial
Total Price:
$0
List Price: $66.37
Savings: $66.37(100%)
Solution Manual Includes
Access to 30 Million+ solutions
Ask 50 Questions from expert
AI-Powered Answers
24/7 Tutor Help
Detailed solutions for Simulation Based Optimization Parametric Optimization Techniques And Reinforcement Learning
Price:
$9.99
/month
Book details
ISBN: 1489977317, 978-1489977311
Book publisher: Springer
Get your hands on the best-selling book Simulation Based Optimization Parametric Optimization Techniques And Reinforcement Learning 2nd Edition for free. Feed your curiosity and let your imagination soar with the best stories coming out to you without hefty price tags. Browse SolutionInn to discover a treasure trove of fiction and non-fiction books where every page leads the reader to an undiscovered world. Start your literary adventure right away and also enjoy free shipping of these complimentary books to your door.
Book Summary: Simulation-Based Optimization: Parametric Optimization Techniques and Reinforcement Learning introduce the evolving area of static and dynamic simulation-based optimization. Covered in detail are model-free optimization techniques – especially designed for those discrete-event, stochastic systems which can be simulated but whose analytical models are difficult to find in closed mathematical forms.Key features of this revised and improved Second Edition include:· Extensive coverage, via step-by-step recipes, of powerful new algorithms for static simulation optimization, including simultaneous perturbation, backtracking adaptive search and nested partitions, in addition to traditional methods, such as response surfaces, Nelder-Mead search and meta-heuristics (simulated annealing, tabu search, and genetic algorithms)· Detailed coverage of the Bellman equation framework for Markov Decision Processes (MDPs), along with dynamic programming (value and policy iteration) for discounted, average, and total reward performance metrics· An in-depth consideration of dynamic simulation optimization via temporal differences and Reinforcement Learning: Q-Learning, SARSA, and R-SMART algorithms, and policy search, via API, Q-P-Learning, actor-critics, and learning automata· A special examination of neural-network-based function approximation for Reinforcement Learning, semi-Markov decision processes (SMDPs), finite-horizon problems, two time scales, case studies for industrial tasks, computer codes (placed online) and convergence proofs, via Banach fixed point theory and Ordinary Differential EquationsThemed around three areas in separate sets of chapters – Static Simulation Optimization, Reinforcement Learning and Convergence Analysis– this book is written for researchers and students in the fields of engineering (industrial, systems, electrical and computer), operations research, computer science and applied mathematics.
Customers also bought these books
Frequently Bought Together
Top Reviews for Books
Si yu
( 5 )
"Delivery was considerably fast, and the book I received was in a good condition."