Go back
Unsupervised Feature Extraction Applied To Bioinformatics A Pca Based And Td Based Approach(2nd Edition)
Authors:
Y H Taguchi

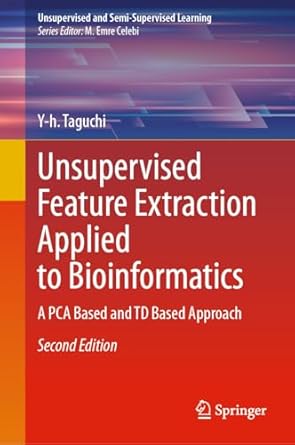
Cover Type:Hardcover
Condition:Used
In Stock
Shipment time
Expected shipping within 2 DaysPopular items with books
Access to 30 Million+ solutions
Free ✝
Ask 50 Questions from expert
AI-Powered Answers
✝ 7 days-trial
Total Price:
$0
List Price: $203.99
Savings: $203.99(100%)
Solution Manual Includes
Access to 30 Million+ solutions
Ask 50 Questions from expert
AI-Powered Answers
24/7 Tutor Help
Detailed solutions for Unsupervised Feature Extraction Applied To Bioinformatics A Pca Based And Td Based Approach
Price:
$9.99
/month
Book details
ISBN: 3031609816, 978-3031609817
Book publisher: Springer
Get your hands on the best-selling book Unsupervised Feature Extraction Applied To Bioinformatics A Pca Based And Td Based Approach 2nd Edition for free. Feed your curiosity and let your imagination soar with the best stories coming out to you without hefty price tags. Browse SolutionInn to discover a treasure trove of fiction and non-fiction books where every page leads the reader to an undiscovered world. Start your literary adventure right away and also enjoy free shipping of these complimentary books to your door.