Question
1 41 Female 5993 8 Yes 6 Yes 2 49 Male 5130 1 No 10 No 3 37 Male 2090 6 Yes 0 Yes 4
1 | 41 | Female | 5993 | 8 | Yes | 6 | Yes |
2 | 49 | Male | 5130 | 1 | No | 10 | No |
3 | 37 | Male | 2090 | 6 | Yes | 0 | Yes |
4 | 32 | Male | 3068 | 0 | No | 7 | No |
5 | 30 | Male | 2693 | 1 | No | 1 | No |
6 | 36 | Male | 5237 | 6 | No | 7 | No |
7 | 29 | Female | 4193 | 0 | Yes | 9 | No |
8 | 31 | Male | 2911 | 1 | No | 5 | No |
9 | 34 | Male | 2661 | 0 | No | 2 | No |
10 | 28 | Male | 2028 | 5 | Yes | 4 | Yes |
11 | 32 | Male | 3298 | 0 | Yes | 6 | No |
12 | 53 | Female | 15427 | 2 | No | 25 | No |
13 | 38 | Male | 3944 | 5 | Yes | 3 | No |
14 | 36 | Male | 3407 | 7 | No | 5 | Yes |
15 | 34 | Female | 11994 | 0 | No | 12 | No |
16 | 21 | Male | 1232 | 1 | No | 0 | No |
17 | 34 | Male | 2960 | 2 | No | 4 | Yes |
18 | 44 | Female | 10248 | 3 | No | 22 | No |
19 | 46 | Female | 18947 | 3 | No | 2 | No |
20 | 44 | Male | 6465 | 2 | Yes | 4 | No |
21 | 30 | Male | 2206 | 1 | No | 10 | No |
22 | 39 | Male | 2086 | 3 | No | 1 | Yes |
23 | 24 | Male | 2293 | 2 | Yes | 2 | Yes |
24 | 43 | Female | 2645 | 1 | No | 5 | No |
25 | 50 | Male | 2683 | 1 | Yes | 3 | Yes |
26 | 35 | Female | 2014 | 1 | No | 2 | No |
27 | 27 | Female | 2341 | 1 | No | 1 | No |
28 | 30 | Female | 4011 | 1 | No | 12 | No |
29 | 41 | Female | 19545 | 1 | No | 22 | Yes |
30 | 37 | Male | 3022 | 4 | No | 1 | No |
31 | 35 | Male | 2269 | 1 | No | 1 | No |
32 | 48 | Male | 5381 | 9 | Yes | 1 | Yes |
33 | 28 | Male | 3441 | 1 | Yes | 2 | Yes |
34 | 44 | Female | 5454 | 5 | Yes | 4 | No |
35 | 35 | Male | 9884 | 2 | Yes | 4 | No |
36 | 33 | Female | 13458 | 1 | Yes | 15 | No |
37 | 35 | Female | 4014 | 3 | Yes | 2 | No |
38 | 31 | Male | 5915 | 3 | No | 7 | No |
39 | 32 | Male | 6162 | 1 | Yes | 9 | No |
40 | 38 | Female | 2406 | 1 | No | 10 | No |
41 | 50 | Female | 18740 | 5 | Yes | 27 | No |
42 | 55 | Female | 14756 | 2 | Yes | 5 | No |
43 | 36 | Male | 6499 | 1 | No | 6 | No |
44 | 36 | Male | 3388 | 0 | Yes | 1 | Yes |
45 | 59 | Female | 5473 | 7 | No | 4 | No |
46 | 29 | Male | 2703 | 0 | No | 5 | No |
47 | 31 | Male | 2501 | 1 | No | 1 | No |
48 | 32 | Male | 6220 | 1 | No | 10 | No |
49 | 36 | Female | 3038 | 3 | No | 1 | No |
50 | 35 | Male | 4312 | 0 | No | 15 | No |
51 | 45 | Male | 13245 | 4 | Yes | 0 | No |
52 | 46 | Male | 5021 | 8 | Yes | 4 | No |
53 | 30 | Male | 5126 | 1 | Yes | 10 | No |
54 | 38 | Female | 5329 | 7 | Yes | 13 | No |
55 | 56 | Male | 7260 | 4 | No | 6 | No |
56 | 23 | Male | 2322 | 3 | No | 0 | No |
57 | 51 | Male | 2075 | 3 | No | 4 | No |
58 | 30 | Male | 4152 | 1 | No | 11 | No |
59 | 46 | Male | 9619 | 1 | No | 9 | Yes |
60 | 40 | Male | 13503 | 1 | No | 22 | No |
61 | 51 | Male | 5441 | 0 | Yes | 10 | No |
62 | 30 | Female | 5209 | 1 | Yes | 11 | No |
63 | 46 | Male | 10673 | 2 | Yes | 10 | No |
64 | 32 | Male | 5010 | 1 | No | 11 | No |
65 | 54 | Female | 13549 | 9 | No | 4 | No |
66 | 24 | Female | 4999 | 0 | No | 3 | No |
67 | 58 | Male | 13872 | 0 | No | 37 | No |
68 | 44 | Male | 2042 | 4 | No | 3 | No |
69 | 32 | Male | 2956 | 1 | No | 1 | No |
70 | 20 | Female | 2926 | 1 | Yes | 1 | Yes |
71 | 34 | Female | 4809 | 1 | No | 16 | No |
72 | 37 | Male | 5163 | 5 | No | 1 | No |
73 | 59 | Female | 18844 | 9 | No | 3 | No |
74 | 50 | Female | 18172 | 3 | Yes | 8 | No |
75 | 25 | Male | 2889 | 1 | No | 2 | No |
76 | 51 | Female | 7484 | 3 | No | 13 | No |
77 | 24 | Male | 2774 | 0 | No | 5 | No |
78 | 34 | Female | 4505 | 6 | No | 1 | No |
79 | 34 | Female | 11631 | 2 | No | 11 | No |
80 | 36 | Female | 2835 | 5 | No | 1 | No |
81 | 30 | Male | 2613 | 1 | No | 10 | No |
82 | 33 | Male | 6146 | 0 | No | 7 | No |
83 | 56 | Female | 4963 | 9 | Yes | 5 | Yes |
84 | 51 | Male | 19537 | 7 | No | 20 | No |
85 | 31 | Male | 6172 | 4 | Yes | 7 | Yes |
86 | 26 | Female | 2368 | 1 | No | 5 | No |
87 | 58 | Female | 10312 | 1 | No | 40 | Yes |
88 | 19 | Male | 1675 | 1 | Yes | 0 | Yes |
89 | 22 | Male | 2523 | 0 | No | 2 | No |
90 | 49 | Female | 6567 | 1 | No | 15 | No |
91 | 43 | Female | 4739 | 4 | No | 3 | No |
92 | 26 | Female | 2942 | 1 | No | 8 | No |
93 | 36 | Male | 4941 | 6 | No | 3 | No |
94 | 51 | Male | 10650 | 2 | No | 4 | Yes |
95 | 39 | Female | 5902 | 4 | No | 15 | No |
96 | 25 | Male | 8639 | 2 | No | 2 | No |
97 | 30 | Male | 6347 | 0 | Yes | 11 | No |
98 | 32 | Female | 4200 | 7 | No | 5 | Yes |
99 | 45 | Male | 3452 | 5 | No | 6 | No |
100 | 30 | Female | 2632 | 1 | No | 5 | No |
101 | 32 | Male | 4668 | 0 | No | 8 | No |
102 | 30 | Female | 3204 | 5 | No | 3 | No |
103 | 30 | Male | 2720 | 0 | No | 5 | No |
104 | 41 | Male | 17181 | 4 | No | 7 | No |
105 | 41 | Male | 2238 | 2 | No | 5 | No |
106 | 40 | Female | 5605 | 1 | No | 20 | No |
107 | 35 | Male | 7295 | 1 | No | 10 | No |
108 | 53 | Male | 2306 | 2 | Yes | 7 | No |
109 | 45 | Male | 2348 | 8 | No | 17 | No |
110 | 32 | Female | 8998 | 1 | No | 9 | No |
111 | 29 | Male | 4319 | 1 | No | 10 | No |
112 | 58 | Female | 3346 | 4 | Yes | 1 | No |
113 | 40 | Male | 10855 | 7 | No | 12 | No |
114 | 34 | Female | 2231 | 6 | No | 4 | No |
115 | 22 | Male | 2323 | 1 | No | 2 | No |
116 | 27 | Male | 2024 | 6 | No | 2 | No |
117 | 28 | Male | 2713 | 1 | No | 5 | No |
Showing 1 to 13 of 1,098 entries, 7 total columns Introduction Angelica is a research statistician working for a management consulting firm in Salt Lake City. Over the years, the firm has developed a strong reputation in human resource consulting. Recently, a large medical device manufacturer, BioImplants, Inc. (or BI), has approached the company for advice on preventing employee turnover. Angelica, with quite a bit of HR consulting experience, was assigned to the project.
BI is a multinational corporation with over $1 billion in annual sales. While employee attrition is a problem in US businesses generally, it is particularly pronounced in growing and dynamic industries like healthcare. It is expensive for companies to rehire a position, costing by some estimates about 21% of the annual salary of the original position.
Angelica interviewed the Director of HR at BI, Stan Devolta, who indicated that the company has been successful in large part due to the work of the HR department and the company's commitment to its employees. It is widely recognized as a desirable place to work. However, Stan admitted that some departments within the company may be better than others at retaining employees, and that employee satisfaction is not universally high. BI would like some insight into employee turnover, and, specifically, answers to questions such as these:
- Who is leaving? - Are there changes that the company could make to retain employees?
Mr. Devolta emphasized that his ultimate goal is to get recommendations for how BI can improve attrition, along with forecasts of improvement and cost savings after implementing the recommendations. He provided Angelica with a sample of the company's HR data.
There is some time pressure. The CEO of BI wants a specific plan for employee retention within a month. Mr. Devolta has therefore stipulated a tight schedule for the analytics project: two weeks. He wants to have time to evaluate the results, revise the analytics if necessary, and fine tune the messaging.
The analytics problem for this project is supervised classification, with a target representing observed employee attrition. The company appears to have sufficient historical data to address the business problem (it has invested heavily in its data infrastructure in the last several years). However, there is missing data. Thus, one of the assumptions for the project is that the existing data contains sufficient information to model the outcome. Another assumption, of course, is that the basic conditions leading to attrition don't change in the near futurethat historical data will be representative of future data.
Based on the conversation with Mr. Devolta, Angelica suspects that the amount of overtime could be related to turnover (as suggested by the HR literature). But she makes no assumptions and wants the data to inform her of the underlying relationships.
```{r} library(tidyverse) library(caret) bi <- read_csv("bioimplants_clean.csv") bi <- bi %>% mutate(gender = as.factor(gender), over_time = as.factor(over_time), attrition = as.factor(attrition))
bi$X1 <- NULL ```
## Questions
## Q1 the average employee attrition rate at BI ```{r} attrition_rate <- mean(bi$attrition == "Yes") attrition_rate table(data$attrition) %>% prop.table() ```
## Q2 Linear probability model.
### Part a
linear probability model for attrition at the company. ```{r}
lm_model <- lm(ifelse(bi$attrition == "Yes", 1, 0) ~ ., data = bi) summary(lm_model) ```
##the effect of `num_companies_worked` on an employee's probability of leaving the company? >
### Part c. Are higher-tenured employees more likely to leave the company?
### Part d. The company is considering assigning overtime to one of its employees (employee details below). How likely will this employee leave the company with and without overtime? (With help of `coef(lm_model)%>% round(5)` command below for the values of the estimates rounded to 5th decimal.) - age: 34 years - gender: Male - monthly_income: $4,000 - num_companies_worked: 2 - years_at_company: 2 ```{r} coef(lm_model)%>% round(5)
```
Step by Step Solution
There are 3 Steps involved in it
Step: 1
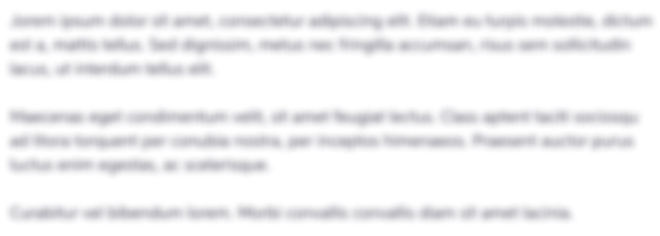
Get Instant Access to Expert-Tailored Solutions
See step-by-step solutions with expert insights and AI powered tools for academic success
Step: 2

Step: 3

Ace Your Homework with AI
Get the answers you need in no time with our AI-driven, step-by-step assistance
Get Started