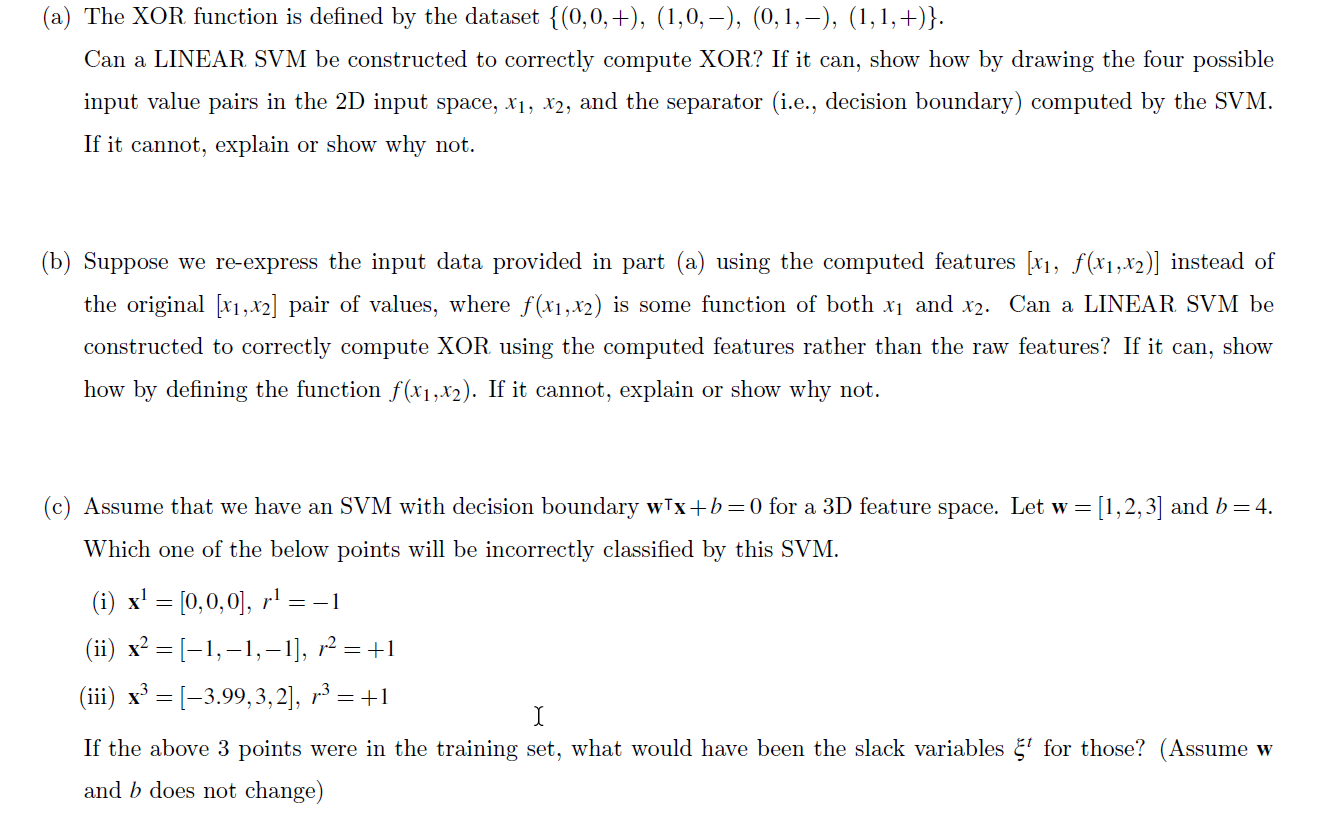
(a) The XOR function is defined by the dataset {(0,0,+), (1,0,), (0,1,), (1,1,+)}. Can a LINEAR SVM be constructed to correctly compute XOR? If it can, show how by drawing the four possible input value pairs in the 2D input space, X1, X2, and the separator (i.e., decision boundary) computed by the SVM. If it cannot, explain or show why not. (b) Suppose we re-express the input data provided in part (a) using the computed features (x1, f(x1,x2)] instead of the original (x1, x2] pair of values, where f(x1,x2) is some function of both x and x2. Can a LINEAR SVM be constructed to correctly compute XOR using the computed features rather than the raw features? If it can, show how by defining the function f(x1,x2). If it cannot, explain or show why not. (c) Assume that we have an SVM with decision boundary wx+b=0 for a 3D feature space. Let w = [1,2,3] and b=4. Which one of the below points will be incorrectly classified by this SVM. (i) x1 = [0,0,0], r1 = -1 (ii) x2 = (-1,-1,-1], r2 = +1 (iii) x3 = 13.99,3,2], r3 = +1 I If the above 3 points were in the training set, what would have been the slack variables & for those? (Assume w and b does not change) (a) The XOR function is defined by the dataset {(0,0,+), (1,0,), (0,1,), (1,1,+)}. Can a LINEAR SVM be constructed to correctly compute XOR? If it can, show how by drawing the four possible input value pairs in the 2D input space, X1, X2, and the separator (i.e., decision boundary) computed by the SVM. If it cannot, explain or show why not. (b) Suppose we re-express the input data provided in part (a) using the computed features (x1, f(x1,x2)] instead of the original (x1, x2] pair of values, where f(x1,x2) is some function of both x and x2. Can a LINEAR SVM be constructed to correctly compute XOR using the computed features rather than the raw features? If it can, show how by defining the function f(x1,x2). If it cannot, explain or show why not. (c) Assume that we have an SVM with decision boundary wx+b=0 for a 3D feature space. Let w = [1,2,3] and b=4. Which one of the below points will be incorrectly classified by this SVM. (i) x1 = [0,0,0], r1 = -1 (ii) x2 = (-1,-1,-1], r2 = +1 (iii) x3 = 13.99,3,2], r3 = +1 I If the above 3 points were in the training set, what would have been the slack variables & for those? (Assume w and b does not change)