Question
Bike Sharing Systems Bike sharing systems are a new generation of bike rentals where the whole process from membership, rental and return has become automatic.
Bike Sharing Systems Bike sharing systems are a new generation of bike rentals where the whole process from membership, rental and return has become automatic. Through these systems, a user is able to easily rent a bike from a particular position and return the bike at another posi- tion. Currently, there are over 500 bike-sharing programs around the world, with some of the best and largest found in Hangzhou (China), Paris (France), London (England), New York City (US) and Montreal (Canada). Great interest in these systems exists due to their role in addressing trac congestion, environmental impact and population health issues in big cities. The data for this assignment comes from one such program, called Capital Bikeshare, operating inWashington in the US. It has over 3000 bicycles that can be rented from over 350 stations across Washington, D.C., Arlington and Alexandria, VA and Montgomery County, MD. Their website encourages users to check out bikes for a trip to work, to run errands, go shopping, or visit friends and family. Users can join Capital Bikeshare for one to three days (casual membership), or for a month or a year (registered membership). Access to the Capital Bikeshare eet of bikes is available 24 hours a day, 365 days a year. The rst 30 minutes of each trip are free. You will use data derived from Capital Bikeshare trip records to build a statistical model for the purposes of predicting the total number of rentals per day. References and Data Sources: Bache, K. & Lichman, M. (2013). UCI Machine Learning Repository http://archive.ics.uci.edu/ml. Irvine, CA: University of California, School of In-formation and Computer Science. Fanaee-T, Hadi, and Gama, Joao, 'Event labeling combining ensemble detectors and background knowledge', Progress in Articial Intelligence (2013): pp. 1-15, Springer Berlin Heidelberg. http://capitalbikeshare.com/system-data
The data file is daily.sas7bdat and contains daily counts of bike rentals for 2011 and 2012, derived from Capital Bikeshare trip history data, with additional weather and seasonal information. The data was downloaded from the UCI Machine Learning Repository. Variables in that le are: Variable Description instant Record index dteday Date season winter, spring summer, autumn (northern hemisphere) yr 0=2011, 1=2012 month Month (January to December) weekday Day of the week (Monday to Sunday) workingday Working day=1, weekend and public holiday = 0 temp Normalised temperature in degrees Celsius; observed temperature di- vided by 41 (max) atemp Normalised `feels like' temperature in degrees Celsius; values divided by 50 (max) hum Normalised humidity; observed values divided by 100 (max) windspeed Normalised wind speed; observed values divided by 67 (max) casual Count of casual users registered Count of registered users count Total count of bike rentals (casual and registered).
Question:
(a)Obtain a Pearson correlation matrix relating variables registered, atemp, temp, hum and windspeed. Also obtain a scatterplot matrix of the same variables. Discuss the relationships. (b)In this question, we investigate observations where workingday=1. Fit a simple regression model relating registered on working days to atemp, with registered as the dependent variable. Discuss the fitted relationship and the goodness of t. Examine residual plots and influence diagnostics and comment on the residual patterns. (c)In this question, we investigate observations where workingday=1. Extend your multiple regression model for registered on working day by including the numerical and categorical predictors. In building your model consider as many potential explanatory variables as possible (you may need to define additional dummy variables). You can use stepwise selection to help you find the most parsimonious (simplest) model with the highest R-square. Be sure to check for collinearity and keep in mind that neither casual nor count should be used as explanatory variables for the total number of users. Summarise how your final model was obtained, including rationale for any modelling decisions you have made, and indicate why that nal was considered the `best'. Report and interpret your final model in detail, including a discussion of model diagnostics. Are there any observations that may require further inspection due to their influence on the model? (d)In this question, we investigate observations where workingday=0. Build a multiple regression model for registered on non-working day, similar to question (c).
Step by Step Solution
There are 3 Steps involved in it
Step: 1
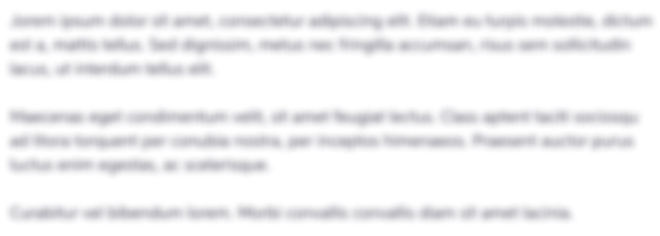
Get Instant Access with AI-Powered Solutions
See step-by-step solutions with expert insights and AI powered tools for academic success
Step: 2

Step: 3

Ace Your Homework with AI
Get the answers you need in no time with our AI-driven, step-by-step assistance
Get Started