Answered step by step
Verified Expert Solution
Question
1 Approved Answer
Coding Style: define a function for each question. please use python - 3 And plase make sure the code compiles this is my third time
Coding Style: define a function for each question. please use python
And plase make sure the code compiles this is my third time posting this question because the answer given do not compile. Use the latest version of all the libraries please and python
Given the Bayesian Networks Inference example discussed in the class, answer the following questions.
The class example includes four random variables: Rain, Maintenance, Train and Appointment. Their dependency relations are: Maintenance depends on Rain, Train depends on Rain and Maintenance, Appointment depends on Train. Construct the Bayesian network and visualize itTip: you can use pgmpy pomegranate or any popular Python library to construct the Bayesian network, and use matplotlib or other library to visualize the network structure
Based on the constructed Bayesian network, make the following inferences: P Rainheavy,
Maintenanceyes PAppointmentattend Rainnone, Maintenanceno
Suppose the probability of attending or missing todays Appointment is not dependent on Train, but
only dependent on yesterdays Appointment. Given the initial state as the probability
distribution of attend and miss and the transition matrix P what is the
probability distribution of attend and miss ten days later? Tip: Simulate the Markov chain over n
steps
Conditional Probability Tables used for and :
PRain
"none":
"light":
"heavy":
PMaintenance Rain
none "yes",
noneno
light "yes",
lightno
heavy "yes",
heavyno
PTrain Rain, Maintenance
none "yes", on time",
none "yes", "delayed",
nonenoon time",
noneno "delayed",
light "yes", on time",
light "yes", "delayed",
lightnoon time",
lightno "delayed",
heavy "yes", on time",
heavy "yes", "delayed",
heavynoon time",
heavyno "delayed",
PAppointment
on time", "attend",
on time", "miss",
delayed "attend",
delayed "miss",
the transition matrix used for :
P $
It means Pattend attend Pattend miss Pmiss
attend Pmiss miss
Step by Step Solution
There are 3 Steps involved in it
Step: 1
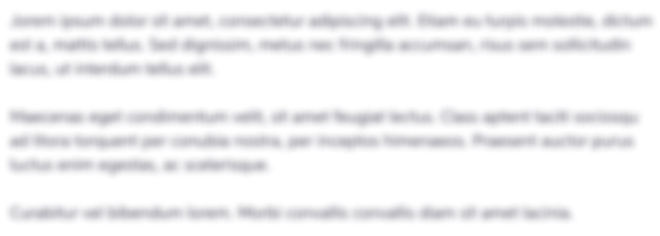
Get Instant Access to Expert-Tailored Solutions
See step-by-step solutions with expert insights and AI powered tools for academic success
Step: 2

Step: 3

Ace Your Homework with AI
Get the answers you need in no time with our AI-driven, step-by-step assistance
Get Started