Answered step by step
Verified Expert Solution
Question
1 Approved Answer
Cross - Validation: Use cross - validation ( like k - fold cross - validation ) to ensure your model generalizes well to unseen data.
CrossValidation: Use crossvalidation like kfold crossvalidation to ensure your model generalizes well to unseen data. Regularization: L and L Regularization: Add regularization terms to the loss function to penalize large weights. L regularization encourages sparsity, while L regularization discourages large weights. Dropout: Randomly drop neurons during training to prevent the model from becoming too reliant on specific neurons. Early Stopping: Monitor the models performance on a validation set and stop training when performance on the validation set starts to degrade, indicating potential overfitting. Data Augmentation: Apply transformations to the training data to generate more diverse examples, helping the model generalize better. Reduce
Step by Step Solution
There are 3 Steps involved in it
Step: 1
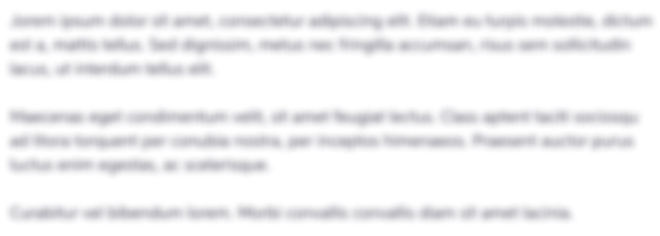
Get Instant Access to Expert-Tailored Solutions
See step-by-step solutions with expert insights and AI powered tools for academic success
Step: 2

Step: 3

Ace Your Homework with AI
Get the answers you need in no time with our AI-driven, step-by-step assistance
Get Started