Do the problem set without limit of page or word count. 1. Millennium Villages and Causal Effects. In the early 2000s, the Millennium Villages project
Do the problem set without limit of page or word count.
1. Millennium Villages and Causal Effects. In the early 2000s, the "Millennium Villages" project was a high-profile anti-poverty program run in villages in Sub-Saharan Africa and de- signed by economist Jeffrey Sachs. The MVP aims to tackle all the root causes of poverty at once by implementing multi-faceted assistance: medical centers, education initiatives, sup- port for improved agricultural productivity, etc. Its motto is "In order to make lasting change in any one sphere of development, we must improve them all." More information on the project can be found here: https://www.millenniumpromise.org/millenniumvillages. In 2012, the project team released an evaluation, published in The Lancet: http://www. sciencedirect.com/science/article/pii/S0140673612602074. This evaluation showed large impacts on a variety of health and poverty indicators in the MVP villages. This evalu- ation was subsequently criticized, however.
The criticism is clearly described in the following article from the Center for Global Devel- opment: https://www.cgdev.org/sites/default/files/Clemens-Demombynes-new-transparenc 1.pdf.1
This question will explore that more thoroughly as a way of getting you to think more deeply about the causal inference methods we discussed in the context of this controversy. The Center for Global Development article, especially the first two sections, is the most useful background reading for this problem.
(a) As described above, the MVP implements a lot of different interventions at the same time. Describe one advantage and one disadvantage of an evaluation of the package as a whole compared with an evaluation of the individual component interventions.
(b) Putting that critique aside, suppose that we are interested in measuring the effect of be- ing a Millenium Development Village on the incidence of mortality for children under 5 years (child mortality).
Describe the following:
i. The population, program and outcome of interest (yes, it's described above but helpful to lay it out first anyway.)
ii. The relevant counterfactual
(c) Suppose a researcher would like to evaluate the MVP. She has heard some fuss about control villages and knows they need to be included in a careful analysis. Specifically, she examines the difference in average mortality between MVP and some non-MVP villages. In regression form, this amounts to the following:
Y =a+bD+ where D takes the value 1 if the village is a MVP village and 0 otherwise.
In the equation above, what letter represents the difference in average mortality between MVP and non-MVP villages?
Explain why this may not represent the true causal effect of MVP support.
Describehowyoumightrevisethemethodologytomoreappropriatelycapturethe causal effect of the program.
(d) Suppose that the researcher instead decides to do a "before-after" comparison of the mortality rate in 2009 in MVP villages with the mortality rate in 2006 in the same villages. In other words, she takes average mortality in 2009 in MVP villages and subtracts average mortality in 2006 in those villages, before they got MVP support.
In this case, what is being used to estimate the counterfactual for MVP villages?
Come up with one reason why this might be a reasonable counterfactual and one reason why it might not be. In your opinion, does this seem like a reasonable strategy to estimate causal effects?
(e) Sachs and his team estimated impacts of the MVP by comparing 9 of the MVP vil- lages to 9 comparison villages. These comparison villages were selected from nearby villages in order to closely "match" the MVP villages on a number of characteristics, and effort was made to ensure that the comparison villages were far enough away from MVP villages that there were no "spillover" effects between them.
Instead of examining the difference in mortality rates between MVP and comparison villages, the Sachs team examined the change in mortality between 2006 and 2009 in MVP villages relative to the same change in control villages.
i. Tounderstandhowthisanalysisworks,wecanre-definetheoutcomeasthechange in mortality betwen 2006 and 2009. That is, we want to know whether the program increased or decreased the rate of change in mortality. Using this outcome, the causal effect of the program on MVP villages is the difference between the change in mortality in these villages minus the counterfactual change had there been no MVP program in these villages.
Describe what is being used to simulate the counterfactual in this case.
Seeifyoucancomeupwithascenarioinwhichthecontrolvillagesdonotprovide a good estimate of the counterfactual.
It turns out that between 2006 and 2009, mortality rates in the control villages rose by 6 deaths per 1000 children under 5. To explore whether this is a "rea- sonable" change in mortality rates, look for a source of data on child mortality in the countries where the MVP villages were located: Nigeria, Ghana, Mali, Sene- gal, Uganda, Kenya, Rwanda, Tanzania, and Malawi. Be sure to document your source. Compare the chages in mortality in these countries with the change in the control villages. Does this comparison suggest that Sachs' estimates could be biased up, down, or neither? Explain.
Why might it be problematic to use only 9 control villages, even if the treatment had been randomly assigned?
2. The Miracle of Microfinance? As we will examine during the Microfinance section, rig- orous evidence on the impacts of microcredit programs was scarce in the 1990s and early 2000s, even though the sector was booming. Starting around 2010, several randomized trials of microcredit were undertaken to fill this evidence gap. This question walks you through some of the analysis in "The Miracle of Microfinance? Evidence from a Randomized Eval- uation" by Banerjee, Duflo, Glennerster, and Kinnan (2015, Hereafter BDGK). The paper is available at https://pubs.aeaweb.org/doi/pdfplus/10.1257/app.20130533. Read the first 11 pages (through the experimental design section). You can skim the rest; we will go over the results in more detail in class.
The study took place in 104 neighborhoods in the Indian city of Hyderabad. The researchers randomly selected half for the intervention. In those neighborhoods, non-governmental mi- crofinance organization (MFI) Spandana began operations. The remaining neighborhoods served as a control group with no intervention.
(a) BDGK's data are based on a set of surveys of households in the study area. The dataset miraclemfi.xlsx on eLC consists of a few key variables from these surveys. I've also posted a text file miraclemfi_labels.txt which provides a brief description of each vari- able.
Lets first "get our hands dirty" by summarizing a few variables.
i. Several features of the context are important for interpreting this study. First, mi- crofinance could be used either to grow a new business or start a new one. What fraction of households have an existing business?
ii. Other MFI's were operating in the area during the evaluation. What fraction of households were borrowing from other MFI's at endline 1?
iii. What fraction of households were in Spandana treatment neighborhoods?
(b) The basic question of the study is whether microfinance access influenced outcomes of interest.
The authors have a number of interesting survey outcomes; I've included a few of them in the dataset if you're interested. Here we're going to focus on women's empower- ment, as measured in the first endline survey. As is common in the literature, the au- thors define women's empowerment as a woman's role in certain household decisions. A number of survey questions are all aggregated into an index, women_emp_index_1. (See Footnote 27 and the associated discussion on page 49.) the _1 at the end of the variable name signifies that it was measured in the first of the two endline surveys. Suppose there was no experiment, and you examined the relationship between microfi- nance access and empowerment using survey data. Specifically, you compare average empowerment in areas with access to an MFI to empowerment in areas without access to an MFI.
Why might this not have a causal interpretation?
(c) Now let's use the experimental variation. Spandana began microfinance activities in a random set of villages in the data. In the remaining villages, they did not conduct any microfinance activities. Now suppose you compare average empowerment in areas with access to Spandana to empowerment in areas without access to Spandana.
Why does this have a causal interpretation, when the analysis in part (b) above does not?
(d) Now let's look at the data. It's a good idea to first see whether Spandana increased access to credit in treatment villages. Compare average MFI borrowing (any_mfi_1) between treatment and control villages. Use a procedure that produces a standard error. For example, you could regress MFI borrowing on treatment:
any_mfi_1i = a+btreatmenti +i How much did Spandana's entry change MFI borrowing?
(e) Now that we've confirmed that the treatment increased borrowing, we can look at the effect on empowerment. Compare average empowerment in treatment and control villages, again using a method that produces a standard error. The regression you can run is:
women_emp_index_1i = a+btreatmenti +i
Is the result statistically significant? Because it is an index, the magnitude is difficult to interpret, but 0.05-0.1 standard deviations would be economically significant, given that empowerment is not the primary outcome in this case.
Note that we are attempting to replicate Column 7 of Table 7 in the BDGK paper. We are going to get slightly different results because of a weighting procedure used by BGDK and because of a standard-error correction. Excel's regression tool doesn't have enough options to get this exactly right.
(f) (Harder-won'tbegraded)Intheliterature,thereisoftenacorrelationbetweenwomen's empowerment and microfinance borrowing, but using randomized trials the causal ef- fect of borrowing on empowerment is small. What does this tell you about the type of women who choose to borrow from MFI's?
Step by Step Solution
There are 3 Steps involved in it
Step: 1
1 Millennium Villages and Causal Effects a Advantage of evaluating the package as a whole It captures the synergistic effects and interactions between different interventions providing a holistic view ...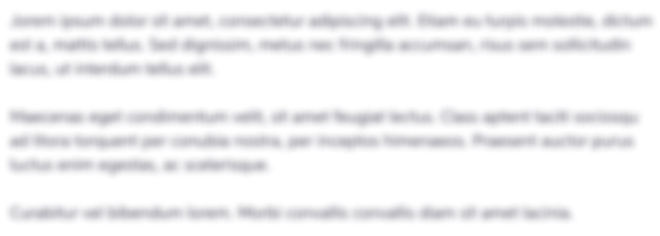
See step-by-step solutions with expert insights and AI powered tools for academic success
Step: 2

Step: 3

Ace Your Homework with AI
Get the answers you need in no time with our AI-driven, step-by-step assistance
Get Started