How do you see the use of Advanced Analytics being applied in the public sector setting? Do you see its use for instance in some of the emerging financial areas such as continuous auditing? Please see reading on advanced analytics below. (Note: Rutgers is a leader in the field of continuous auditing and sponsors an international conference each year on this topic.)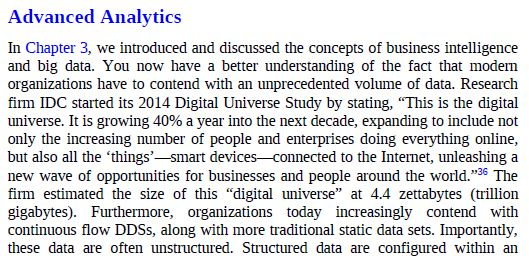
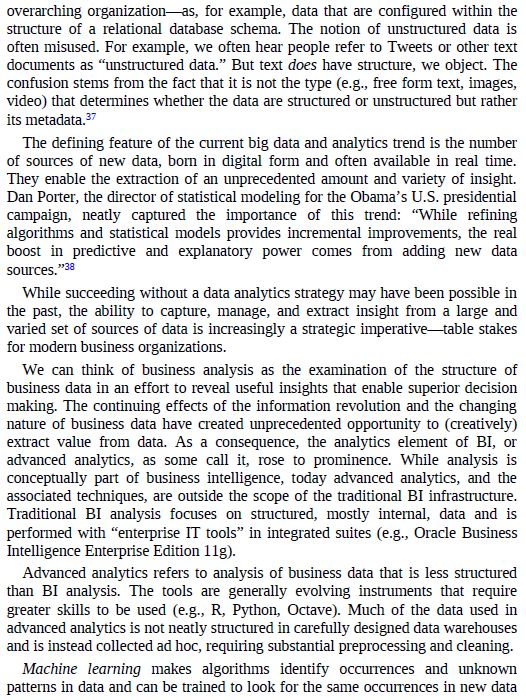
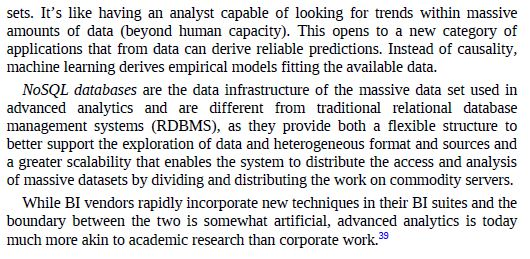
Advanced Analytics In Chapter 3, we introduced and discussed the concepts of business intelligence and big data. You now have a better understanding of the fact that modem organizations have to contend with an unprecedented volume of data. Research fim IDC started its 2014 Digital Universe Study by stating, "This is the diigital universe. It is growing 40% a year into the next decade, expanding to include not only the increasing number of people and enterprises doing everything online, but also all the 'things'-smart devices-connected to the Internet, unleashing a new wave of opportunities for businesses and people around the world."36 The fim estimated the size of this "digital universe" at 4.4 zettabytes (trillion gigabytes). Furthermore, organizations today increasingly contend with continuous flow DDSS, along with more traditional static data sets. Importantly, these data are often unstructured. Structured data are configured within an overarching organization as, for example, data that are configured within the structure of a relational database schema. The notion of unstructured data is often misused. For example, we often hear people refer to Tweets or other text documents as "unstructured data." But text does have structure, we object. The confusion stems from the fact that it is not the type (e.g., free form text, images, video) that deternmines whether the data are structured or unstructured but rather its metadata.37 The defining feature of the current big data and analytics trend is the number of sources of new data, bom in digital form and often available in real time. They enable the extraction of an unprecedented amount and variety of insight Dan Porter, the director of statistical modeling for the Obama's U.S. presidential campaign, neatly captured the importance of this trend: "While refining algorithms and statistical models provides incremental improvements, the real boost in predictive and explanatory power comes from adding new data sources."38 While succeeding without a data analytics strategy may have been possible in the past, the ability to capture, manage, and extract insight from a large and varied set of sources of data is increasingly a strategic imperative table stakes for modern business organizations We can think of business analysis as the examination of the structure of business data in an effort to reveal useful insights that enable superior decision making. The continuing effects of the information revolution and the changing nature of business data have created unprecedented opportunity to (creatively) extract value from data. As a consequence, the analytics element of BI, or advanced analytics, as some call it, rose to prominence. While analysis is conceptually part of business intelligence, today advanced analytics, and the associated techniques, are outside the scope of the traditional BI infrastructure. Traditional BI analysis focuses on structured, mostly internal, data and is performed with "enterprise IT tools" in integrated suites (e.g., Oracle Business Intelligence Enterprise Edition 11g). Advanced analytics refers to analysis of business data that is less structured than BI analysis. The tools are generally evolving instruments that require greater skills to be used (e.g., R, Python, Octave). Much of the data used in advanced analytics is not neatly structured in carefully designed data warehouses and is instead collected ad hoc, requiring substantial preprocessing and cleaning. Machine learning makes algorithms identify occurrences and unknown patterns in data and can be trained to look for the same occurrences in new data sets. It's like having an analyst capable of looking for trends within massive amounts of data (beyond human capacity). This opens to a new category of applications that from data can derive reliable predictions. Instead of causality, machine learning derives empirical models fitting the available data. NOSQL databases are the data infrastructure of the massive data set used in advanced analytics and are different from traditional relational database management systems (RDBMS), as they provide both a flexible structure to better support the exploration of data and heterogeneous format and sources and a greater scalability that enables the system to distribute the access and analysis of massive datasets by dividing and distributing the work on commodity servers. While BI vendors rapidly incorporate new techniques in their BI suites and the boundary between the two is somewhat artificial, advanced analytics is today much more akin to academic research than corporate work.39 Advanced Analytics In Chapter 3, we introduced and discussed the concepts of business intelligence and big data. You now have a better understanding of the fact that modem organizations have to contend with an unprecedented volume of data. Research fim IDC started its 2014 Digital Universe Study by stating, "This is the diigital universe. It is growing 40% a year into the next decade, expanding to include not only the increasing number of people and enterprises doing everything online, but also all the 'things'-smart devices-connected to the Internet, unleashing a new wave of opportunities for businesses and people around the world."36 The fim estimated the size of this "digital universe" at 4.4 zettabytes (trillion gigabytes). Furthermore, organizations today increasingly contend with continuous flow DDSS, along with more traditional static data sets. Importantly, these data are often unstructured. Structured data are configured within an overarching organization as, for example, data that are configured within the structure of a relational database schema. The notion of unstructured data is often misused. For example, we often hear people refer to Tweets or other text documents as "unstructured data." But text does have structure, we object. The confusion stems from the fact that it is not the type (e.g., free form text, images, video) that deternmines whether the data are structured or unstructured but rather its metadata.37 The defining feature of the current big data and analytics trend is the number of sources of new data, bom in digital form and often available in real time. They enable the extraction of an unprecedented amount and variety of insight Dan Porter, the director of statistical modeling for the Obama's U.S. presidential campaign, neatly captured the importance of this trend: "While refining algorithms and statistical models provides incremental improvements, the real boost in predictive and explanatory power comes from adding new data sources."38 While succeeding without a data analytics strategy may have been possible in the past, the ability to capture, manage, and extract insight from a large and varied set of sources of data is increasingly a strategic imperative table stakes for modern business organizations We can think of business analysis as the examination of the structure of business data in an effort to reveal useful insights that enable superior decision making. The continuing effects of the information revolution and the changing nature of business data have created unprecedented opportunity to (creatively) extract value from data. As a consequence, the analytics element of BI, or advanced analytics, as some call it, rose to prominence. While analysis is conceptually part of business intelligence, today advanced analytics, and the associated techniques, are outside the scope of the traditional BI infrastructure. Traditional BI analysis focuses on structured, mostly internal, data and is performed with "enterprise IT tools" in integrated suites (e.g., Oracle Business Intelligence Enterprise Edition 11g). Advanced analytics refers to analysis of business data that is less structured than BI analysis. The tools are generally evolving instruments that require greater skills to be used (e.g., R, Python, Octave). Much of the data used in advanced analytics is not neatly structured in carefully designed data warehouses and is instead collected ad hoc, requiring substantial preprocessing and cleaning. Machine learning makes algorithms identify occurrences and unknown patterns in data and can be trained to look for the same occurrences in new data sets. It's like having an analyst capable of looking for trends within massive amounts of data (beyond human capacity). This opens to a new category of applications that from data can derive reliable predictions. Instead of causality, machine learning derives empirical models fitting the available data. NOSQL databases are the data infrastructure of the massive data set used in advanced analytics and are different from traditional relational database management systems (RDBMS), as they provide both a flexible structure to better support the exploration of data and heterogeneous format and sources and a greater scalability that enables the system to distribute the access and analysis of massive datasets by dividing and distributing the work on commodity servers. While BI vendors rapidly incorporate new techniques in their BI suites and the boundary between the two is somewhat artificial, advanced analytics is today much more akin to academic research than corporate work.39