Question
In this problem, you will consider an example resembles medical imaging reconstruction in MRI. We begin with a true image image of dimension 50 50
In this problem, you will consider an example resembles medical imaging reconstruction in MRI. We begin with a true image image of dimension 50 50 (i.e., there are 2500 pixels in total). Data is cs.mat; you can plot it first. This image is truly sparse, in the sense that 2084 of its pixels have a value of 0, while 416 pixels have a value of 1. You can think of this image as a toy version of an MRI image that we are interested in collecting.
Because of the nature of the machine that collects the MRI image, it takes a long time to measure each pixel value individually, but it's faster to measure a linear combination of pixel values. We measure n = 1300 linear combinations, with the weights in the linear combination being random, in fact, independently distributed as N (0, 1). Because the machine is not perfect, we don't get to observe this directly, but we observe a noisy version. These measurements are given by the entries of the vector
y = Ax + n, where y R1300, A R13002500, and n N(0,25 I1300) where In denotes the identity matrix of
size n n. In this homework, you can generate the data y using this model. Now the question is: can we model y as a linear combination of the columns of x to recover some
coefficient vector that is close to the image? Roughly speaking, the answer is yes.
Key points here: although the number of measurements n = 1300 is smaller than the dimension p = 2500, the true image is sparse. Thus we can recover the sparse image using few measurements exploiting its structure. This is the idea behind the field of compressed sensing.
The image recovery can be done using lasso min y Ax2 + x1.
Now use lasso to recover the image and select using 10-fold cross-validation. Plot the cross-validation error curves, and show the recovered image
To compare, also use ridge regression to recover the image:
Select using 10-fold cross-validation. Plot the cross-validation error curves, and show the recovered image. Which approaches give a better recovered image?
Step by Step Solution
There are 3 Steps involved in it
Step: 1
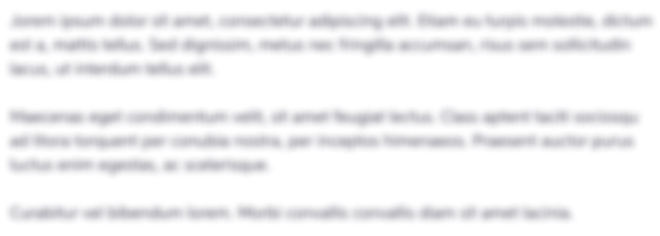
Get Instant Access to Expert-Tailored Solutions
See step-by-step solutions with expert insights and AI powered tools for academic success
Step: 2

Step: 3

Ace Your Homework with AI
Get the answers you need in no time with our AI-driven, step-by-step assistance
Get Started