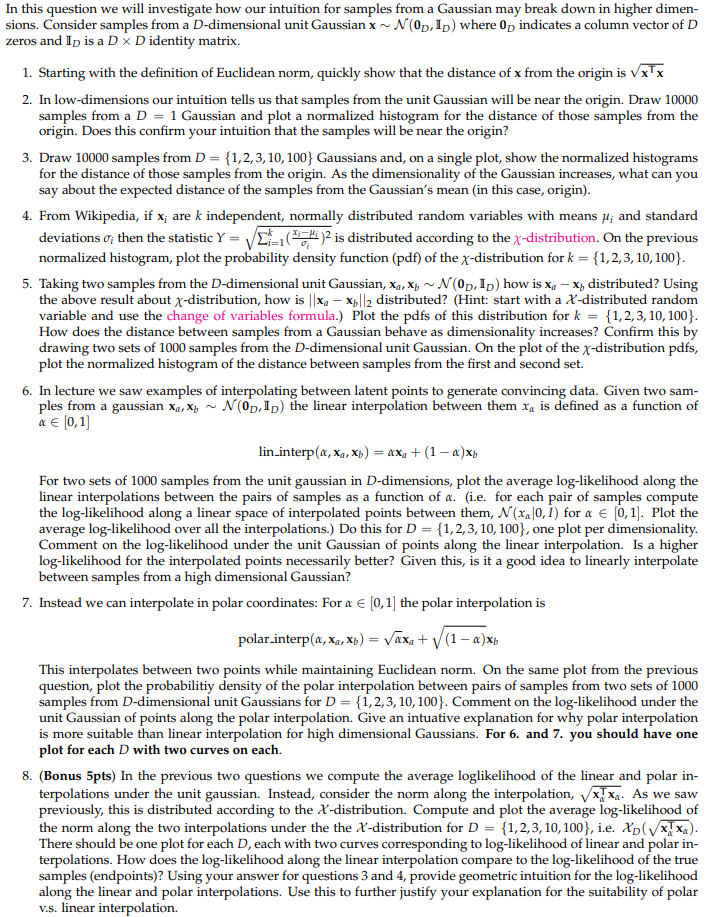
In this question we will investigate how our intuition for samples from a Gaussian may break down in higher dimen- sions. Consider samples from a D-dimensional unit Gaussian x N(0D, ID) where 0p indicates a column vector of D zeros and Io is a Dx D identity matrix. 1. Starting with the definition of Euclidean norm, quickly show that the distance of x from the origin is Vxx 2. In low-dimensions our intuition tells us that samples from the unit Gaussian will be near the origin. Draw 10000 samples from a D-1 Gaussian and plot a normalized histogram for the distance of those samples from the origin. Does this confirm your intuition that the samples will be near the origin? 3. Draw 10000 samples from D-1,2,3, 10, 100 Gaussians and, on a single plot, show the normalized histograms for the distance of those samples from the origin. As the dimensionality of the Gaussian increases, what can you say about the expected distance of the samples from the Gaussian's mean (in this case, origin) 4. From Wikipedia, if xi are k independent, normally distributed random variables with means , and standard deviations i then the statistic Y V kl (rch 2 is distributed according to the X distribution. On the previous normalized histogram, plot the probability density function (pdf) of the x-distribution for k 5. Taking two samples from the 1,2,3, 10, 100) al unit Gaussian, X,N(OD, Ip) how is xa x distributed? Using the above result about -distribution, how is xa-xb 2 distributed? (Hint: start with a X-distributed random variable and use the change of variables formula.) Plot the pdfs of this distribution for k- (1,2,3, 10, 100) How does the distance between samples from a Gaussian behave as dimensionality increases? Confirm this by drawing two sets of 1000 samples from the D-dimensional unit Gaussian. On the plot of the -distribution pdfs, plot the normalized histogram of the distance between samples from the first and second set. 6. In lecture we saw examples of interpolating between latent points to generate convincing data. Given two sam- ples from a gaussian Xa,Xb ~ N(0D,ID) the linear interpolation between them xa is defined as a function of a 0,1 lin-interp(a, xa, Xb)-ax, + (1-a)Xb For two sets of 1000 samples from the unit gaussian in D-dimensions, plot the average log-likelihood along the linear interpolations between the pairs of samples as a function of a. (i.e. for each pair of samples compute the log-likelihood along a linear space of interpolated points between them, N(Xa 0,1) for 0, 1 . Plot the average log-likelihood over all the interpolations.) Do this for D-1,2,3,10, 100, one plot per dimensionality Comment on the log-likelihood under the unit Gaussian of points along the linear interpolation. Is a higher log-likelihood for the interpolated points necessarily better? Given this, is it a good idea to linearly interpolate between samples from a high dimensional Gaussian? 7. Instead we can interpolate in polar coordinates: For e [0, 11 the polar interpolation is polar interp(a, Xa, Xb)-vaxa (1-a)x, This interpolates between two points while maintaining Euclidean norm. On the same plot from the previous question, plot the probabilitiy density of the polar interpolation between pairs of samples from two sets of 1000 samples from D-dimensional unit Gaussians for D 11,2,3, 10, 1003. Comment on the log-likelihood under the unit Gaussian of points along the polar interpolation. Give an intuative explanation for why polar interpolation is more suitable than linear interpolation for high dimensional Gaussians. For 6. and 7. you should have one plot for each D with two curves on each. 8. (Bonus 5pts) In the previous two questions we compute the average loglikelihood of the linear and polar in- terpolations under the unit gaussian. Instead, consider the norm along the interpolation, Vx!xa As we saw previously, this is distributed according to the A-distribution. Compute and plot the average log-likelihood of the norm along the two interpolations under the the X-distribution for D 1,2,3,10, 100), ie. p(Vxa) There should be one plot for each D, each with two curves corresponding to log-likelihood of linear and polar in- terpolations. How does the log-likelihood along the linear interpolation compare to the log-likelihood of the true samples (endpoints)? Using your answer for questions 3 and 4, provide geometric intuition for the log-likelihood along the linear and polar interpolations. Use this to further justify your explanation for the suitability of polar v.s. linear interpolation. In this question we will investigate how our intuition for samples from a Gaussian may break down in higher dimen- sions. Consider samples from a D-dimensional unit Gaussian x N(0D, ID) where 0p indicates a column vector of D zeros and Io is a Dx D identity matrix. 1. Starting with the definition of Euclidean norm, quickly show that the distance of x from the origin is Vxx 2. In low-dimensions our intuition tells us that samples from the unit Gaussian will be near the origin. Draw 10000 samples from a D-1 Gaussian and plot a normalized histogram for the distance of those samples from the origin. Does this confirm your intuition that the samples will be near the origin? 3. Draw 10000 samples from D-1,2,3, 10, 100 Gaussians and, on a single plot, show the normalized histograms for the distance of those samples from the origin. As the dimensionality of the Gaussian increases, what can you say about the expected distance of the samples from the Gaussian's mean (in this case, origin) 4. From Wikipedia, if xi are k independent, normally distributed random variables with means , and standard deviations i then the statistic Y V kl (rch 2 is distributed according to the X distribution. On the previous normalized histogram, plot the probability density function (pdf) of the x-distribution for k 5. Taking two samples from the 1,2,3, 10, 100) al unit Gaussian, X,N(OD, Ip) how is xa x distributed? Using the above result about -distribution, how is xa-xb 2 distributed? (Hint: start with a X-distributed random variable and use the change of variables formula.) Plot the pdfs of this distribution for k- (1,2,3, 10, 100) How does the distance between samples from a Gaussian behave as dimensionality increases? Confirm this by drawing two sets of 1000 samples from the D-dimensional unit Gaussian. On the plot of the -distribution pdfs, plot the normalized histogram of the distance between samples from the first and second set. 6. In lecture we saw examples of interpolating between latent points to generate convincing data. Given two sam- ples from a gaussian Xa,Xb ~ N(0D,ID) the linear interpolation between them xa is defined as a function of a 0,1 lin-interp(a, xa, Xb)-ax, + (1-a)Xb For two sets of 1000 samples from the unit gaussian in D-dimensions, plot the average log-likelihood along the linear interpolations between the pairs of samples as a function of a. (i.e. for each pair of samples compute the log-likelihood along a linear space of interpolated points between them, N(Xa 0,1) for 0, 1 . Plot the average log-likelihood over all the interpolations.) Do this for D-1,2,3,10, 100, one plot per dimensionality Comment on the log-likelihood under the unit Gaussian of points along the linear interpolation. Is a higher log-likelihood for the interpolated points necessarily better? Given this, is it a good idea to linearly interpolate between samples from a high dimensional Gaussian? 7. Instead we can interpolate in polar coordinates: For e [0, 11 the polar interpolation is polar interp(a, Xa, Xb)-vaxa (1-a)x, This interpolates between two points while maintaining Euclidean norm. On the same plot from the previous question, plot the probabilitiy density of the polar interpolation between pairs of samples from two sets of 1000 samples from D-dimensional unit Gaussians for D 11,2,3, 10, 1003. Comment on the log-likelihood under the unit Gaussian of points along the polar interpolation. Give an intuative explanation for why polar interpolation is more suitable than linear interpolation for high dimensional Gaussians. For 6. and 7. you should have one plot for each D with two curves on each. 8. (Bonus 5pts) In the previous two questions we compute the average loglikelihood of the linear and polar in- terpolations under the unit gaussian. Instead, consider the norm along the interpolation, Vx!xa As we saw previously, this is distributed according to the A-distribution. Compute and plot the average log-likelihood of the norm along the two interpolations under the the X-distribution for D 1,2,3,10, 100), ie. p(Vxa) There should be one plot for each D, each with two curves corresponding to log-likelihood of linear and polar in- terpolations. How does the log-likelihood along the linear interpolation compare to the log-likelihood of the true samples (endpoints)? Using your answer for questions 3 and 4, provide geometric intuition for the log-likelihood along the linear and polar interpolations. Use this to further justify your explanation for the suitability of polar v.s. linear interpolation