Answered step by step
Verified Expert Solution
Question
1 Approved Answer
Machine learning approaches to identify Parkinson s disease using voice signal features. Parkinson s Disease ( PD ) is the second most common age -
Machine learning approaches to identify Parkinsons disease using voice signal features.
Parkinsons Disease PD is the second most common agerelated neurological disorder that leads to a range of motor and cognitive symptoms. A PD diagnosis is difficult since its symptoms are quite like those of other disorders, such as normal aging and essential tremor. When people reach visible symptoms such as difficulties walking and communicating begin to emerge. Even though there is no cure for PD certain medications can relieve some of the symptoms. Patients can maintain their lifestyles by controlling the complications caused by the disease. At this point, it is essential to detect this disease and prevent it from progressing. The diagnosis of the disease has been the subject of much research. In this HW your objective is to detect PD using Machine Learning ML model such as KNearest Neighbor KNN to differentiate between healthy and PD patients by voice signal features.
Dataset
The study included voice recordings from people, including people with Parkinsons Disease PD males and females and eight Healthy Controls HCmales and females The dataset contains records, columns, and as presented in Table a series of biomedical voice measurements. Table is divided into columns that represent each of the voice measurements and rows which represent vocal recordings from individuals the name column An average of six recordings were made for each patient; six recordings were taken from patients, and seven recordings were taken from nine patients. The patients ages ranged from to years mean standard deviation and the time since diagnosis ranged from to years. Each row corresponds to one voice recording for s The voice was recorded in an industrial acoustic company soundtreated booth by a microphone placed cm from the mouth. In the dataset, the status column is set to for HC and for those with PD to distinguish healthy individuals from those with PD
Table Matrix column entries attributes:
name ASCII subject name and recording number
MDVP:FoHz Average vocal fundamental frequency
MDVP:FhiHz Maximum vocal fundamental frequency
MDVP:FloHz Minimum vocal fundamental frequency
MDVP:Jitter MDVP:JitterAbs MDVP:RAP, MDVP:PPQ Jitter:DDP Several
measures of variation in fundamental frequency
MDVP:Shimmer, MDVP:ShimmerdB Shimmer:APQ Shimmer:APQ MDVP:APQ, Shimmer:DDA Several measures of variation in amplitude
NHR HNR Two measures of ratio of noise to tonal components in the voice
status Health status of the subject one Parkinson's, zero healthy
RPDE, D Two nonlinear dynamical complexity measures
DFA Signal fractal scaling exponent
spread spread PPE Three nonlinear measures of fundamental frequency variation
Use the Machine Learning algorithm KNearest Neighbor KNN and the DBVoiceFeatures.csv database to discriminate and evaluate classification performance of healthy and PD patients by voice signal features.
Use:dvs python
a Parameter optimization pts
c Discuss the results obtained different metrics
Step by Step Solution
There are 3 Steps involved in it
Step: 1
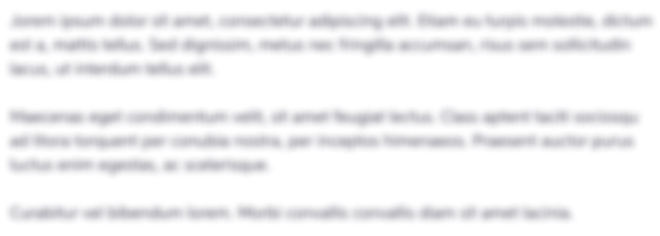
Get Instant Access to Expert-Tailored Solutions
See step-by-step solutions with expert insights and AI powered tools for academic success
Step: 2

Step: 3

Ace Your Homework with AI
Get the answers you need in no time with our AI-driven, step-by-step assistance
Get Started