Question
Modify the perceptron implementation to use the adaline model (using the linear activation function). You should modify the code to use the linear activation function
Modify the perceptron implementation to use the adaline model (using the linear activation function). You should modify the code to use the linear activation function and the update algorithm accordingly. You should train your model using the input data for the OR gate (Note: here you can use the real output 0 or 1 to update the weights, while the predicted output will be a real number. You also need to determine a threshold to convert the output into the final output 0 or 1).
Code: # Code from Chapter 2 of Machine Learning: An Algorithmic Perspective # by Stephen Marsland (http://seat.massey.ac.nz/personal/s.r.marsland/MLBook.html) # You are free to use, change, or redistribute the code in any way you wish for # non-commercial purposes, but please maintain the name of the original author. # This code comes with no warranty of any kind. # Stephen Marsland, 2008 from numpy import * class pcn: #""" A basic Perceptron (the same pcn.py except with the weights printed #and it does not reorder the inputs)"""
def __init__(self,inputs,targets): #""" Constructor """ # Set up network size if ndim(inputs)>1: self.nIn = shape(inputs)[1] else: self.nIn = 1 if ndim(targets)>1: self.nOut = shape(targets)[1] else: self.nOut = 1 self.nData = shape(inputs)[0] # Initialise network self.weights = random.rand(self.nIn+1,self.nOut)*0.1-0.05 def pcntrain(self,inputs,targets,eta,nIterations): #""" Train the thing """ # Add the inputs that match the bias node inputs = concatenate((inputs,-ones((self.nData,1))),axis=1) # Training change = range(self.nData) for n in range(nIterations): self.outputs = self.pcnfwd(inputs); self.weights += eta*dot(transpose(inputs),targets-self.outputs) print ("Iteration: ", n) print (self.weights) activations = self.pcnfwd(inputs) print ("Final outputs are:") print (activations) return self.weights def adltrain(self,inputs,targets,eta,nIterations): #""" Train the thing """ # Add the inputs that match the bias node inputs = concatenate((inputs,-ones((self.nData,1))),axis=1) # Training change = range(self.nData) for n in range(nIterations): self.outputs = self.pcnfwd(inputs); self.weights += eta*dot(transpose(inputs),targets-self.outputs) print ("Iteration: ", n) print (self.weights) activations = self.pcnfwd(inputs) print ("Final outputs are:") print (activations) return self.weights def adlfwd(self, inputs): #run network forward using adaline return def pcnfwd(self,inputs): #""" Run the network forward """ outputs = dot(inputs,self.weights) # Threshold the outputs return where(outputs>0,1,0) def confmat(self,inputs,targets): #"""Confusion matrix""" # Add the inputs that match the bias node inputs = concatenate((inputs,-ones((self.nData,1))),axis=1) outputs = dot(inputs,self.weights) nClasses = shape(targets)[1] if nClasses==1: nClasses = 2 outputs = where(outputs>0,1,0) else: # 1-of-N encoding outputs = argmax(outputs,1) targets = argmax(targets,1) cm = zeros((nClasses,nClasses)) for i in range(nClasses): for j in range(nClasses): cm[i,j] = sum(where(outputs==i,1,0)*where(targets==j,1,0)) print (cm) print (trace(cm)/sum(cm)) import numpy as np inputs = np.array([[0,0],[0,1],[1,0],[1,1]]) targets = np.array([[0],[1],[1],[1]]) p = pcn(inputs, targets) p.pcntrain(inputs, targets, 0.1, 6)
Step by Step Solution
There are 3 Steps involved in it
Step: 1
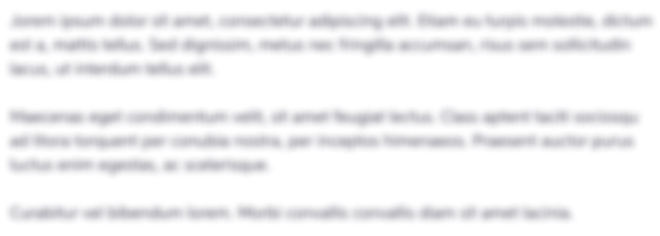
Get Instant Access to Expert-Tailored Solutions
See step-by-step solutions with expert insights and AI powered tools for academic success
Step: 2

Step: 3

Ace Your Homework with AI
Get the answers you need in no time with our AI-driven, step-by-step assistance
Get Started