Answered step by step
Verified Expert Solution
Question
1 Approved Answer
Part II Inference Algorithms and Methods (20 points) The K-means algorithm, also known as the Lloyd-Max algorithm in Information Theory, aims at assigning K
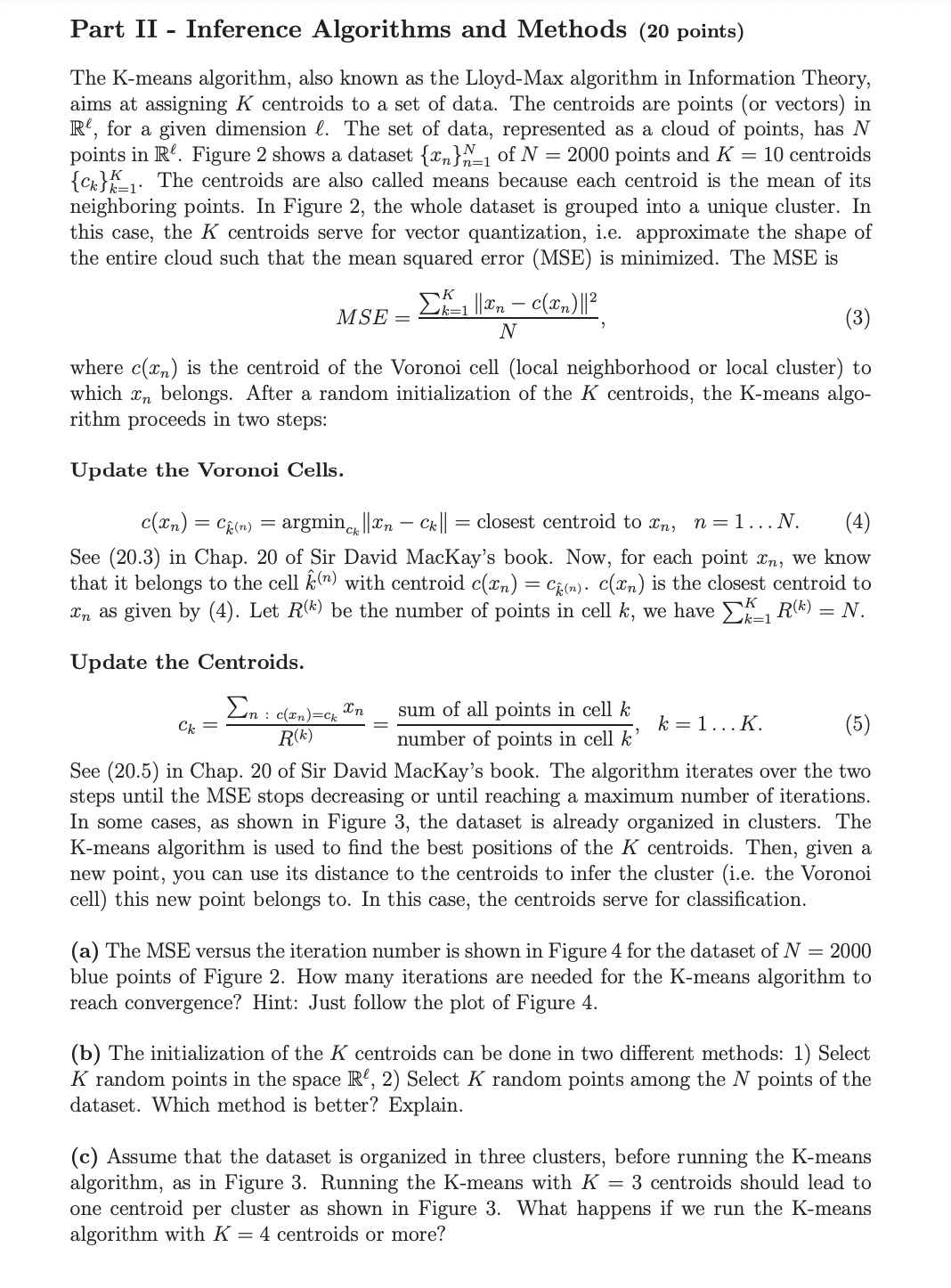
Part II Inference Algorithms and Methods (20 points) The K-means algorithm, also known as the Lloyd-Max algorithm in Information Theory, aims at assigning K centroids to a set of data. The centroids are points (or vectors) in Re, for a given dimension . The set of data, represented as a cloud of points, has N points in R. Figure 2 shows a dataset {n}_1 of N = 2000 points and K = 10 centroids {ck}_ The centroids are also called means because each centroid is the mean of its neighboring points. In Figure 2, the whole dataset is grouped into a unique cluster. In this case, the K centroids serve for vector quantization, i.e. approximate the shape of the entire cloud such that the mean squared error (MSE) is minimized. The MSE is (3) where c() is the centroid of the Voronoi cell (local neighborhood or local cluster) to which I belongs. After a random initialization of the K centroids, the K-means algo- rithm proceeds in two steps: Update the Voronoi Cells. c(xn) = Ck(n) n = 1... N. (4) See (20.3) in Chap. 20 of Sir David MacKay's book. Now, for each point n, we know that it belongs to the cell (n) with centroid c(xn) = C(n). (xn) is the closest centroid to In as given by (4). Let R(k) be the number of points in cell k, we have k_ R(k) = N. k=1 = MSE = Update the Centroids. argminen - Ck || closest centroid to In, - k=1 ||n C(xn)|| N : c(en)=ck R(K) Xn sum of all points in cell k number of points in cell k' Ck = (5) See (20.5) in Chap. 20 of Sir David MacKay's book. The algorithm iterates over the two steps until the MSE stops decreasing or until reaching a maximum number of iterations. In some cases, as shown in Figure 3, the dataset is already organized in clusters. The K-means algorithm is used to find the best positions of the K centroids. Then, given a new point, you can use its distance to the centroids to infer the cluster (i.e. the Voronoi cell) this new point belongs to. In this case, the centroids serve for classification. k = 1... K. (a) The MSE versus the iteration number is shown in Figure 4 for the dataset of N = 2000 blue points of Figure 2. How many iterations are needed for the K-means algorithm to reach convergence? Hint: Just follow the plot of Figure 4. (b) The initialization of the K centroids can be done in two different methods: 1) Select K random points in the space R, 2) Select K random points among the N points of the dataset. Which method is better? Explain. (c) Assume that the dataset is organized in three clusters, before running the K-means algorithm, as in Figure 3. Running the K-means with K = 3 centroids should lead to one centroid per cluster as shown in Figure 3. What happens if we run the K-means algorithm with K = 4 centroids or more?
Step by Step Solution
There are 3 Steps involved in it
Step: 1
a The number of iterations needed for the Kmeans algorithm to reach convergence cannot be determined ...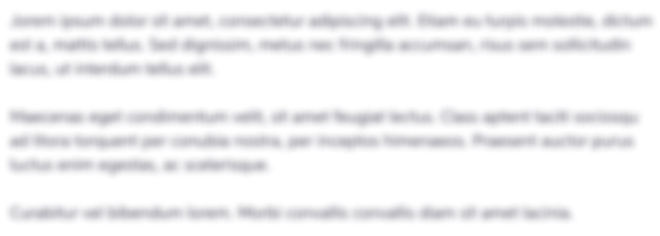
Get Instant Access to Expert-Tailored Solutions
See step-by-step solutions with expert insights and AI powered tools for academic success
Step: 2

Step: 3

Ace Your Homework with AI
Get the answers you need in no time with our AI-driven, step-by-step assistance
Get Started