Answered step by step
Verified Expert Solution
Question
1 Approved Answer
Please provide the answer for part e. 3 Logistic Regression Assume we have two features 2 = (1,22). Each sample is ( 2 1 or
Please provide the answer for part e.
3 Logistic Regression Assume we have two features 2 = (1,22). Each sample is ( 2 1 or 0. 1 ) with associated label y) that is either (a) Decision boundary. (5 pts) Logistic regression assumes log ( 02.03 ) = 0 + 0,0 +0,22. Let's define the prediction threshold as p, such that if Py = 12,0) >p, where 0 log 2 (b) Decision boundary, cont. [5 ptsThe line defined by 6. + 011 + 02.12 = log (2) is called the decision boundary of the logistic regression model, since it splits the plane in two regions of different predictions. What is the decision boundary when we fairly set p = 0.5? Describe, in plain language, what happens to the decision boundary when p is changed. (c) Linear separability problem. [5 pts Suppose we have the following data: ( 11 1 1 (2) 0 0 1 (3) 0 1 0 1 0 0 Table 1: Data Plot this data on a piece of paper, if you will. Is it possible to find a line on the plane to separate the sample points of different classes? (d) Linear separability, cont. (10 pts) Fix the threshold as p=0.5. Prove, in this case, that there is no set of parameters wo, W1, w, that can make correct classifications on all samples. Will I be able to solve the previous problem by altering the threshold? Briefly describe why. (Hint: start with r(2). What must Wo satisfy, if w(2) is classified correctly? Continue with other samples until you obtain a contradiction. ) (e) Basis expansion. [5 pts) Now, the original features, 21, 22, is not enough to make the samples separable by a straight line. In times like these, a linear model on the original features won't work perfectly. Luckily, with a simple trick we can make the problem solvable! We will use a basis function: (11,02), and the logistic regression model becomes log ( 2:0) = wo + w121 + w202 + W30(81,12). Design a basis function (21,22) to make the four samples linear separable and find the weights (wo, W1, W2, W3) that makes the classification work perfectly with threshold 0.5. (Hint: (102) should assume the same value for (1), (2), and another value for (3), (4), Then with only you can make perfect classifications already. 3 Logistic Regression Assume we have two features 2 = (1,22). Each sample is ( 2 1 or 0. 1 ) with associated label y) that is either (a) Decision boundary. (5 pts) Logistic regression assumes log ( 02.03 ) = 0 + 0,0 +0,22. Let's define the prediction threshold as p, such that if Py = 12,0) >p, where 0 log 2 (b) Decision boundary, cont. [5 ptsThe line defined by 6. + 011 + 02.12 = log (2) is called the decision boundary of the logistic regression model, since it splits the plane in two regions of different predictions. What is the decision boundary when we fairly set p = 0.5? Describe, in plain language, what happens to the decision boundary when p is changed. (c) Linear separability problem. [5 pts Suppose we have the following data: ( 11 1 1 (2) 0 0 1 (3) 0 1 0 1 0 0 Table 1: Data Plot this data on a piece of paper, if you will. Is it possible to find a line on the plane to separate the sample points of different classes? (d) Linear separability, cont. (10 pts) Fix the threshold as p=0.5. Prove, in this case, that there is no set of parameters wo, W1, w, that can make correct classifications on all samples. Will I be able to solve the previous problem by altering the threshold? Briefly describe why. (Hint: start with r(2). What must Wo satisfy, if w(2) is classified correctly? Continue with other samples until you obtain a contradiction. ) (e) Basis expansion. [5 pts) Now, the original features, 21, 22, is not enough to make the samples separable by a straight line. In times like these, a linear model on the original features won't work perfectly. Luckily, with a simple trick we can make the problem solvable! We will use a basis function: (11,02), and the logistic regression model becomes log ( 2:0) = wo + w121 + w202 + W30(81,12). Design a basis function (21,22) to make the four samples linear separable and find the weights (wo, W1, W2, W3) that makes the classification work perfectly with threshold 0.5. (Hint: (102) should assume the same value for (1), (2), and another value for (3), (4), Then with only you can make perfect classifications alreadyStep by Step Solution
There are 3 Steps involved in it
Step: 1
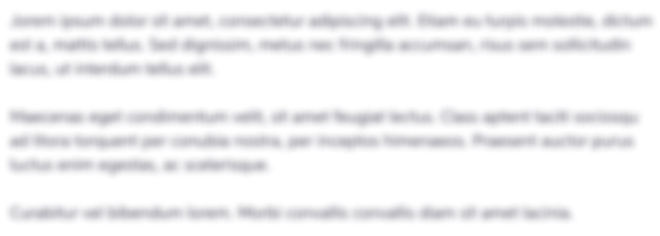
Get Instant Access to Expert-Tailored Solutions
See step-by-step solutions with expert insights and AI powered tools for academic success
Step: 2

Step: 3

Ace Your Homework with AI
Get the answers you need in no time with our AI-driven, step-by-step assistance
Get Started