Question
Please read the case study first and then create report of 1200 words minimum. Thank you You are to assume the role of consultant to
Please read the case study first and then create report of 1200 words minimum. Thank you
You are to assume the role of consultant to the business in the case. The document you prepare will be a report that is submitted to the management team. You will analyze the organizations internal and external environment using situation analysis and based on that analysis diagnosis the primary problem the organization is faced with. Then you will offer alternatives that could potentially solve that problem, analyze their costs and benefits, make a recommendation for solving the problem, create an action plan for implementation and indicate how the implemented action plan will be evaluated.
Introduction
Situation Analysis (SWOT)
Problem Diagnosis
Identification of Alternatives
Analysis of Each Alternative
Decision
Action Plan for Implementation
Evaluation
Conclusion
For the exclusive use of N. Ahsan, 2023 IVEy Publishing MCCORMICK \& CO: DEPLOYING ARTIFICIAL INTELLIGENCE IN NEW PRODUCT DEVELOPMENT lekertifying information to pretuct ednfidenthally. maserials of the higheat qualfs aubmit any emata to poblianeasidgmey.ea. Meratat: 20210028 In February 2020, Jim Martin, director of Global Creative Intelligence at McCormick \& Company, Inc. (McCormick), an American multinational flavours and spices company, was examining the way forward with the compamy's product development initiatives imvolving the use of artificial intelligence (AI). Martin was heading a cross-functional core team of twelve members at the company's headquarters in Hunt Valley, Maryland. The team was interfacing with over 500 product developers located at the company's locations worldwide. McCormick had launched three flavours developed with AI in November 2019, and the market response had been favourable. As he pulled into the driveway at McCormick's research centre on a Monday morning. Martin was facing three managerial dilemmas: How should MoCormick get its product developers to begin to trust the findings of Al? How should McCormick teach and train MI to become smarter? Finally, how should McCormick deal with global-local dynamics within the organization when launching Al-engincered products? THE SEASONING, SAUCE, AND CONDIMENT SEGMENT The packaged foods industry, of which the seassoning, sauce, and condiment segment was part, was valued globally at Us52.37 trillion in 2019. With the five largest companies together holding less than 10 per cent of the global market share, the packaged foods industry was fragmented. Private labels held 12 per cent of the market share, and 96 per cent of industry products, such as groceries, was distributed through storebased retailing. 2 The industry was in the middle of seismic changes driven by three factors: demography, distribution, and sustaimability. Millennials, defined as thooe born between 1981 and 19963, were the single largest demographic unit who were also on their way to their prime working and spending years. Their shopping and consumption habits were different from those of their predecessors, the baby boomers. Millennials' 1Alldollaramountsintheeletearein US dollars. BtatulesErutudeninder. 2 Michael Dimock, "Defining Gefwnations Where Milenida End and Qenerebion 2 Begira," Pew Research Center, danuary The seasoning, sauce, and condiment segment had revenues of $21.8 billion in the United States in 2019 . It had been growing at 0.4 per cent per year. Within this segment, the flavours market was valued at 20 per cent of the 521.8 billion revemues." Flavour played a crucial role in the commercial success of a food product. Product developers had access to thousands of ingredients, both locally and globally, to develop flavour formulations. A common ingredient like garlic powder, for example, was available in dozens of variants differing by metrics such as particle size, country of origin, drying process, and flavour profile. Even while the combinations of available ingredients were changing, new ingredients were also being introduced regularly. 7 There had been little innovation for decades in the way product developers worked. Their work was iterative and had not changed even with the deployment of tools such as MI. Flavour formulation was a resourceand time-intensive process. Designing new flavour experiences was more of an art: The art came into play because it took many years for a person to become an expert product developer. The science part was not fully understood, largely because the flawour spoce did not have an established mathematical description." Speed was of the essence in the demanding and competitive emvironment of product development. Being the first to respond with a new and novel product or flavour was a compelling competitive advantage in the industry. A major challenge for product developers was to develop icons that would stand the test of time in an industry where the shelf life of a new product did not exiend beyond five years. Product stickiness was a litmus test for a product developer." Flavour experience was a combination of at least three senses: aroma, taste, and appearance. There was a long-standing view that the nooe, mouth, and brain dominated how people experienced flavour. Scientists were now of the view that the eye also played a powerful role in the experience. There was also evidence that in addition to the four commonly understood states of taste-sour, sweet, salty, and bitter-there was at least one more taste known as umami, and that every taste receptor, wherever it occurred, could detect all five states. A genetic component in flavour experience had also been recognired, which explained why some people experienced cilantro-also known as coriander-as a delightful herb while others experienced it as unpleasant." "plackaged Food." " "Packiged Focd." " IBISWorld, Beosening, Sauet, and Condiment Producton io the US, IBISWund Report 31194 , November 2012. "Hamed Faridi, Richard Qoodwin, Robin Leugee, and James Martin, "Artifial Intelioonse and the Future of Flavor Innovifion," Ceveal Foods Word 64, na. 6 (Nowember-Decomber 2019\%, "Faria, Coodwin, Lougee, and Mertin, "Artfifidil Inkellgenee." "Faria, Coodwin, Leugee, and Martin, "Autficial Intelligenoe." ARTIFICIAL INTELLIGENCE The tern MI meant "the capability of a machine to imitate the natural intelligence of haman behavior, such as visual perception, learning, reasoning, understanding natural langugge, and problem solving. wil Al could also be described as "the development of computer programs'systems that are able to perform tasks that usually require human intelligence." AI was a framework of different technologies brought together to enable a machine to act with levels of intelligence comparable to the human mind by mimicking it in four ways: sensing, comprehending, acting. and learning. It allowed the machine to perceive the world around it by processing images, sounds, speech, text, and data, emabling the machine to recognire patterns in the information it collected and to take actions in the physical or digital world based on that comprehension. AI also enabled a machine to optimize its performance by contimuously leaming from the successes or failures of those actions." .3 Economists considered AI a general-purpose technology along the lines of electricity or the internal combustion engine, the impact of which went beyond their immediate relevance toward unforeseen applications. They believed that AI was thus well positioned, for example, to scale up the disruption being caused by digitalization. 14 In the summer of 1956, after the advent of modern computing, the term "AI" was coined and the field of AI was established as an academic discipline in the United States. The proliferation of interest in AI was due to the confluence of three trends: the availability of massive amounts of data, increased computing power as seen with the arrival of graphical processing units, and advancements in algonithms. 19 AI was being applied to many fields, ranging from health care, agriculture, and education to aviation, finance, and market analysis. It was already introducing major changes in the packaged foods industry, especially in product development. The net worth of Al was predicted to reach $54 billion by 202616 In a 2019 cross-industry survey, 47 per cent of business executives reported that their companies had embedded at least one AI capability in their business processes, while 30 per cent reported that they were piloting Al initiatives. Individual companies were facing five major challenges in coping with Al. The first pertained to integrating Al strategy into the company's overall business strategy. The second was about prioritiving revenue growth over cost reduction in deploying Al. The third was about aligning the production of AI with its consumption. The fourth pertained to unifying AI initiatives with the larger business transfomation efforts of the company. Finally, the fifth challenge was around making appropriate investments in AI around relevant talent, data, and processes - in addition to the core technology. 17 Each company's experience in meeting those challenges was unique to its business, systems, and culture. creative." 15 Academic research continued to expand the definition of what it meant to be creative. The goal of computational creativity was to replicate creativity by using a computer to achieve one of three ends: (1) constructing a program capable of human-level creativity, (2) formulating an algorithmic perspective on creative behaviour in humans, or (3) designing programs that could enhance haman ereativity without humans themselves being creative. IBM was among the companies that believed human-level creativity was possible for computers. It was applying computational creativity to the development of new culinary recipes using a four-step process. First, it downloaded related descriptions of different cuisines from various sources in the public domain and process them by deploying big data. Second, it used a "novelty algorithm" to combine ingredients that would not be nomally combined based on factors such as flavour pleasentness. Third, a human (such as a product developer) chose an ingredient as a base for the computational machine to come up with a recipe. Finally, a human chose one of the constructed recipes and made it. 30 There was a set of criteria that researchers in computational creativity used to ascertain whether a compuser was indeed creative. One of them was fluency, which referred, in general, to the number of new ideas generated. With culinary creativity, fluency was about the number of recipes the computer came up with. Related criteria were: How original were the ideas? How good, useful, and relevant were they? Relevance pertained to market relevance. Creativity was also measured by the alternative complements the computer suggested in the event of non-availability of raw materials, and its ability to "adjust" formulas based on intended user reactions such as "pleasantness" or "gender appropriateness." Computational creativity did not suggest the "final" recipe but only a recipe that would have a "high probability of success " Its goal was not to spit out a new gravy formula, for instance, but to help product developers come up with a new gravy formula faster. It often gave information that tumed out to be incorrect. However, it gave enough good pointers that people had no hesitation in returning to it. 2a MACHINE LEARNING Machine learning was another subset of Al. Simply stated, it trained a machine to leam. It taught a machine to perform specific tasks by relying on pattems, independently and without instructions. Virtual personal assistants, such as Alexa and Siri, were examples of machine leaming applications. More common industrial applications included predicting traffic flows, detecting crimes before they happened through video surveillance predictions, recognizing and identifying faces, filtering malware, and providing online customer support. Machine learning was also being used by financial services companies to prevent fraud, by manufacturing companies to manage cobot-driven assembly lines, by health care companies to detect chronic diseases, and by agriculture companies to determine sowing dates. 12 In the food and beverage industries, the AI system used a suite of machine learning algorithms to generate and recommend suggestions for formulas and recipes. The algorithms leamed five different attributes: 23 1. Applicationc This came into play in learning whether a formula was, for example, a barbecue sauce, dry seasoning, or rice dish. If the application was a dry seasoning, then the system would know that it should not generate suggestions incorporating liquids. 2. Functional substitutes: The algorithms would leam about ingredients that could be substituted for each other, such as lime and lemon. 3. Complements: Similarly, the algorithms would leam about ingredients that could be used together, such as basil and oregano. 4. Distance: The algonithms would also learn the notion of distance between two formulas. The closer the distance, the more similar the formulas. 5. Success: The algorithm became familiar with success factors (such as taste tests, elient wins, and sales) to predict which formulas were more likely to have greater success. MCCORMICK: COMPANY BACKGROUND Set up in 1889 in a basement in Baltimore, Maryland, by Willoughby M. McCormick to sell flavours door to door, McComick \& Company was a 130-year-old global leader in flawour. By 2019, the compamy had 12,400 full-time cmployees worldwide. It had revenues of $5.3 billion and a gross profit of $2.1 billion (see Exhibit 1). Over time, the compamy had grown both organically and through acquisitions, with the latter generating one-third of sales. McCormick had two business segments, the consumer segment and the flavour solutions segment, which together manufactured and marketed about 17,000 stock kecping units. The consumer segment had brands with a retail portfolio that included spices, herbs, seasonings, desserts, recipe mixes, extracts, condiments, and marinades. The flavour solutions segment portfolio consisted of flavourings, branded food services products, condiments, and coating systems and ingredients. The consumer segment contributed about 60 per cent of sales and 70 per cent of operating income, while the flavour solutions segment contributed 40 per cent of sales and 30 per cent of operating income. The company was also a leading supplier of private labels, also known as siore brands, in both segments. It had thus covered all price points from premium to popular categories. McCormick was a global enterprise with a total of twenty-five state-of-the-art manufacturing facilities, including ten in the United States, three in China; two each in Australia, France, and the United Kingdom; and one each in Canada, India, Italy, Mexico, and Poland. The organirational structure was a combination of products and regions. The company sourced 14,000 raw materials from eighty-five countries. Among the core raw materiak it used were dairy products, pepper, vanilla, garlic, hot and bell peppers, onions, rice, and wheat flour. Pepper and related spices were sourced from Asian countries while dairy products and onions were sourced locally. The company was involved principally in business-to-business (B2B) transactions. Its customers spanned a broad spectrum of retailers including grocery chains; mass merchandisers; warehouse clubs; discount stores; drug stores; and e-commerce retailers, whom it served through wholesalers. Walmart Inc. was the single largest institutional customer of the company's consumer segment, accounting for 11 per cent of McCormick's consolidated sales anmully. Similarly, PepsiCo, Inc. was the single largest customer of the Flavour Solutions segment, accounting for 10 per cent of consolidated sales annually. The top throe flavour solutions customers represented about 45 per cent of the segment's global sales. The company had an ongoing comprehensive continuous improvement plan. This was a four-year cost reduction initiative that was launched in 2016 with a targeted savings goal of $400 million. The plan had generated $463 million in cost savings by November 2019 , exceeding its goal a year ahead of schedule. McCormick founded its research and development department in 1951, making it the first company in the food industry to have done so. The company grew largely through acquisitions and had a track record of timely and successful integrations. Among its high-profile acquisitions was the foods division of British multinational Reckitt Benckiser Group plo, which it acquired in 2017 for about $4.21 billion. The division had attractive positions across the hot sauce, mustard, crispy vegetables, and barbecue sauce categories in the US and Canadian markets. The company considered flavour an "advantaged" global food category. Its research had shown that 79 per cent of US consumers added flavourful ingredients and spices to their cooking, 81 per cent of French consumers were imterested in trying new flavours, 71 per cent of Australian consumers loved trying new spices and seasonings, and 60 per cent of UK consumers were keen on trying new ingredients. With the proliferation of television cooking shows, international travel, and the popularity of ethnic restaurants, consumers were becoming food-savvy. However, the biggest advantage McConmick had was that Generation Z, according to its research, was "hyper focused" on flavour." PRODUCT DEVELOPMENT AT MCCORMICK McCormick had product developers at its twenty labs in fourteen countries across five continents. These developers had varied backgrounds as food scientists, chemists, chemical engineers, nutritionists, and chefs. They would start with a basic recipe, known in the industry as a seed fonmula, and build from it. They relied on a six-step Stage-Gate system, as it was called, to create flavours: ideation, development, testing, scaleup, commercialisation, and maintenance. Much of the effort went imto the first two steps. For example, while working on a new Tuscan chicken recipe, the developers would first search the company"s database for previous Tuscan chicken recipes and use these to "tweak" the flavours. Finding the right recipe meant adding, subtracting, and changing ingredients. The process involved, on average, about 150 iterations to fine-tune the recipe into a market-ready product, and the process duration was anywhere from six to eighteen months. McCormick was known in the industry for its annual Flavor Forecast. At the beginning of each year, the company announced the tastes it believed would define that year. In its Jamaary 2019 forecast, McCormick suggested that basil seeds might be the new "super food." It also predicted that a Japanese sesame blend called gomasio could become the trendy new way to cnhance the taste of pasta or roasted vegetables. McCormick's imvolvement in Al began with a radio program that the company's chief scientific officer. Hamed Fanidi, heard in December 2012. It was an interview with Lay Varshney, a computer scientist at IBM Research, who spoke of how a computer "could be made creative in the kitchen" by giving it access to a hage number of recipes serving as the computer's "inspiration database" and by letting the computer "rearrange the recipes, add new ingredients and generate millions of new ideas for recipes." BBM Research had already stepped into the kitchen a few years prior when it used Watson, its famous supercomputer, to create a recipe app that could spit out new cooking ideas. Watson had come up with workable recipes, such as one that was a hybrid between an Indian curry and a Spanish paella comprising potatoes, pork, beef with some spices and turmeric and mango rum topping. Some suggestions were wild, like flavourful salad combinations, and some were outrageous, like putting mayonnaise in a Bloody Mary. A paper published in 2011 suggested that "there are approximately 10 recipes in use worldwide, compared to the vast theoretical possibility of 1015 recipes. wh There was clearly a gap that MI could bridge, as Fanidi saw at the time. In 2013 , he launched an initiative with IBM Research to identify the potential of AI to create new flavours. McCormick had more than forty years of documented data in its system. The data however, were in silos and legacy systems, lodged in a variety of formats and languages scatterod around the world. They were never shared. The first priority was to reorganize those data into an accessible form, merging data from multiple systems, digitizing data stored on paper, and standardizing data captured across organizational boundaries. The goal was to gain clarity on the relationships among various data sources and their significance. The second was to build the technological infrastructure to manage the data. This was where IBM Research came into play. IBM researchers enlisted product developers at MoCormick for inputs toward building the system. There was also the larger and more critical task of preparing the team of 500 product developers and the organixation in general for the incoming Al tochnology- Building on its recent experiences in using MI to pair flavours as well as its proprietary [BM Research N ] for Product Composition, IBM Research used advanced machine-leaming algorithms to sift through McCormick's data. The Al system it developed for McCormick was meant to identify "possible alternative raw material complements and substitutes for a formula." The way prodact developers worked at MoCormick had not changed in decades, it was labour-intensive. Looking for a formula that met all the requirements was often akin to searching for a needle in a haystack. Product developers clearly needed tools to make them more efficient and effective. With the new partnership with IBM, the deployment of big data and digitization of the flavour space seemed to be the way forwand. In February 2019, McCormick and IBM Research signed a formal collaboration pioneering the use of A] in developing flavours. MoCormick's vision for bringing Al to its organization was threefold: elevating the capability and impact of every product developer to the level of the best team member, accelerating the rate of innovation by at least three times, and significantly enhancing new-product staying power in the market. THE ISSUES BEFORE MARTIN McCormick used the AI system to launch three products in November 2019 under its spice brand, Recipe Mix. The flavours it had developed for the products included Tuscan chicken, bourbon pork tenderloin, and New Orleans sausage. While the products were showing early signs of acceptance in the marketplace, Martin was dealing with some core dilemmas at his desk. Getting Product Developers to Trust the Findings of MI There were two mutually reinforcing elements in addressing this first issue: trust and engagement. It was important to get the product developers to engage with Al as part of getting them to trust it. This was where generating some wins not necessanily quick wins with Al seemed necessary. Demonstrable evidence of success with AI would make it easier to get the developers to engage with AI. For example, the team would have a mandate to develop a new gravy line and three developers would be asked to come up with a brown gravy, turkey gravy, and chicken gravy. If only two of them used AI and succeeded, the thind was bound to conclude that they also better use Al so as not to be seen as failing. Bringing them around was not easy, nor was it simply a matter of time before the product developers could engage with Al. There were five areas of contradiction in the attributes of MI and individual product developers that were causing a trust deficit among the product developers. First, consumer preference for a flavour was the result of one's emotional, physical, and spiritual experiences, some of which went back to early childhood. Creating a flavour experience involved the comvergence of three senses - taste, smell, and sight - in all of their historical sweep. AI could not taste or smell the way humans could. Acconding to McCormick, Al could not fommalate a rocipe for colour because it did not identify colours. Al was colour blind. Second, as they gained more experience and greater confidence, product developers veered towands a suite of personal favoanites of ingredients. Most developers had a go-to solution they lnew and trusted. A product developer thus relied on indivhaul experiential successes or failures to determine the path forward. This contrasied with AI, which relied on the collective experiential suocesses and failures of all developers on a team. Third, as an extension of the above, personal bias invariably came into play with a product developer. Al did not have any of the cultural biases that humans normally had. A classic example was pirna seasoning, for which a product developer would automatically think of ltalian flavours. Al might come up with a fluvour like cumin-likely better and tastier. This would take the product developer outside their confort zone. Fourth, what was known as hedonic motivation came into play with product developers. If one's emotional experience could be thought of as a gauge ranging from bad to good, the primary motivation would be to keep the needle on the gauge as close to good as possible. If a product developer had a hedonic target of between 5 and 7 , for example, they would be inclined to move toward full commercialization by hitting a sensory evaluation of 7 . Al had no such limits. It would always push the boundaries of product development in a way that humans could not, and it would do so tirclessly. Fifth, a product developer over time became an expert in very specific, but very marrow, sets of combinations. In other words, they developed tunnel vision. Given that the potential raw material combinations were too huge for a single individual to even begin to comprehend, a product developer would miss out on a world of opportunities as a matter of coutine. AI had no such problem, nor did it have a problem processing extraordinarily large amounts of data. It could expand a product developer's imagination to try new things they would not otherwise try. Generally, however, product designers found it uncomfortable to get on board with Al. A senior product developer would see it as too intrusive. A junior product developer would take it lightly and end up playing the Al like a video game. How to Teach and Train AI to Become Smarter The need to teach and train Al to become smarter with each passing day was based on the premise that Al should be like a product developer and not the other way around. AI was nothing more than a tool to be used by pooduct developers to improve their individual efficiency and effectiveness. It was helpful that Al was not a deterministic software program and that it was wired intrinsically for continuous learning. It was designed as a learning machine, and every day would be betier than the previous day for AI because it never stopped incorporating feedback from developers into its next suggestion. With more and more data being peled upon it and with rolling foedback, it was only improving itself. Nevertheless, in improving itoelf, Al was also prone to making mistakes as a matter of routine. Consider, for example, someone designing an algonithm to find cats in the wikd and training Al in a relevant algorithm. The training could happen at tao levels: first, by showing stationary pictures of various animals (such as dogs. horses, and racoons in addition to cats) and second, by giving real-world data in terms of videos of live animals and asking it to identify cats. Dogs: check; horses: check; cats: check. Ln processing a racoon, Al might think of it as a cat because of its little black nose and a pair of boown eyes. This was where learning kicked in, and it happened with the feedback. Al moukd continue to learn until it "adjusted" in response to feedback. Feedback was the lifeblood of AL. Slip-aps occurred when the feedback was not continuous. An important element that came into play in this regard was what was known in the industry as drift. When Al and product developers did not communicate and drifted apart, it was difficult to get back on track with the same momentum. Equally important in teaching and training Al was the generation of the most relevant and high. quality data, which would form the basis of the feedback. It was important to be committed to fine-tuning AI, whose "neural networks," as they were called, were always hungry for both data and feedback. Teaching and training Al were too nascent in the flavour segment to arrive at a set of best practices. However, there were learnings from the automotive sector, such as providing insights into the shape of things to come. AI was in extensive use in driverless cars, where the limitations of neural netwodks were becoming evident. Al had trouble handling "edge" cases situations where there was a data vacuum. A driverless car could handle crosswalks, pedestrians, and dense traffic with ease but had trouble processing an anomaly such as children dressed in unusual Halloween costumes weaving across the street after dusk. There were also learnings from social media, such as the iPhone X 's facial recognition system failing to recognize "moming faces" characterized by puffy, haggard looks after waking up from skeep. 29 As a result, when an iconic global product was replicated from one part of the world to another, its profile was localized to incorporate local tastes and supply chain limitations, even as the essence of the product was preserved. A product such as Montreal Steak Seasoning, a signature spice blend developed by McCormick's Canadian operations seventy years ago, could thus be replicated in China by adapting it to local tastes. The compamy's product developers in China did not have to reinvent the wheel. The company had also developed a line of noodles, sauces, and seasonings that it called Simply Asia, which captured the distinct character and flavours of each Asian region. Around 2010, McCormick first developed a vision to create "one global lab" in the company. Its partnership with IBM in 2013 set the stage for this. McCormick was planning to create all-new products by 2022 using the AI platform. By then, the system woald have more than half a million formulations and one billion data points, both internal and external. The company faced two dilemmas in managing global-local dynamics: data consistency and data bias. - Data consisteney: While conducting a "liking test," for example, the point scale had to be uniform among countries. If the test was conducted on a five-point scale in the United States and a seven-point scale in the United Kingdom, the interpretation of soores would be flawed. Similarly, when testing was done while the participants were at a dining table in one country and on a patio in another, the ratings would not be amenable to common interpretation. - Data bins: The data set of McCormick, as a company, was biased towards the United States because McCormick was an American company that had been doing business in the United States for 130 years. An important part of managing the global-local dynamics on a platform like Al was to recognize the bias and find ways to eliminate it. Establishing uniform business processes was the first step toward not only ensuring data consistency but also eliminating data bias. The use of AI at McCormick was promising. However, it demanded a lot from the organization and its people. McCormick's core mission was to excel at the development and delivery of flavours, not AI innovation. Martin wondered how best to proceedStep by Step Solution
There are 3 Steps involved in it
Step: 1
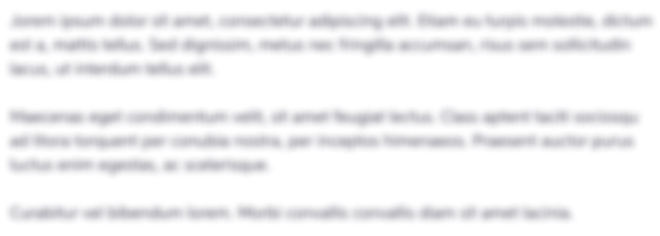
Get Instant Access to Expert-Tailored Solutions
See step-by-step solutions with expert insights and AI powered tools for academic success
Step: 2

Step: 3

Ace Your Homework with AI
Get the answers you need in no time with our AI-driven, step-by-step assistance
Get Started