Question
Please Use R to answer this question: Camer Pharmaceuticals is testing a new product in the market 1 . The demand for the new product
Please Use R to answer this question:
Camer Pharmaceuticals is testing a new product in the market1. The demand for the new product is estimated to be Normally distributed with a mean 2,000,000 and standard deviation 250,000. The demand is estimated to grow at a rate of 4% per year. The R&D costs are estimated to be between $500 millions of dollars and $800 millions of dollars with a most likely value of $700 millions of dollars. Clinical trial costs are estimated to be between $135 millions of dollars and $160 millions of dollars with a most likely value of $150 millions of dollars. There are competitors in the market, and Camer Pharmaceuticals estimates that their market share in the first year will be any number between 6% and 10%, with each number being equally likely. The company estimates that their market share will grow by 20% each year. A monthly prescription is estimated to generate a revenue of $240. The variable costs are estimated to be $30. Develop a simulation model that calculates the net present value (NPV) of this project over 3 years assuming a discount rate of 10%. Run the simulation for 1000 iterations and answer the following questions.
a.What is the distribution of the NPV (mean and standard deviation)?
b.What are the first quartile, median, and third quartile of NPV?
c.What is the probability that NPV over 3 years will not be positive?
d.What NPV are we likely to observe with a probability of at least 0.9?
e.What cumulative net profit in the third year are we likely to observe with a probability of at least 0.9?
f.What is the 95% confidence interval for the mean NPV? Interpret the resulting confidence interval.
g.What is the number of iterations needed if we want to estimate the NPV within $4,000,000?
Interpret the sensitivity chart.
Here is the sample code related to the question
library(tidyverse)
library(extraDistr)
# starting at time 1, describe the npv of cash flows occurring
# once per period
npv<-function(cf,i)
sum(cf*(1+i)^-(1:length(cf)))
#lognormal distribution, parametrized by its own mean and sd
qlnorm2<-function(p,mean,sd)
qlnorm(p=p,
meanlog=log(mean*(1+sd^2/mean^2)^-.5),
sdlog=log(1+sd^2/mean^2)^.5)
rlnorm2<-function(n,mean,sd)
qlnorm2(runif(n),mean,sd)
#certain inputs
options(digits=3)
n<-10000
y<-5
i<-.09
mkt_share=0.08
Unit_Profit<-12*(130-40)
#uncertain inputs
stochastic_model<-
data.frame(mkt_size=rnorm(n,2,.4),
RD=runif(n,600,800),
CT=rlnorm2(n,150,30))
temp<-replicate(n,
expr=data.frame(year=1:y,
size_growth=c(1,rtriang(y-1,1.02,1.06,1.03)),
share_growth=c(1,rtriang(y-1,1.15,1.25,1.2))
),
simplify=F)
#calculate NPV and Cumulative Profit
for(row in 1:n){
a<-temp[[row]] %>%
mutate(Sales=mkt_share*stochastic_model$mkt_size[row]*
cumprod(size_growth*share_growth),
Profit=Sales*Unit_Profit,
Cum_Profit=cumsum(Profit)-
stochastic_model$RD[row]-
stochastic_model$CT[row])
temp[[row]]<-a
}
stochastic_model$year_df<-temp
rm(a)
rm(temp)
stochastic_model$NPV<-
lapply(X=stochastic_model$year_df,
FUN=function(df) df$Profit) %>%
sapply(FUN=npv,i=i)-
stochastic_model$RD-
stochastic_model$CT
flat_df<-stochastic_model %>%unnest(year_df)
#calculate summary statistics for NPV, cum_profit
m<-stochastic_model$NPV %>%mean() #mean NPV
s<-stochastic_model$NPV %>%sd() #sd NPV
m
s
quantile(stochastic_model$NPV,c(.025,.975)) #95% conf int of NPV
qnorm(c(.025,.975))*s/sqrt(n)+m #95% conf int for mean of NPV
mean(stochastic_model$NPV>0) #likelihood that NPV will be positive
mean((flat_df %>% filter(year==3))$Cum_Profit>0) #likelihood of breaking even by y3
#create risk profiles for outputs of interest
ggplot(stochastic_model)+
geom_histogram(aes(x=NPV,y=..count../sum(..count..)),
fill='dark blue',color='blue',
breaks=seq(-700,1000,100))+
ylab("probability")+
geom_vline(xintercept=quantile(stochastic_model$NPV,c(.025,.975)),
linetype='dashed',color='red')+
theme_classic()
ggplot(flat_df)+
geom_density(aes(x=Cum_Profit,
group=year,
fill=as.factor(year)),
alpha=0.5)+
theme_classic()
size_tb<-sapply(stochastic_model$year_df,
function(df) df$size_growth) %>%
t() %>%
as.data.frame() %>%
select(-1)
colnames(size_tb)<-paste('size_growth_y',2:5,sep='')
share_tb<-sapply(stochastic_model$year_df,
function(df) df$share_growth) %>%
t() %>%
as.data.frame() %>%
select(-1)
colnames(share_tb)<-paste('share_growth_y',2:5,sep='')
corr_table<-stochastic_model %>%
select(NPV,mkt_size,RD,CT) %>%
cbind(size_tb,share_tb)
X<-cor(corr_table)[,1][-1]
ggplot()+geom_col(aes(x=reorder(names(X),abs(X)),y=X,fill=X>0),
color='black')+
coord_flip()+
scale_fill_manual(values=c('red','blue'),guide='none')+
ylab('uncertain input')+
xlab('correlation with npv')+
theme_classic()
Step by Step Solution
There are 3 Steps involved in it
Step: 1
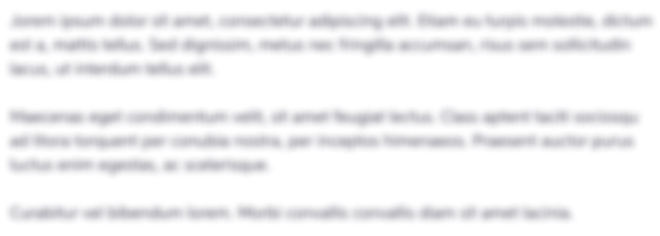
Get Instant Access to Expert-Tailored Solutions
See step-by-step solutions with expert insights and AI powered tools for academic success
Step: 2

Step: 3

Ace Your Homework with AI
Get the answers you need in no time with our AI-driven, step-by-step assistance
Get Started