Answered step by step
Verified Expert Solution
Question
1 Approved Answer
Project 4: The California Bureau of Prison's board of directors wanted to be able to predict parole violations (DV) by prison punishment record (IV) and
Project 4: The California Bureau of Prison's board of directors wanted to be able to predict parole violations (DV) by prison punishment record (IV) and prior criminal record (IV). Information on 4000 inmates of the state penitentiary system from 1989 - 1994 records were scrubbed. The data collected is provided in the accompanying excel table (parole data set.xlsx). Columns are coded as follows. Incidence of Parole Violation, by punishment record and prior criminal record for 4000 inmates of California State penitentiary system from 1989- 1994. Punishment record in prison 0=None, 1=1-2 times, 2=3 or more times Prior record 0=none, 1=fine/probation, 2=reform school, 3=jail, 4=penitentiary Subsequent parole violation 0=No, 1=Yes */ Number of inmates Use the Check list (Table 10.16) found on page 482 of the text book and the information provided below to write a report on the Logistic Regression for the data set provided. (Hint: Report should be similar in scope to \"Results at the end of Chapter 10\". Logistic Regression Output Logistic Regression Case Processing Summary Unweighted Casesa Selected Cases N Included in Analysis Missing Cases Total Unselected Cases Total Percent 4000 100.0 0 .0 4000 100.0 0 .0 4000 100.0 a. If weight is in effect, see classification table for the total number of cases. Dependent Variable Encoding Original Value Internal Value No 0 Yes 1 Categorical Variables Codings Parameter coding Frequency Prior Record Prison Punish Rec. None (1) (2) (3) (4) 2540 1.000 .000 .000 .000 Fine/Probation 356 .000 1.000 .000 .000 Reform School 448 .000 .000 1.000 .000 Jail 440 .000 .000 .000 1.000 Penitentiary 216 .000 .000 .000 .000 2468 1.000 .000 1-2 Times 956 .000 1.000 3 or More Times 576 .000 .000 None Block 0: Beginning Block Classification Tablea,b Predicted Subseq Parole Violations Observed Step 0 No Subseq Parole Violations Percentage Yes Correct No 2924 0 100.0 Yes 1076 0 .0 Overall Percentage 73.1 a. Constant is included in the model. b. The cut value is .500 Variables in the Equation B Step 0 Constant S.E. -1.000 Wald .036 df 786.087 Sig. 1 .000 Exp(B) .368 Variables not in the Equation Score Step 0 Variables df Sig. PPUNREC 72.685 2 .000 PPUNREC(1) 72.259 1 .000 PPUNREC(2) 31.225 1 .000 117.669 4 .000 PRREC(1) 94.508 1 .000 PRREC(2) .001 1 .976 PRREC(3) 51.526 1 .000 PRREC(4) 18.399 1 .000 161.998 6 .000 PRREC Overall Statistics Block 1: Method = Enter Omnibus Tests of Model Coefficients Chi-square Step 1 df Sig. Step 157.367 6 .000 Block 157.367 6 .000 Model 157.367 6 .000 Model Summary Cox & Snell R Step 1 -2 Log likelihood Square 4500.727a Nagelkerke R Square .039 .056 a. Estimation terminated at iteration number 4 because parameter estimates changed by less than .001. Classification Tablea Predicted Subseq Parole Violations Observed Step 1 No Subseq Parole Violations Yes Percentage Correct No 2924 0 100.0 Yes 1076 0 .0 Overall Percentage 73.1 a. The cut value is .500 Variables in the Equation B Step 1a S.E. PPUNREC Wald df Sig. 44.853 2 .000 Exp(B) PPUNREC(1) -.498 .102 23.712 1 .000 .608 PPUNREC(2) -.002 .113 .000 1 .983 .998 87.301 4 .000 PRREC PRREC(1) -.807 .148 29.571 1 .000 .446 PRREC(2) -.575 .184 9.734 1 .002 .563 PRREC(3) .019 .170 .013 1 .911 1.019 PRREC(4) -.209 .172 1.480 1 .224 .811 Constant -.149 .158 .896 1 .344 .861 a. Variable(s) entered on step 1: PPUNREC, PRREC. SEQUENTIAL LOGISTIC REGRESSION OUTPUT Logistic Regression Case Processing Summary Unweighted Casesa Selected Cases N Included in Analysis Missing Cases Total Unselected Cases Total Percent 4000 100.0 0 .0 4000 100.0 0 .0 4000 100.0 a. If weight is in effect, see classification table for the total number of cases. Dependent Variable Encoding Original Value Internal Value No 0 Yes 1 Categorical Variables Codings Parameter coding Frequency Prior Record Prison Punish Rec. None (1) (2) (3) (4) 2540 1.000 .000 .000 .000 Fine/Probation 356 .000 1.000 .000 .000 Reform School 448 .000 .000 1.000 .000 Jail 440 .000 .000 .000 1.000 Penitentiary 216 .000 .000 .000 .000 2468 1.000 .000 1-2 Times 956 .000 1.000 3 or More Times 576 .000 .000 None Block 0: Beginning Block Classification Tablea,b Predicted Subseq Parole Violations Observed Step 0 Subseq Parole Violations Overall Percentage a. Constant is included in the model. b. The cut value is .500 No Percentage Yes Correct No 2924 0 100.0 Yes 1076 0 .0 73.1 Variables in the Equation B Step 0 Constant S.E. -1.000 Wald .036 df 786.087 Sig. 1 Exp(B) .000 .368 Variables not in the Equation Score Step 0 Variables df Sig. PPUNREC 72.685 2 .000 PPUNREC(1) 72.259 1 .000 PPUNREC(2) 31.225 1 .000 72.685 2 .000 Overall Statistics Block 1: Method = Enter Omnibus Tests of Model Coefficients Chi-square Step 1 df Sig. Step 71.516 2 .000 Block 71.516 2 .000 Model 71.516 2 .000 Model Summary Cox & Snell R Step -2 Log likelihood 1 4586.577 Square a Nagelkerke R Square .018 .026 a. Estimation terminated at iteration number 4 because parameter estimates changed by less than .001. Classification Tablea Predicted Subseq Parole Violations Observed Step 1 No Subseq Parole Violations Yes Percentage Correct No 2924 0 100.0 Yes 1076 0 .0 Overall Percentage 73.1 a. The cut value is .500 Variables in the Equation B Step 1 a S.E. PPUNREC Wald df Sig. 71.739 2 .000 Exp(B) PPUNREC(1) -.653 .100 42.921 1 .000 .520 PPUNREC(2) -.067 .111 .370 1 .543 .935 Constant -.601 .087 47.552 1 .000 .548 a. Variable(s) entered on step 1: PPUNREC. Block 2: Method = Enter Omnibus Tests of Model Coefficients Chi-square Step 1 df Sig. Step 85.850 4 .000 Block 85.850 4 .000 Model 157.367 6 .000 Model Summary Cox & Snell R Step 1 -2 Log likelihood Square 4500.727a Nagelkerke R Square .039 .056 a. Estimation terminated at iteration number 4 because parameter estimates changed by less than .001. Classification Tablea Predicted Subseq Parole Violations Observed Step 1 No Subseq Parole Violations Yes Percentage Correct No 2924 0 100.0 Yes 1076 0 .0 Overall Percentage 73.1 a. The cut value is .500 Variables in the Equation B Step 1a S.E. PPUNREC Wald df Sig. 44.853 2 .000 Exp(B) PPUNREC(1) -.498 .102 23.712 1 .000 .608 PPUNREC(2) -.002 .113 .000 1 .983 .998 87.301 4 .000 PRREC PRREC(1) -.807 .148 29.571 1 .000 .446 PRREC(2) -.575 .184 9.734 1 .002 .563 PRREC(3) .019 .170 .013 1 .911 1.019 PRREC(4) -.209 .172 1.480 1 .224 .811 Constant -.149 .158 .896 1 .344 .861 a. Variable(s) entered on step 1: PRREC. ROC Curve Case Processing Summary Subseq Parole Violations Valid N (listwise) Positivea 1076 Negative 2924 Larger values of the test result variable(s) indicate stronger evidence for a positive actual state. a. The positive actual state is Yes. Area Under the Curve Asymptotic 95% Confidence Interval Test Result Variable(s) Std. Errora Area Asymptotic Sig.b Lower Bound Upper Bound Prison Punish Rec. .575 .010 .000 .555 .595 Prior Record .590 .010 .000 .569 .610 Predicted probability .620 .010 .000 .600 .640 The test result variable(s): Prison Punish Rec., Prior Record, Predicted probability has at least one tie between the positive actual state group and the negative actual state group. Statistics may be biased. a. Under the nonparametric assumption b. Null hypothesis: true area = 0.5 Univariate Statistics Missing N Count Percent PPUNREC 4000 0 .0 PRREC 4000 0 .0 SUBPARVIO 4000 0 .0 Regression Variables Entered/Removeda Variables Variables Entered Removed Model 1 Method Prior Record, Prison Punish Rec. . Enter b a. Dependent Variable: Subseq Parole Violations b. All requested variables entered. Model Summary Model 1 R .187 R Square a .035 Adjusted R Std. Error of the Square Estimate .034 a. Predictors: (Constant), Prior Record, Prison Punish Rec. .436 ANOVAa Model 1 Sum of Squares Regression df Mean Square 27.460 2 13.730 Residual 759.096 3997 .190 Total 786.556 3999 F Sig. .000b 72.294 a. Dependent Variable: Subseq Parole Violations b. Predictors: (Constant), Prior Record, Prison Punish Rec. Coefficientsa Model 1 Unstandardized Standardized Coefficients Coefficients B Std. Error Collinearity Statistics Beta (Constant) .195 .009 Prison Punish Rec. .062 .010 Prior Record .048 .005 t Sig. Tolerance VIF 21.019 .000 .102 6.446 .000 .968 1.033 .139 8.828 .000 .968 1.033 a. Dependent Variable: Subseq Parole Violations Collinearity Diagnosticsa Variance Proportions Prison Punish Model Dimension Eigenvalue Condition Index (Constant) Rec. Prior Record 1 1 2.059 1.000 .10 .10 .10 2 .556 1.925 .00 .50 .67 3 .385 2.311 .90 .40 .22 a. Dependent Variable: Subseq Parole Violations Logistic Regression Subsequent Parole Violation as a function of Prior Punishment Received and Prior Record. Variables in the Equation B Step 1a S.E. PPUNREC Wald df Sig. 44.853 2 .000 Exp(B) PPUNREC(1) -.498 .102 23.712 1 .000 .608 PPUNREC(2) -.002 .113 .000 1 .983 .998 87.301 4 .000 PRREC PRREC(1) -.807 .148 29.571 1 .000 .446 PRREC(2) -.575 .184 9.734 1 .002 .563 PRREC(3) .019 .170 .013 1 .911 1.019 PRREC(4) -.209 .172 1.480 1 .224 .811 Constant -.149 .158 .896 1 .344 .861 a. Variable(s) entered on step 1: PPUNREC, PRREC. \f
Step by Step Solution
There are 3 Steps involved in it
Step: 1
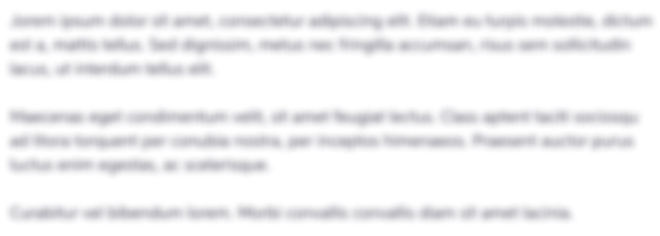
Get Instant Access to Expert-Tailored Solutions
See step-by-step solutions with expert insights and AI powered tools for academic success
Step: 2

Step: 3

Ace Your Homework with AI
Get the answers you need in no time with our AI-driven, step-by-step assistance
Get Started