Answered step by step
Verified Expert Solution
Question
1 Approved Answer
Good communication is just as stimulating as black coffee and just as hard to sleep after. - Anne Morrow Lindbergh In May 2021, David Black,
Step by Step Solution
There are 3 Steps involved in it
Step: 1
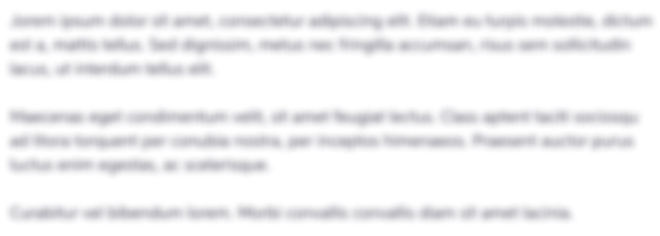
Get Instant Access to Expert-Tailored Solutions
See step-by-step solutions with expert insights and AI powered tools for academic success
Step: 2

Step: 3

Ace Your Homework with AI
Get the answers you need in no time with our AI-driven, step-by-step assistance
Get Started