Answered step by step
Verified Expert Solution
Question
1 Approved Answer
Location Income ($1000) Size Years Credit Balance($) Urban 54 3 12 4016 Rural 30 2 12 3159 Suburban 32 4 17 5100 Suburban 50 5
Location Income ($1000) Size Years Credit Balance($) Urban 54 3 12 4016 Rural 30 2 12 3159 Suburban 32 4 17 5100 Suburban 50 5 14 4742 Rural 31 2 4 1864 Urban 55 2 9 4070 Rural 37 1 20 2731 Urban 40 2 7 3348 Suburban 66 4 10 4764 Urban 51 3 16 4110 Urban 25 3 11 4208 Urban 48 4 16 4219 Rural 27 1 19 2477 Rural 33 2 12 2514 Urban 65 3 12 4214 Suburban 63 4 13 4965 Urban 55 6 15 4412 Urban 21 2 18 2448 Rural 44 1 7 2995 Urban 37 5 5 4171 Suburban 62 6 13 5678 Urban 21 3 16 3623 Suburban 55 7 15 5301 Rural 42 2 19 3020 Urban 41 7 18 4828 Suburban 54 6 14 5573 Rural 30 1 14 2583 Urban 48 2 8 3866 Urban 34 5 5 3586 Suburban 67 4 13 5037 Rural 50 2 11 3605 Urban 67 5 1 5345 Urban 55 6 10 5370 Urban 52 2 11 3890 Urban 62 3 2 4705 Urban 64 2 6 4157 Suburban 22 3 18 3899 Urban 29 4 4 3890 Suburban 39 2 18 2972 Rural 35 1 11 3121 Urban 39 4 15 4183 Suburban 54 3 9 3730 Suburban 23 6 18 4127 Rural 27 2 1 2921 Urban 26 7 17 4603 Suburban 61 2 14 4273 Rural 30 2 14 3067 Rural 22 4 16 3074 Suburban 46 5 13 4820 Suburban 66 4 20 5149 Chowdhury 1 The Seven elements of a Test of Hypothesis are: 1. Null Hypothesis - A theory about the specific values of one or more population parameters. The theory generally represents the status quo, and we accept it until proven false. 2. Alternative (research) hypothesis (Ha)- A theory about the specific values of one or more population parameters. The theory generally represents the status quo, and we accept it until proven false 3. Test statistic - A sample statistic used to decide whether to reject the null hypothesis. 4. Rejection Region - The numerical values of the test statistic for which the null hypothesis will be rejected. 5. Assumptions- Clear statements of any assumptions made about the populations being sampled. 6. Experiment and calculation of test statistics- Performance of the sampling experiment and determination of the numerical value of the test statistic. 7. Conclusiona. If the numerical value of the test statistic falls in the rejection region then we reject the null hypothesis and conclude that the alternative is true. b. If the test statistic does not fall in the rejection region, then we do not reject H0 as we have insufficient data to do so. a. The average (mean) annual income was less than $50,000 I found that the average annual incomes are 43.74 or $46,060, and the standard deviation to be 14.64 or $14.064. Set up Hypothesis Test o Ho: =50 o H1: <50 for a= 0.5 and \"<\" in the ha, i found that z so \"rejection region\" would be z<-1.645 1 chowdhury next calculated test statistic, using formula below to calculate statistic z. x u0 where is mean null hypothesis s>0.40 In order to conduct the large sample z-test, we first need to verify that the sample size is large enough. o nPo= 50(0.40) = 20 and 50 (1-0.44) = 30, both are larger than 15, so we can conclude that sample size is large enough to apply the large sample z test. 2 Chowdhury 1 Z= (0.44 - 0.400)/ 0.69282= 0.58 where s phat= sqrt (((0.40) (0.60))/50= 0.069282 This is a one tailed (upper or right since HA has \">\"). Our rejection regions would be z > 1.645. 0.58 is not greater than 1.645 (and is not in the rejection regions) so we would notReject the Ho. The p-value= 0.282. If the p-value is less than alpha, reject the null hypothesis and accept the alternative hypothesis, at the given alpha. Because the p-value = 0.282 is more than alpha = 0.05: we do not reject the null hypothesis H0: =40 and we do not accept the alternative hypothesis Ha: <40, at =.05. Since we are not rejecting the Ho, we are saying there is insufficient evidence to conclude the true population of customers who live in the Suburban location is greater than 40%. c. The average (mean) number of years lived in the current home is less than 13 years. o The average number of years in the current home form survey data to be 12.260, and the standard deviation to be 5.086 o Set up Hypothesis Test Ho: u = 13 H1: u<13 For a = 0005 and \"<\" in the Ha, I found that z= -1.645, so the \"rejection Region would be z < -1.645 Now we calculate the test statistic where u0 is the mean in the null hypothesis and x = s/ n z x u0 x 3 Chowdhury 1 x = 5.086/ z= (12.26 -13)/0.7193= -1.03, because n (50)= 0.7193 Because the p-value = 0.152 is more than alpha = 0.05: we do not reject the null hypothesis H0: =13 and we do not accept the alternative hypothesis Ha: <13, at =.05. Test statistic of -1.03 does not fall in the rejection region of z < -1.645, therefore, we would not reject the null hypothesis and say there is insufficient evidence to indicate U<13 d. the average (mean) credit balance for suburban customers is more than $4300. o we found rhe those surveyed $3970, and standard deviation 932. set up hypothesis test ho: u h1:> 4300 For a = .05 and \">\" in the Ha, I found z= 1.645, so the Rejection Region would be z > 1.645. Now we calculate the test statistic z x u0 x where u0 is the mean in the null hypothesis and x = s/ n z= (3970- 4300)/131.8 = -2.50, because x = 932/ n (50)= 131.8 The p-value= 0.994. If the p-value is less than alpha, reject the null hypothesis and accept the alternative hypothesis, at the given alpha. Because the p-value = 0.994 is not less than alpha = .05: we do not reject the null hypothesis H0: =4300 and we do not accept the alternative hypothesis Ha: >4300 at =.05. 4 Chowdhury 1 Test statistic of -2.50 does not fall in the rejection region of Z> -1.645, therefore, we would NOT reject the null hypothesis and say there is insufficient evidence to indicate U>4300. Appendix 2) Follow this up with computing 95% confidence intervals for each of the variables described in a.-d., and gain interpreting these intervals. 5 Chowdhury 1 a. The average (mean) annual income was less than $50,000 One-Sample Z: Income ($1000) The assumed standard deviation = 14.64 Variable Income ($1000) N Mean 50 43.74 StDev 14.64 SE Mean 2.07 95% CI (39.68, 47.80) Conclusion: According to the confidence interval, we are 95% confident that the true mean income lies between $39,680 and $47,800. b. The true population proportion of customers who live in an urban area exceeds 40% Sample X N Sample p 95% CI Z-Value P-Value 1 22 50 0.44 (0.302411, 0.577589) 0.58 0.564 Conclusion: According to the confidence interval, we are 95% confident that the mean population lies between 0.302 and 0.577. c. The average (mean) number of years lived in the current home is less than 13 years One-Sample Z: Income ($1000) The assumed standard deviation = 5.086 Variable Income ($1000) N Mean 50 43.740 StDev 14.640 SE Mean 95% CI 0.719 (42.330, 45.150) Conclusion: According to the confidence interval, we are 95% confident that the average mean of people living in their current homes lies between 42.33 and 45.15. d. The average (mean) credit balance for suburban customers is more than $4300 One-Sample Z: Credit Balance($) The assumed standard deviation = 932 Variable Credit Balance($) N Mean 50 3970 StDev 932 SE Mean 132 95% CI (3712, 4229) Conclusion: We are 95% confident that the true mean credit balance lies between $3,712 and $4,229. Chowdhury 1 Minitab calculations for first part of Part B Project a. The average (mean) annual income was less than $50,000Descriptive Statistics: Income ($1000) Descriptive Statistics: Income ($1000) Variable Income ($1000) Mean 43.74 StDev 14.64 Minimum 21.00 Maximum 67.00 One-Sample Z Test of mu = 50 vs < 50 The assumed standard deviation = 14.64 N 50 Mean 43.74 SE Mean 2.07 95% Upper Bound 47.15 Z -3.02 P 0.001 Distribution Plot Normal, Mean=0, StDev=1 0.4 Density 0.3 0.2 0.1 0.05 0.0 -1.645 0 X b.The true population proportion of customers who live in an urban area exceeds 40%. Location Rural Suburban Urban N= Count 13 15 22 50 Percent 26.00 30.00 44.00 Test and CI for One Proportion Test of p = 0.4 vs p > 0.4 Chowdhury 1 Sample 1 X 22 N 50 Sample p 0.440000 95% Lower Bound 0.324532 Z-Value 0.58 P-Value 0.282 Test and CI for One Proportion Sample 1 X 22 N 50 Sample p 0.440000 95% CI (0.302411, 0.577589) c.The average (mean) number of years lived in the current home is less than 13 years Descriptive Statistics: Years Variable Years Mean 12.260 StDev 5.086 Minimum 1.000 Maximum 20.000 One-Sample Z: Years Test of mu = 13 vs < 13 The assumed standard deviation = 5.086 Variable Years N 50 Mean 12.260 StDev 5.086 SE Mean 0.719 95% Upper Bound 13.443 Z -1.03 P 0.152 Distribution Plot Normal, Mean=0, StDev=1 0.4 Density 0.3 0.2 0.1 0.05 0.0 -1.645 0 X d. The average (mean) credit balance for suburban customers is more than $4300 Descriptive Statistics: Credit Balance($) Chowdhury 1 Variable Credit Balance($) Mean 3970 StDev 932 Minimum 1864 Maximum 5678 One-Sample Z: Credit Balance($) Test of mu = 4300 vs > 4300 The assumed standard deviation = 932 Variable Credit Balance($) N 50 Mean 3970 StDev 932 SE Mean 132 95% Lower Bound 3754 Distribution Plot Normal, Mean=0, StDev=1 0.4 Density 0.3 0.2 0.1 0.05 0.0 0 X 1.645 Z -2.50 P 0.994 Location Income ($1000) Size Years Credit Balance($) Urban 54 3 12 4016 Rural 30 2 12 3159 Suburban 32 4 17 5100 Suburban 50 5 14 4742 Rural 31 2 4 1864 Urban 55 2 9 4070 Rural 37 1 20 2731 Urban 40 2 7 3348 Suburban 66 4 10 4764 Urban 51 3 16 4110 Urban 25 3 11 4208 Urban 48 4 16 4219 Rural 27 1 19 2477 Rural 33 2 12 2514 Urban 65 3 12 4214 Suburban 63 4 13 4965 Urban 55 6 15 4412 Urban 21 2 18 2448 Rural 44 1 7 2995 Urban 37 5 5 4171 Suburban 62 6 13 5678 Urban 21 3 16 3623 Suburban 55 7 15 5301 Rural 42 2 19 3020 Urban 41 7 18 4828 Suburban 54 6 14 5573 Rural 30 1 14 2583 Urban 48 2 8 3866 Urban 34 5 5 3586 Suburban 67 4 13 5037 Rural 50 2 11 3605 Urban 67 5 1 5345 Urban 55 6 10 5370 Urban 52 2 11 3890 Urban 62 3 2 4705 Urban 64 2 6 4157 Suburban 22 3 18 3899 Urban 29 4 4 3890 Suburban 39 2 18 2972 Rural 35 1 11 3121 Urban 39 4 15 4183 Suburban 54 3 9 3730 Suburban 23 6 18 4127 Rural 27 2 1 2921 Urban 26 7 17 4603 Suburban 61 2 14 4273 Rural 30 2 14 3067 Rural 22 4 16 3074 Suburban 46 5 13 4820 Suburban 66 4 20 5149 1. Generate a scatterplot for CREDIT BALANCE vs. SIZE, including the graph of the "best fit" line. Interpret. Scatterplot of Credit Balance($) vs Size 6000 Credit Balance($) 5000 4000 3000 2000 1 2 3 4 5 6 7 Size Plots are upward sloping and fairly aligned almost in a straight lined which suggest of fairly positive linear relationship between variables 2. Determine the equation of the "best fit" line, which describes the relationship between CREDIT BALANCE and SIZE. Coefficients Term Coef SE Coef T-Value P-Value VIF Constant 2591 195 13.29 0.000 Size 403.2 50.9 7.91 0.000 1.00 Regression Equation Credit Balance($) = 2591 + 403.2 Size Since the slope is positive, the relationship is positive. One unit change in price increases Credit balance by $ 403.2 Credit balance is equal to 2591 when size is equal to zero 3. Determine the coefficient of correlation. Interpret. Correlation: Size, Credit Balance($) Pearson correlation of Size and Credit Balance($) = 0.752 P-Value = 0.000 R= 0.752 This is a strong positive correlation/relationship between this two variables 4. Determine the coefficient of determination. Interpret. R2=0.5662 This means 56.62% of variation in credit balance is explained by size 5. Test the utility of this regression model (use a two tail test with =.05). Interpret your results, including the p-value. H0: model is NOT significant Ha: Model is significant Regression Analysis: Credit Balance($) versus Size Analysis of Variance Source DF Adj SS Adj MS F-Value P-Value Regression 1 24092210 24092210 62.64 0.000 Size 1 24092210 24092210 62.64 0.000 Error 48 18460853 384601 Lack-of-Fit 5 2499467 499893 1.35 0.263 Pure Error 43 15961386 371195 Total 49 42553062 P-value=0.000 Since p-value<0.025 we reject H0 and conclude that the general model is significant 6. Based on your findings in 1-5, what is your opinion about using SIZE to predict CREDIT BALANCE? Explain. It will be okay to use size to predict credit balance since from 1-5, it has been proven that there is linear relationship between this two variables. However, considering that size has only 56.62% effect on variation is credit balance, other variables that affect credit balance should be incorporated. 7. Compute the 95% confidence interval for 1 (the population slope). Interpret this interval. (300.8, 505.7) This means that we are 95% confidence that the slope will be within this interval 8. Using an interval, estimate the average credit balance for customers that have household size of 5. Interpret this interval. (3337.9, 5877.2) This means that 95% of the customers that have household size of 5 will have credit balance that is within this interval 9. Using an interval, predict the credit balance for a customer that has a household size of 5. Interpret this interval. (3331.6, 5873.6) This means we are 95% confidence that a the credit balance for a customer that has a household size of 5 will be within this interval 10. What can we say about the credit balance for a customer that has a household size of 10? Explain your answer. No, Since 10 is out of range of the values used as predictor as the maximum value used is 7, predicting credit balance at size 10 will be inaccurate. In an attempt to improve the model, we attempt to do a multiple regression model predicting CREDIT BALANCE based on INCOME, SIZE and YEARS. 11. Using MINITAB run the multiple regression analysis using the variables INCOME, SIZE and YEARS to predict CREDIT BALANCE. State the equation for this multiple regression model. Coefficients Term Coef SE Coef T-Value P-Value VIF Constant 1276 274 4.66 0.000 Income ($1000) 32.27 4.35 7.42 0.000 1.10 Size 346.9 36.0 9.63 0.000 1.07 Years 7.9 12.3 0.64 0.526 1.07 Regression Equation Credit Balance($) = 1276 + 32.27 Income ($1000) + 346.9 Size + 7.9 Years 12. Perform the Global Test for Utility (F-Test). Explain your conclusion. Analysis of Variance Source DF Seq SS Seq MS F-Value P-Value Regression 3 34255444 11418481 63.30 0.000 Income ($1000) 1 16703393 16703393 92.60 0.000 Size 1 17478430 17478430 96.90 0.000 Years 1 73620 73620 0.41 0.526 Error 46 8297619 180383 Total 49 42553062 Since p-value for the general model is less than 0.05, the model is significant. All the predictors significantly contribute to the model apart from year as its p-value=0.526>0.05 13. Perform the t-test on each independent variable. Explain your conclusions and clearly state how you should proceed. In particular, which independent variables should we keep and which should be discarded. Coefficients Term Coef SE Coef T-Value P-Value VIF Constant 1276 274 4.66 0.000 Income ($1000) 32.27 4.35 7.42 0.000 1.10 Size 346.9 36.0 9.63 0.000 1.07 Years 7.9 12.3 0.64 0.526 1.0 Income and Size significantly contribute to the model but years does not as its pvalue=0.526>0.05 Therefore keep income and size. Discharge Years. 14. Is this multiple regression model better than the linear model that we generated in parts 110? Explain. Yes, since 80.50% of credit balance is explained by new model as compared to only 56.62% in the previous model PROJECT PART C Reliable Housewares is a local store that sells many household items and issues its own credit card to its customers. The store manager wants to study the purchasing behavior of its "credit" customers. To that end, he has come to DeVry and asked our MBA students for help. The manager has brought with him data on five variables of 50 randomly selected credit customers. LOCATION (Rural, Urban, Suburban - Household location of the credit customer) INCOME (in $1,000's - be careful with this) SIZE (Household Size - number of people living in the household of credit customer) YEARS (the number of years that the customer has lived in the current location) CREDIT BALANCE ($ balance on customer's store credit card) Regression and Correlation Analysis Using MINITAB perform the regression and correlation analysis for the data on CREDIT BALANCE (Y) and SIZE (X) by answering the following. 1. Generate a scatterplot for CREDIT BALANCE vs. SIZE, including the graph of the "best fit" line. Interpret. 2. Determine the equation of the "best fit" line, which describes the relationship between CREDIT BALANCE and SIZE. 3. Determine the coefficient of correlation. Interpret. 4. Determine the coefficient of determination. Interpret. 5. Test the utility of this regression model (use a two tail test with =.05). Interpret your results, including the p-value. 6. Based on your findings in 1-5, what is your opinion about using SIZE to predict CREDIT BALANCE? Explain. 7. Compute the 95% confidence interval for 1 (the population slope). Interpret this interval. 8. Using an interval, estimate the average credit balance for customers that have household size of 5. Interpret this interval. 9. Using an interval, predict the credit balance for a customer that has a household size of 5. Interpret this interval. 10. What can we say about the credit balance for a customer that has a household size of 10? Explain your answer. 1|Page In an attempt to improve the model, we attempt to do a multiple regression model predicting CREDIT BALANCE based on INCOME, SIZE and YEARS. 11. Using MINITAB run the multiple regression analysis using the variables INCOME, SIZE and YEARS to predict CREDIT BALANCE. State the equation for this multiple regression model. 12. Perform the Global Test for Utility (F-Test). Explain your conclusion. 13. Perform the t-test on each independent variable. Explain your conclusions and clearly state how you should proceed. In particular, which independent variables should we keep and which should be discarded. 14. Is this multiple regression model better than the linear model that we generated in parts 1-10? Explain. All DeVry University policies are in effect, including the plagiarism policy. Project Part C report is due by the end of Week 7. Project Part C is worth 100 total points. See grading rubric below. Summarize your results from 1-14 in a report that is three pages or less in length and explains and interprets the results in ways that are understandable to someone who does not know statistics. Submission: A report in Microsoft Word containing the summary report + all of the work done in 1-14 (Minitab Output + interpretations) as an appendix. Report Format: A. Summary Report B. Bullets 1-14 addressed with appropriate output, graphs and interpretations. Be sure to number each bullet 1-14. Project Part C: Grading Rubric Category Points Description Questions 1 - 12 and 14 - 5 pts. each 65 addressed with appropriate output, graphs and interpretations Question 13 15 addressed with appropriate output, graphs and interpretations 20 writing, grammar, clarity, logic, and cohesiveness 100 A quality paper will meet or exceed all of the above requirements. Summary Total 2|Page Reliable Housewares Summary Report Thank you for providing the data for 50 of your \"credit\" customers, it was very helpful in allowing the analysis of these customers. The following sections will break down your suppositions regarding the purchasing behavior of your \"credit\" customers. Your beliefs were partially correct and each section below will detail the statistical reasoning behind the truth or error of these beliefs. Section A You are pretty sure that the average income of your \"credit\" customers is less than $50,000. You are correct! The data provided showed that the customer's average income is actually $43,740. This is statistically significantly (that means a lot) below the $50,000 that you believed. In fact, we can be pretty sure, 95% confident in fact, that their incomes range from $39,682.10 to $47,797.90. A few of the customers, 19 to be exact, have incomes greater than $50,000, but the overall average is as you suspected. The 10 customers with incomes less than $30,000 pull the average down to where you suspected. Section B You are pretty sure that the fraction of your customers who live in an urban area is greater than 40%. Sorry, wrong on this one. The data provided showed that the number of customers that live in an urban area is 22. That is 44% the 50 customers in the provided data. Sadly, this is not statistically significantly above the 40% that you believe. In fact, we can be 95% confident that they true percentage lies between 36.98% and 51.02%. That low end of 36.98% makes it just low enough so that we can't be sure, based on the data provided, that your belief on the customers is correct. Section C You are pretty sure that the average number of years that your customers have lived in their current homes is less than 13 years. Sorry, wrong again! The data provided showed that the customer's average year in their current home is 12.26 years. While is number is less than 13 years, is it just not statistically significantly below the 13 years that you thought. We can be 95% confident that their years in their current homes range from 10.85 to 13.67 years. That 13.67 top end is enough to make one not sure enough to back up your belief. Close, but just not quite. Section D You are pretty sure that the average credit balance for your suburban customers is more than $4,300. This one you got right! The data provided showed that the suburban customer's average credit balance is a whopping $4,675.33. This is statistically significantly above the $4,300 that you thought. For your information, the 95% confidence range of credit balances for your suburban customers is $4,450.14, $4,900.53. While 5 of your 15 suburban customers have a balance of less than $4,300, the bigger balances bring the overall average up to where you believed that it was. Appendix A 1. Null hypothesis (H0): $50,000 (Average annual income of credit customers is more than $50,000.) 2. Alternative hypothesis (Ha): < $50,000 (Average annual income of credit customers is less than $50,000.) (The claim) 3. Test statistic: z= x x x 4374050000 = = = x s 14639.6 n n 50 -3.0236 4. Rejection region: z < -1.645, which corresponds to = 0.05. 5. Conclusion: The sample mean lies 3.02 sample standard deviations below the hypothesized value of $50,000. Since this value of z exceeds (is much less than) -1.645, it falls into the rejection region. That is, we reject the null hypothesis that $50,000 and conclude that < $50,000. Thus, it appears that the average annual income of credit customers is less than $50,000. Confidence Interval: Now, we use the one-tailed value of z for = 0.05, which is 1.96. CI =x z s 1.9614639.6 =$ 43,740 = ($39,682.10, $47,797.90) n 50 Minitab Output: One-Sample Z: Income ($1000) Test of = 50 vs < 50 The assumed standard deviation = 14.639 Variable Income ($1000) N 50 Mean 43.74 StDev 14.64 SE Mean 2.07 Boxplot of Income ($1000) (with Ho and 95% Z-confidence interval for the Mean, and StDev = 14.6396) _ X Ho 20 30 40 50 Income ($1000) 60 70 95% Upper Bound 47.15 Z -3.02 P 0.001 Appendix B 1. Null hypothesis (H0): p 0.4 (The true population proportion of credit customers who live in an urban area is less than or equal to 40%.) 2. Alternative hypothesis (Ha): p > 0.4 (The true population proportion of credit customers who live in an urban area greater than 40%.) (The claim) z= 3. Test statistic: ^p p0 p0 (1 p 0) n = .44.4 = .4 (1.4 ) .5773 50 4. Rejection region: z > 1.645, which corresponds to = 0.05. 5. Conclusion: The sample proportion lies .5773 sample standard deviations above the hypothesized value of p 0.4. Since this value of z does not exceeds 1.645, it does not fall into the rejection region. That is, we do not reject the null hypothesis that p 0.4. Thus, the true population proportion of credit customers who live in an urban area is less than or equal to 40%.) Confidence Interval: Now, we use the one-tailed value of z for = 0.05, which is 1.96. CI = ^p z ^p ( 1 ^p ) .44 ( 1.44 ) =0.40 1.96 = (.3698, .5102) => (36.98%, 51.02%) n 50 Minitab Output: Test and CI for One Proportion Test of p = 0.4 vs p > 0.4 Sample 1 X 22 N 50 Sample p 0.440000 95% Lower Bound 0.324532 Using the normal approximation. Z-Value 0.58 P-Value 0.282 Appendix C 1. Null hypothesis (H0): 13 (The average number of years lived in the current home is greater than or equal to 13 years.) 2. Alternative hypothesis (Ha): < 13 (The average number of years lived in the current home is less than 13 years.) (The claim) z= 3. Test statistic: x x x 12.2613 = = = x s 5.086 -1.028 n n 50 4. Rejection region: z < -1.645, which corresponds to = 0.05. 5. Conclusion: The sample mean lies 1.028 below the hypothesized value of 13. Since this value of z does not exceed (is less than) -1.645, it does not fall into the rejection region. That is, we do not reject the null hypothesis that 13. Thus, it appears that the average number of years lived in the current home is greater than or equal to 13 years. Confidence Interval: Now, we use the one-tailed value of z for = 0.05, which is 1.96. CI =x z s 1.965.086 =$ 13 = (10.85, 13.67) years n 50 Minitab Output: One-Sample Z: Years Test of = 13 vs < 13 The assumed standard deviation = 5.086 Variable Years N 50 Mean 12.260 StDev 5.086 SE Mean 0.719 95% Upper Bound 13.443 Boxplot of Years (with Ho and 95% Z-confidence interval for the Mean, and StDev = 5.086) _ X Ho 0 5 10 Years 15 20 Z -1.03 P 0.152 Appendix D 1. Null hypothesis (H0): $4,300 (The credit balance for suburban customers is less than or equal to $4,300.) 2. Alternative hypothesis (Ha): > $4,300 (The credit balance for suburban customers is greater than $4,300.) (The claim) 3. Because there are only 15 suburban customers, (n < 30) we must use the t test. The degrees of freedom (df) is one less than the number of customers (n - 1) = 14. Test statistic: t= x x x 4675.334300 = = = x s 742.365 3.575 n n 50 4. Rejection region: t > 1.96, which corresponds to = 0.05 for a one-tailed t test with 14 degrees of freedom. 5. Conclusion: The sample mean lies well above the hypothesized value of $4,300. Since the calculated value of t exceeds 2.145, it falls into the rejection region. That is, we reject the null hypothesis that $4,300 and conclude the credit balance for suburban customers is greater than $4,300. Thus, it appears that the credit balance for suburban customers is greater than $4,300. Confidence Interval: CI =x t s 2.145742.365 =$ 4,300 = ($4,450.14, $4,900.53) n 50 Minitab output: One-Sample T: Credit Balance($) Test of = 4300 vs > 4300 Variable Credit Balance($) N 15 Mean 4675 StDev 742 SE Mean 192 95% Lower Bound 4338 Boxplot of Credit Balance($) (with Ho and 95% t-confidence interval for the mean) _ X Ho 3000 3500 4000 4500 Credit Balance($) 5000 5500 6000 T 1.96 P 0.035 1. Generate a scatterplot for CREDIT BALANCE vs. SIZE, including the graph of the "best fit" line. Interpret. Scatterplot of Credit Balance($) vs Size 6000 Credit Balance($) 5000 4000 3000 2000 1 2 3 4 5 6 7 Size Plots are upward sloping and fairly aligned almost in a straight lined which suggest of fairly positive linear relationship between variables 2. Determine the equation of the "best fit" line, which describes the relationship between CREDIT BALANCE and SIZE. Coefficients Term Constant Size Coef SE Coef T-Value 2591 195 13.29 403.2 50.9 7.91 P-Value 0.000 0.000 VIF 1.00 Regression Equation Credit Balance($) = 2591 + 403.2 Family Size Since the slope is positive, the relationship is positive. One unit change in price increases Credit balance by $ 403.2 Credit balance is equal to 2591 when size is equal to zero 3. Determine the coefficient of correlation. Interpret. Correlation: Size, Credit Balance($) Pearson correlation of Family Size and Credit Balance($) = 0.752 R= 0.752 This is a strong positive correlation/relationship between these two variables 4. Determine the coefficient of determination. Interpret. R2=0.5662 This means 56.62% of variation in credit balance is explained by family size. 5. Test the utility of this regression model (use a two tail test with =.05). Interpret your results, including the p-value. H0: Model is NOT significant Ha: Model is significant Regression Analysis: Credit Balance($) versus Family Size Analysis of Variance Source Regression Size Error Lack-of-Fit Pure Error Total DF 1 1 48 5 43 49 Adj SS 24092210 24092210 18460853 2499467 15961386 42553062 Adj MS 24092210 24092210 384601 499893 371195 F-Value 62.64 62.64 P-Value 0.000 0.000 1.35 0.263 P-value=0.000 Since p-value<0.025 we reject H0 and conclude that the general model is significant 6. Based on your findings in 1-5, what is your opinion about using SIZE to predict CREDIT BALANCE? Explain. It will be okay to use size to predict credit balance since from 1-5, it has been proven that there is linear relationship between this two variables. However, considering that size has only 56.62% effect on variation is credit balance, other variables that affect credit balance should be incorporated. 7. Compute the 95% confidence interval for 1 (the population slope). Interpret this interval. (300.8, 505.7) This means that we are 95% confidence that the slope will be within this interval. 8. Using an interval, estimate the average credit balance for customers that have household size of 5. Interpret this interval. (3337.9, 5877.2) This means that we can be 95% confident that the customers that have household size of 5 will have an average credit balance that is within this interval. 9. Using an interval, predict the credit balance for a customer that has a household size of 5. Interpret this interval. (3331.6, 5873.6) This means that we can be 95% confident that the credit balance for a chosen customer that has a household size of 5 will be within this interval. 10. What can we say about the credit balance for a customer that has a household size of 10? Explain your answer. No, Since 10 is out of range of the values used as predictor as the maximum value used is 7, predicting credit balance at size 10 will be inaccurate. In an attempt to improve the model, we attempt to do a multiple regression model predicting CREDIT BALANCE based on INCOME, SIZE and YEARS. 11. Using MINITAB run the multiple regression analysis using the variables INCOME, SIZE and YEARS to predict CREDIT BALANCE. State the equation for this multiple regression model. Coefficients Term Constant Income ($1000) Size Years Coef 1276 32.27 346.9 7.9 SE Coef 274 4.35 36.0 12.3 T-Value 4.66 7.42 9.63 0.64 P-Value 0.000 0.000 0.000 0.526 VIF 1.10 1.07 1.07 Regression Equation Credit Balance($) = 1276 + 32.27 Income ($1000) + 346.9 Size + 7.9 Years 12. Perform the Global Test for Utility (F-Test). Explain your conclusion. Analysis of Variance Source Regression Income ($1000) Size Years Error Total DF 3 1 1 1 46 49 Seq SS 34255444 16703393 17478430 73620 8297619 42553062 Seq MS 11418481 16703393 17478430 73620 180383 F-Value 63.30 92.60 96.90 0.41 P-Value 0.000 0.000 0.000 0.526 Since p-value for the general model is less than 0.05, the model is significant. All the predictors significantly contribute to the model apart from year as its p-value=0.526>0.05 13. Perform the t-test on each independent variable. Explain your conclusions and clearly state how you should proceed. In particular, which independent variables should we keep and which should be discarded. Coefficients Term Constant Income ($1000) Size Years Coef 1276 32.27 346.9 7.9 SE Coef 274 4.35 36.0 12.3 T-Value 4.66 7.42 9.63 0.64 P-Value 0.000 0.000 0.000 0.526 VIF 1.10 1.07 1.0 Income and Size significantly contribute to the model but years does not as its pvalue=0.526>0.05 Therefore keep income and size. Discard Years. 14. Is this multiple regression model better than the linear model that we generated in parts 110? Explain. Yes, since 80.50% of credit balance is explained by new model as compared to only 56.62% in the previous model Summary of Report Family size can definitely have a big impact on your credit balance. Family income is also a good predictor of credit balance, but years in their current home was not as helpful. When tests were run to see if credit balance went up when the family size when up, one can find the data to be very related. For each new family member added, the credit balance of the family was shown to go up by $403.20. This report showed that using family size alone, in this set of customers, one can predict their credit balance for a family of size 5 within a few thousand dollars, $3337.90 to $5877.20 to be exact. The statistical tests used showed that the probability of this relationship being by chance was extremely low (less than 0.1 %). However, statistical testing showed that family size along only explained about half (56.62%) of the variability in credit balance was explained by family size. That leads one to wonder, what other variables could affect credit balance? Next, statistical tests were run where we added the additional dimensions of family income and years in their current home. The family income helped a lot to explain the credit balance, but years in their current home was not as helpful. For every $1000 increase in family income, $32.27 was added to the credit balance. The statistical tests used showed that the probability of this relationship being by chance was extremely low (less than 0.1 %). Maybe people who earn more money feel comfortable holding more of a credit balance. With the addition of family income, we are not able to explain most (80.50%) of the variability in credit balance. Years in the current home was tested and it was determined that it did not predict the increase in credit balance statistically well, so it most likely should not be used in determining credit balance. The statistical test for years in the family home showed that its relation to credit balance could be entirely by chance. In conclusion, family size and family income level are good predictors of a family's credit balance, but years in their current home is not much of a help in this prediction. Reliable Housewares Summary Report Thank you for providing the data for 50 of your \"credit\" customers, it was very helpful in allowing the analysis of these customers. The following sections will break down your suppositions regarding the purchasing behavior of your \"credit\" customers. Your beliefs were partially correct and each section below will detail the statistical reasoning behind the truth or error of these beliefs. Section A You are pretty sure that the average income of your \"credit\" customers is less than $50,000. You are correct! The data provided showed that the customer's average income is actually $43,740. This is statistically significantly (that means a lot) below the $50,000 that you believed. In fact, we can be pretty sure, 95% confident in fact, that their incomes range from $39,682.10 to $47,797.90. A few of the customers, 19 to be exact, have incomes greater than $50,000, but the overall average is as you suspected. The 10 customers with incomes less than $30,000 pull the average down to where you suspected. Section B You are pretty sure that the fraction of your customers who live in an urban area is greater than 40%. Sorry, wrong on this one. The data provided showed that the number of customers that live in an urban area is 22. That is 44% the 50 customers in the provided data. Sadly, this is not statistically significantly above the 40% that you believe. In fact, we can be 95% confident that they true percentage lies between 36.98% and 51.02%. That low end of 36.98% makes it just low enough so that we can't be sure, based on the data provided, that your belief on the customers is correct. Section C You are pretty sure that the average number of years that your customers have lived in their current homes is less than 13 years. Sorry, wrong again! The data provided showed that the customer's average year in their current home is 12.26 years. While is number is less than 13 years, is it just not statistically significantly below the 13 years that you thought. We can be 95% confident that their years in their current homes range from 10.85 to 13.67 years. That 13.67 top end is enough to make one not sure enough to back up your belief. Close, but just not quite. Section D You are pretty sure that the average credit balance for your suburban customers is more than $4,300. This one you got right! The data provided showed that the suburban customer's average credit balance is a whopping $4,675.33. This is statistically significantly above the $4,300 that you thought. For your information, the 95% confidence range of credit balances for your suburban customers is $4,450.14, $4,900.53. While 5 of your 15 suburban customers have a balance of less than $4,300, the bigger balances bring the overall average up to where you believed that it was. Appendix A 1. Null hypothesis (H0): $50,000 (Average annual income of credit customers is more than $50,000.) 2. Alternative hypothesis (Ha): < $50,000 (Average annual income of credit customers is less than $50,000.) (The claim) 3. Test statistic: z= x x x 4374050000 = = = x s 14639.6 n n 50 -3.0236 4. Rejection region: z < -1.645, which corresponds to = 0.05. 5. Conclusion: The sample mean lies 3.02 sample standard deviations below the hypothesized value of $50,000. Since this value of z exceeds (is much less than) -1.645, it falls into the rejection region. That is, we reject the null hypothesis that $50,000 and conclude that < $50,000. Thus, it appears that the average annual income of credit customers is less than $50,000. Confidence Interval: Now, we use the one-tailed value of z for = 0.05, which is 1.96. CI =x z s 1.9614639.6 =$ 43,740 = ($39,682.10, $47,797.90) n 50 Minitab Output: One-Sample Z: Income ($1000) Test of = 50 vs < 50 The assumed standard deviation = 14.639 Variable Income ($1000) N 50 Mean 43.74 StDev 14.64 SE Mean 2.07 Boxplot of Income ($1000) (with Ho and 95% Z-confidence interval for the Mean, and StDev = 14.6396) _ X Ho 20 30 40 50 Income ($1000) 60 70 95% Upper Bound 47.15 Z -3.02 P 0.001 Appendix B 1. Null hypothesis (H0): p 0.4 (The true population proportion of credit customers who live in an urban area is less than or equal to 40%.) 2. Alternative hypothesis (Ha): p > 0.4 (The true population proportion of credit customers who live in an urban area greater than 40%.) (The claim) z= 3. Test statistic: ^p p0 p0 (1 p 0) n = .44.4 = .4 (1.4 ) .5773 50 4. Rejection region: z > 1.645, which corresponds to = 0.05. 5. Conclusion: The sample proportion lies .5773 sample standard deviations above the hypothesized value of p 0.4. Since this value of z does not exceeds 1.645, it does not fall into the rejection region. That is, we do not reject the null hypothesis that p 0.4. Thus, the true population proportion of credit customers who live in an urban area is less than or equal to 40%.) Confidence Interval: Now, we use the one-tailed value of z for = 0.05, which is 1.96. CI = ^p z ^p ( 1 ^p ) .44 ( 1.44 ) =0.40 1.96 = (.3698, .5102) => (36.98%, 51.02%) n 50 Minitab Output: Test and CI for One Proportion Test of p = 0.4 vs p > 0.4 Sample 1 X 22 N 50 Sample p 0.440000 95% Lower Bound 0.324532 Using the normal approximation. Z-Value 0.58 P-Value 0.282 Appendix C 1. Null hypothesis (H0): 13 (The average number of years lived in the current home is greater than or equal to 13 years.) 2. Alternative hypothesis (Ha): < 13 (The average number of years lived in the current home is less than 13 years.) (The claim) z= 3. Test statistic: x x x 12.2613 = = = x s 5.086 -1.028 n n 50 4. Rejection region: z < -1.645, which corresponds to = 0.05. 5. Conclusion: The sample mean lies 1.028 below the hypothesized value of 13. Since this value of z does not exceed (is less than) -1.645, it does not fall into the rejection region. That is, we do not reject the null hypothesis that 13. Thus, it appears that the average number of years lived in the current home is greater than or equal to 13 years. Confidence Interval: Now, we use the one-tailed value of z for = 0.05, which is 1.96. CI =x z s 1.965.086 =$ 13 = (10.85, 13.67) years n 50 Minitab Output: One-Sample Z: Years Test of = 13 vs < 13 The assumed standard deviation = 5.086 Variable Years N 50 Mean 12.260 StDev 5.086 SE Mean 0.719 95% Upper Bound 13.443 Boxplot of Years (with Ho and 95% Z-confidence interval for the Mean, and StDev = 5.086) _ X Ho 0 5 10 Years 15 20 Z -1.03 P 0.152 Appendix D 1. Null hypothesis (H0): $4,300 (The credit balance for suburban customers is less than or equal to $4,300.) 2. Alternative hypothesis (Ha): > $4,300 (The credit balance for suburban customers is greater than $4,300.) (The claim) 3. Because there are only 15 suburban customers, (n < 30) we must use the t test. The degrees of freedom (df) is one less than the number of customers (n - 1) = 14. Test statistic: t= x x x 4675.334300 = = = x s 742.365 3.575 n n 50 4. Rejection region: t > 1.96, which corresponds to = 0.05 for a one-tailed t test with 14 degrees of freedom. 5. Conclusion: The sample mean lies well above the hypothesized value of $4,300. Since the calculated value of t exceeds 2.145, it falls into the rejection region. That is, we reject the null hypothesis that $4,300 and conclude the credit balance for suburban customers is greater than $4,300. Thus, it appears that the credit balance for suburban customers is greater than $4,300. Confidence Interval: CI =x t s 2.145742.365 =$ 4,300 = ($4,450.14, $4,900.53) n 50 Minitab output: One-Sample T: Credit Balance($) Test of = 4300 vs > 4300 Variable Credit Balance($) N 15 Mean 4675 StDev 742 SE Mean 192 95% Lower Bound 4338 Boxplot of Credit Balance($) (with Ho and 95% t-confidence interval for the mean) _ X Ho 3000 3500 4000 4500 Credit Balance($) 5000 5500 6000 T 1.96 P 0.035 1. Generate a scatterplot for CREDIT BALANCE vs. SIZE, including the graph of the "best fit" line. Interpret. Scatterplot of Credit Balance($) vs Size 6000 Credit Balance($) 5000 4000 3000 2000 1 2 3 4 5 6 7 Size Plots are upward sloping and fairly aligned almost in a straight lined which suggest of fairly positive linear relationship between variables 2. Determine the equation of the "best fit" line, which describes the relationship between CREDIT BALANCE and SIZE. Coefficients Term Constant Size Coef SE Coef T-Value 2591 195 13.29 403.2 50.9 7.91 P-Value 0.000 0.000 VIF 1.00 Regression Equation Credit Balance($) = 2591 + 403.2 Family Size Since the slope is positive, the relationship is positive. One unit change in price increases Credit balance by $ 403.2 Credit balance is equal to 2591 when size is equal to zero 3. Determine the coefficient of correlation. Interpret. Correlation: Size, Credit Balance($) Pearson correlation of Family Size and Credit Balance($) = 0.752 R= 0.752 This is a strong positive correlation/relationship between these two variables 4. Determine the coefficient of determination. Interpret. R2=0.5662 This means 56.62% of variation in credit balance is explained by family size. 5. Test the utility of this regression model (use a two tail test with =.05). Interpret your results, including the p-value. H0: Model is NOT significant Ha: Model is significant Regression Analysis: Credit Balance($) versus Family Size Analysis of Variance Source Regression Size Error Lack-of-Fit Pure Error Total DF 1 1 48 5 43 49 Adj SS 24092210 24092210 18460853 2499467 15961386 42553062 Adj MS 24092210 24092210 384601 499893 371195 F-Value 62.64 62.64 P-Value 0.000 0.000 1.35 0.263 P-value=0.000 Since p-value<0.025 we reject H0 and conclude that the general model is significant 6. Based on your findings in 1-5, what is your opinion about using SIZE to predict CREDIT BALANCE? Explain. It will be okay to use size to predict credit balance since from 1-5, it has been proven that there is linear relationship between this two variables. However, considering that size has only 56.62% effect on variation is credit balance, other variables that affect credit balance should be incorporated. 7. Compute the 95% confidence interval for 1 (the population slope). Interpret this interval. (300.8, 505.7) This means that we are 95% confidence that the slope will be within this interval. 8. Using an interval, estimate the average credit balance for customers that have household size of 5. Interpret this interval. (3337.9, 5877.2) This means that we can be 95% confident that the customers that have household size of 5 will have an average credit balance that is within this interval. 9. Using an interval, predict the credit balance for a customer that has a household size of 5. Interpret this interval. (3331.6, 5873.6) This means that we can be 95% confident that the credit balance for a chosen customer that has a household size of 5 will be within this interval. 10. What can we say about the credit balance for a customer that has a household size of 10? Explain your answer. No, Since 10 is out of range of the values used as predictor as the maximum value used is 7, predicting credit balance at size 10 will be inaccurate. In an attempt to improve the model, we attempt to do a multiple regression model predicting CREDIT BALANCE based on INCOME, SIZE and YEARS. 11. Using MINITAB run the multiple regression analysis using the variables INCOME, SIZE and YEARS to predict CREDIT BALANCE. State the equation for this multiple regression model. Coefficients Term Constant Income ($1000) Size Years Coef 1276 32.27 346.9 7.9 SE Coef 274 4.35 36.0 12.3 T-Value 4.66 7.42 9.63 0.64 P-Value 0.000 0.000 0.000 0.526 VIF 1.10 1.07 1.07 Regression Equation Credit Balance($) = 1276 + 32.27 Income ($1000) + 346.9 Size + 7.9 Years 12. Perform the Global Test for Utility (F-Test). Explain your conclusion. Analysis of Variance Source Regression Income ($1000) Size Years Error Total DF 3 1 1 1 46 49 Seq SS 34255444 16703393 17478430 73620 8297619 42553062 Seq MS 11418481 16703393 17478430 73620 180383 F-Value 63.30 92.60 96.90 0.41 P-Value 0.000 0.000 0.000 0.526 Since p-value for the general model is less than 0.05, the model is significant. All the predictors significantly contribute to the model apart from year as its p-value=0.526>0.05 13. Perform the t-test on each independent variable. Explain your conclusions and clearly state how you should proceed. In particular, which independent variables should we keep and which should be discarded. Coefficients Term Constant Income ($1000) Size Years Coef 1276 32.27 346.9 7.9 SE Coef 274 4.35 36.0 12.3 T-Value 4.66 7.42 9.63 0.64 P-Value 0.000 0.000 0.000 0.526 VIF 1.10 1.07 1.0 Income and Size significantly contribute to the model but years does not as its pvalue=0.526>0.05 Therefore keep income and size. Discard Years. 14. Is this multiple regression model better than the linear model that we generated in parts 110? Explain. Yes, since 80.50% of credit balance is explained by new model as compared to only 56.62% in the previous model Summary of Report Family size can definitely have a big impact on your credit balance. Family income is also a good predictor of credit balance, but years in their current home was not as helpful. When tests were run to see if credit balance went up when the family size when up, one can find the data to be very related. For each new family member added, the credit balance of the family was shown to go up by $403.20. This report showed that using family size alone, in this set of customers, one can predict their credit balance for a family of size 5 within a few thousand dollars, $3337.90 to $5877.20 to be exact. The statistical tests used showed that the probability of this relationship being by chance was extremely low (less than 0.1 %). However, statistical testing showed that family size along only explained about half (56.62%) of the variability in credit balance was explained by family size. That leads one to wonder, what other variables could affect credit balance? Next, statistical tests were run where we added the additional dimensions of family income and years in their current home. The family income helped a lot to explain the credit balance, but years in their current home was not as helpful. For every $1000 increase in family income, $32.27 was added to the credit balance. The statistical tests used showed that the probability of this relationship being by chance was extremely low (less than 0.1 %). Maybe people who earn more money feel comfortable holding more of a credit balance. With the addition of family income, we are not able to explain most (80.50%) of the variability in credit balance. Years in the current home was tested and it was determined that it did not predict the increase in credit balance statistically well, so it most likely should not be used in determining credit balance. The statistical test for years in the family home showed that its relation to credit balance could be entirely by chance. In conclusion, family size and family income level are good predictors of a family's credit balance, but years in their current home is not much of a help in this prediction. 1. Generate a scatterplot for CREDIT BALANCE vs. SIZE, including the graph of the "best fit" line. Interpret. Scatterplot of Credit Balance($) vs Size 6000 Credit Balance($) 5000 4000 3000 2000 1 2 3 4 5 6 7 Size Plots are upward sloping and fairly aligned almost in a straight lined which suggest of fairly positive linear relationship between variables 2. Determine the equation of the "best fit" line, which describes the relationship between CREDIT BALANCE and SIZE. Coefficients Term Constant Size Coef SE Coef T-Value 2591 195 13.29 403.2 50.9 7.91 P-Value 0.000 0.000 VIF 1.00 Model Summary S 620.162 R-sq 56.62% R-sq(adj) 55.71% R-sq(pred) 53.02% Pearson correlation of Credit Balance($) and Size = 0.752 P-Value = 0.000 Regression Equation Credit Balance($) = 2591 + 403.2 Family Size Since the slope is positive, the relationship is positive. One unit change in price increases Credit balance by $ 403.2 Credit balance is equal to 2591 when size is equal to zero 3. Determine the coefficient of correlation. Interpret. Correlation: Size, Credit Balance($) Pearson correlation of Family Size and Credit Balance($) = 0.752 R= 0.752 This is a strong positive correlation/relationship between these two variables 4. Determine the coefficient of determination. Interpret. R2=0.5662 This means 56.62% of variation in credit balance is explained by family size. 5. Test the utility of this regression model (use a two tail test with =.05). Interpret your results, including the p-value. H0: Model is NOT significant Ha: Model is significant Regression Analysis: Credit Balance($) versus Family Size Analysis of Variance Source Regression Size Error Lack-of-Fit Pure Error Total DF 1 1 48 5 43 49 Adj SS 24092210 24092210 18460853 2499467 15961386 42553062 Adj MS 24092210 24092210 384601 499893 371195 F-Value 62.64 62.64 P-Value 0.000 0.000 1.35 0.263 P-value=0.000 Since p-value<0.025 we reject H0 and conclude that the general model is significant 6. Based on your findings in 1-5, what is your opinion about using SIZE to predict CREDIT BALANCE? Explain. It will be okay to use size to predict credit balance since from 1-5, it has been proven that there is linear relationship between this two variables. However, considering that size has only 56.62% effect on variation is credit balance, other variables that affect credit balance should be incorporated. 7. Compute the 95% confidence interval for 1 (the population slope). Interpret this interval. (300.8, 505.7) This means that we are 95% confidence that the slope will be within this interval. 8. Using an interval, estimate the average credit balance for customers that have household size of 5. Interpret this interval. (3337.9, 5877.2) This means that we can be 95% confident that the customers that have household size of 5 will have an average credit balance that is within this interval. 9. Using an interval, predict the credit balance for a customer that has a household size of 5. Interpret this interval. (3331.6, 5873.6) This means that we can be 95% confident that the credit balance for a chosen customer that has a household size of 5 will be within this interval. 10. What can we say about the credit balance for a customer that has a household size of 10? Explain your answer. No, Since 10 is out of range of the values used as predictor as the maximum value used is 7, predicting credit balance at size 10 will be inaccurate. In an attempt to improve the model, we attempt to do a multiple regression model predicting CREDIT BALANCE based on INCOME, SIZE and YEARS. 11. Using MINITAB run the multiple regression analysis using the variables INCOME, SIZE and YEARS to predict CREDIT BALANCE. State the equation for this multiple regression model. Coefficients Term Constant Income ($1000) Size Years Coef 1276 32.27 346.9 7.9 SE Coef 274 4.35 36.0 12.3 T-Value 4.66 7.42 9.63 0.64 P-Value 0.000 0.000 0.000 0.526 VIF 1.10 1.07 1.07 Model Summary S 424.715 R-sq 80.50% R-sq(adj) 79.23% R-sq(pred) 77.15% Regression Equation Credit Balance($) = 1276 + 32.27 Income ($1000) + 346.9 Size + 7.9 Years 12. Perform the Global Test for Utility (F-Test). Explain your conclusion. Analysis of Variance Source Regression Income ($1000) Size Years Error Total DF 3 1 1 1 46 49 Seq SS 34255444 16703393 17478430 73620 8297619 42553062 Seq MS 11418481 16703393 17478430 73620 180383 F-Value 63.30 92.60 96.90 0.41 P-Value 0.000 0.000 0.000 0.526 Since p-value for the general model is less than 0.05, the model is significant. All the predictors significantly contribute to the model apart from year as its p-value=0.526>0.05 13. Perform the t-test on each independent variable. Explain your conclusions and clearly state how you should proceed. In particular, which independent variables should we keep and which should be discarded. Coefficients Term Constant Income ($1000) Coef 1276 32.27 SE Coef 274 4.35 T-Value 4.66 7.42 P-Value 0.000 0.000 VIF 1.10 Size Years 346.9 7.9 36.0 12.3 9.63 0.64 0.000 0.526 1.07 1.0 Income and Size significantly contribute to the model but years does not as its pvalue=0.526>0.05 Therefore keep income and size. Discard Years. 14. Is this multiple regression model better than the linear model that we generated in parts 110? Explain. Yes, since 80.50% of credit balance is explained by new model as compared to only 56.62% in the previous model Summary of Report Family size can definitely have a big impact on your credit balance. Family income is also a good predictor of credit balance, but years in their current home was not as helpful. When tests were run to see if credit balance went up when the family size when up, one can find the data to be very related. For each new family member added, the credit balance of the family was shown to go up by $403.20. This report showed that using family size alone, in this set of customers, one can predict their credit balance for a family of size 5 within a few thousand dollars, $3337.90 to $5877.20 to be exact. The statistical tests used showed that the probability of this relationship being by chance was extremely low (less than 0.1 %). However, statistical testing showed that family size along only explained about half (56.62%) of the variability in credit balance was explained by family size. That leads one to wonder, what other variables could affect credit balance? Next, statistical tests were run where we added the additional dimensions of family income and years in their current home. The family income helped a lot to explain the credit balance, but years in their current home was not as helpful. For every $1000 increase in family income, $32.27 was added to the credit balance. The statistical tests used showed that the probability of this relationship being by chance was extremely low (less than 0.1 %). Maybe people who earn more money feel comfortable holding more of a credit balance. With the addition of family income, we are not able to explain most (80.50%) of the variability in credit balance. Years in the current home was tested and it was determined that it did not predict the increase in credit balance statistically well, so it most likely should not be used in determining credit balance. The statistical test for years in the family home showed that its relation to credit balance could be entirely by chance. In conclusion, family size and family income level are good predictors of a family's credit balance, but years in their current home is not much of a help in this prediction. Reliable Housewares Summary Report Thank you for providing the data for 50 of your \"credit\" customers, it was very helpful in allowing the analysis of these customers. The following sections will break down your suppositions regarding the purchasing behavior of your \"credit\" customers. Your beliefs were partially correct and each section below will detail the statistical reasoning behind the truth or error of these beliefs. Section A You are pretty sure that the average income of your \"credit\" customers is less than $50,000. You are correct! The data provided showed that the customer's average income is actually $43,740. This is statistically significantly (that means a lot) below the $50,000 that you believed. In fact, we can be pretty sure, 95% confident in fact, that their incomes range from $39,580.00 to 47,900.00. A few of the customers, 19 to be exact, have incomes greater than $50,000, but the overall average is as you suspected. The 10 customers with incomes less than $30,000 pull the average down to where you suspected. Section B You are pretty sure that the fraction of your customers who live in an urban area is greater than 40%. Sorry, wrong on this one. The data provided showed that the number of customers that live in an urban area is 22. That is 44% of the 50 customers in the provided data. Sadly, this is not statistically significantly above the 40% that you believe. In fact, we can be 95% confident that they true percentage lies between 30.24% and 57.76%. That low end of 30.24% makes it just low enough so that we can't be sure, based on the data provided, that your belief on the customers is correct. Section C You are pretty sure that the average number of years that your customers have lived in their current homes is less than 13 years. Sorry, wrong again! The data provided showed that the customer's average year in their current home is 12.26 years. While is number is less than 13 years, is it just not statistically significantly below the 13 years that you thought. We can be 95% confident that their years in their current homes range from 10.82 to 13.71 years. That 13.71 top end is enough to make one not sure enough to back up your belief. Close, but just not quite. Section D You are pretty sure that the average credit balance for your suburban customers is more than $4,300. This one you got right! The data provided showed that the suburban customer's average credit balance is a whopping $4,675.33. This is statistically significantly above the $4,300 that you thought. For your information, the 95% confidence range of credit balances for your suburban customers is $4,264.00 to $5,086.00. While 5 of your 15 suburban customers have a balance of less than $4,300, the bigger balances bring the overall average up to where you believed that it was. Great job! You were right 50% of the time about your customers, and most importantly, right about the financial issues that they may have. A low income and high credit balance are good indicators to keep an eye one going forward. Appendix A 1. Null hypothesis (H0): $50,000 (Average annual income of credit customers is more than $50,000.) 2. Alternative hypothesis (Ha): < $50,000 (Average annual income of credit customers is less than $50,000.) (The claim) 3. Test statistic: z= x x x 43.74050 = = = x s 14 .639 6 -3.0236 n n 50 4. Rejection region: z < -1.645, which corresponds to = 0.05. 5. Conclusion: The sample mean lies 3.02 sample standard deviations below the hypothesized value of $50,000. Since this value of z exceeds (is much less than) -1.645, it falls into the rejection region. That is, we reject the null hypothesis that $50,000 and conclude that < $50,000. Thus, it appears that the average annual income of credit customers is less than $50,000. Confidence Interval: Variable Income ($1000) N 50 Mean 43.74 StDev 14.64 SE Mean 2.07 95% CI (39.58, 47.90) Minitab Output: One-Sample Z: Income ($1000) Test of = 50 vs < 50 The assumed standard deviation = 14.639 Variable Income ($1000) N 50 Mean 43.74 StDev 14.64 SE Mean 2.07 95% Upper Bound 47.15 Z -3.02 P 0.001 Appendix B 1. Null hypothesis (H0): p 0.4 (The true population proportion of credit customers who live in an urban area is less than or equal to 40%.) 2. Alternative hypothesis (Ha): p > 0.4 (The true population proportion of credit customers who live in an urban area greater than 40%.) (The claim) z= 3. Test statistic: ^p p0 p0 (1 p 0) n = .44.4 = .4 (1.4 ) .5773 50 4. Rejection region: z > 1.645, which corresponds to = 0.05. 5. Conclusion: The sample proportion lies .5773 sample standard deviations above the hypothesized value of p 0.4. Since this value of z does not exceeds 1.645, it does not fall into the rejection region. That is, we do not reject the null hypothesis that p 0.4. Thus, the true population proportion of credit customers who live in an urban area is less than or equal to 40%.) Minitab Output: Test and CI for One Proportion Sample 1 X 22 N 50 Sample p 0.440000 95% CI (0.302411, 0.577589) Test of p = 0.4 vs p > 0.4 Sample 1 X 22 N 50 Sample p 0.440000 95% Lower Bound 0.324532 Using the normal approximation. Z-Value 0.58 P-Value 0.282 Appendix C 1. Null hypothesis (H0): 13 (The average number of years lived in the current home is greater than or equal to 13 years.) 2. Alternative hypothesis (Ha): < 13 (The average number of years lived in the current home is less than 13 years.) (The claim) z= 3. Test statistic: x x x 12.2613 = = = x s 5.086 -1.028 n n 50 4. Rejection region: z < -1.645, which corresponds to = 0.05. 5. Conclusion: The sample mean lies 1.028 below the hypothesized value of 13. Since this value of z does not exceed (is less than) -1.645, it does not fall into the rejection region. That is, we do not reject the null hypothesis that 13. Thus, it appears that the average number of years lived in the current home is greater than or equal to 13 years. Confidence Interval: Variable Years N 50 Mean 12.260 StDev 5.086 SE Mean 0.719 95% CI (10.815, 13.705) Minitab Output: One-Sample Z: Years Test of = 13 vs < 13 The assumed standard deviation = 5.086 Variable Years N 50 Mean 12.260 StDev 5.086 SE Mean 0.719 95% Upper Bound 13.443 Z -1.03 P 0.152 Appendix D 1. Null hypothesis (H0): $4,300 (The credit balance for suburban customers is less than or equal to $4,300.) 2. Alternative hypothesis (Ha): > $4,300 (The credit balance for suburban customers is greater than $4,300.) (The claim) 3. Because there are only 15 suburban customers, (n < 30) we must use the t test. The degrees of freedom (df) is one less than the number of customers (n - 1) = 14. Test statistic: t= x x x 4675.334300 = = = x s 742.365 3.575 n n 50 4. Rejection region: t > 1.96, which corresponds to = 0.05 for a one-tailed t test with 14 degrees of freedom. 5. Conclusion: The sample mean lies well above the hypothesized value of $4,300. Since the calculated value of t exceeds 1.96, it falls into the rejection region. That is, we reject the null hypothesis that $4,300 and conclude the credit balance for suburban customers is greater than $4,300. Thus, it appears that the credit balance for suburban customers is greater than $4,300. Confidence Interval: Variable Credit Balance($) N 15 Mean 4675 StDev 742 SE Mean 192 95% CI (4264, 5086) Minitab output: One-Sample T: Credit Balance($) Test of = 4300 vs > 4300 Variable Credit Balance($) N 15 Mean 4675 StDev 742 SE Mean 192 95% Lower Bound 4338 T 1.96 P 0.035 Reliable Housewares Summary Report Thank you for providing the data for 50 of your \"credit\" customers, it was very helpful in allowing the analysis of these customers. The following sections will break down your suppositions regarding the purchasing behavior of your \"credit\" customers. Your beliefs were partially correct and each section below will detail the statistical reasoning behind the truth or error of these beliefs. Section A You are pretty sure that the average income of your \"credit\" customers is less than $50,000. You are correct! The data provided showed that the customer's ave
Step by Step Solution
There are 3 Steps involved in it
Step: 1
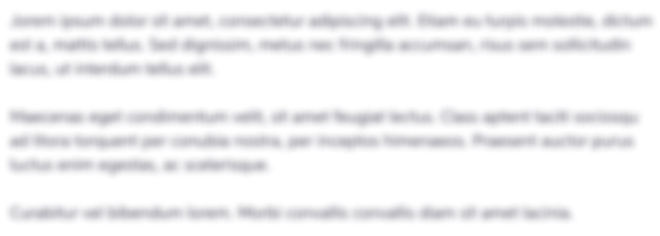
Get Instant Access to Expert-Tailored Solutions
See step-by-step solutions with expert insights and AI powered tools for academic success
Step: 2

Step: 3

Ace Your Homework with AI
Get the answers you need in no time with our AI-driven, step-by-step assistance
Get Started