Answered step by step
Verified Expert Solution
Question
1 Approved Answer
Read the above passage and then answer short questionsThe research method of this paper can be further upgraded and changed. Could you give a general
Read the above passage and then answer short questionsThe research method of this paper can be further upgraded and changed. Could you give a general explanation?
Application Research Based on Machine Learning in Network Privacy Security Abstracts the hottest frontier technology in the field of artificial intelligence, achine learning is subverting various industries step by step. In the future, it will penetrate all aspects of our lives and become an indispensable technology around us. Among then, network security is an area where nachine learning can show off its strengths. Anong nang network securityproblems, privacyprotection is a nore difficult problem, so it needs nore introduction of ner technologies, ner nethods and nev ideas such as rachine learning to help solve some problems. The research contents for this include four parts: an overview of achine learning, the significance of machine learning in network security, the application process of rachine learning in network security research, and the application of rachine learning in privacy protectio. It focuses on the issues related to privacy protectia and proposes to combine the rost advanced matching algorithm in deep learning nethods with infonation theory data protection technology, so as to introduce it into biometric authenticatia. Thile ensuring that the loss of natching accuracy is viniral, a high standard privacy protection algorith is concluded, which enables businesses, goverment entities, and end users to wore videly accept privacy protection technology. Leyvords-tachine learning: Detvort security: privacy protectie I. INTRODUCTION With the development and advancement of information technology, humans have become extremely efficient in work, study, and communication, but at the same time, people's definition of privacy and security is constantly being refreshed. Your online shopping history will reveal your spending habits; your Weibo will tell the world about your lifestyle: your circle of friends and contacts will expose your personal network: your emails and text messages will reveal your working status. The App on your mobile phone can locate your location. These problems can be solved well by relying on traditional privacy protection technolog. For example, in biometrics technolog, sensitive information used to identify the user's identity is very likely to be the target of attack, but the technical optimization carried out on top of this enhances its security, but it makes the protected user's information match accurately the degree is reduced. This will undoubtedly bring a bad user experience and make the technology difficult to popularize. Behind the problem is that the existing privacy protection algorithm has serious defects. In recent years, with the rise of the field of artificial intelligence, the introduction of machine learning technology has brought new hope to many industries that have entered the bottleneck. Network security is an important field that benefits a lot. Machine leaming technology is deeply applied to privacy protection. Relevant problems will provide new thinking for solving the problem of mutual restriction of matching accuracy and security in biometric authentication. II. MACHINE LEARNING OVERVIEW 4. Introduction Machine learning is a branch of artificial intelligence. Artificial intelligence is committed to creating machines that can perform complex tasks better than humans. These tasks usually involve judgment, strategy, and cognitive reasoning [1]. These skills were initially considered to be the "forbidden zone" of machines. Compared with manual programming, machine learning systems automatically learn programs from data, which is very attractive. In the past two decades, machine learning has rapidly spread in computer science and other fields, and is widely used in web search, spam filtering, recommendation systems, advertising, credit evaluation, fraud detection, and stock trading. B. Basic principles Machine learning uses example data or past experience to train computers to optimize computer performance standards. When people cannot directly write computer programs to solve specific problems, but need to rely on instance data or experience, they need machine learning. The real world is always regular. Machine learning is to automatically discover rules from known instances, establish a prediction model for unknown instances and continuously improve and improve prediction performance based on experience. The so-called "learning" is actually model training. To put it more bluntly, a conclusion is derived based on some known conditions. This conclusion is a function, some parts of the function are a constant, but the constant itself is not known [2]. We need to analyze massive data to further infer these missing constants. III. THE SIGNIFICANCE OF THE APPLICATION OF MACHINE LEARNING IN NETWORK SECURITY The significance of the application of machine learning in network security is mainly reflected in the fact that machine learning relies on its own powerful data analysis capabilities. At the same time of application, it can help users respond to network security events in a timely and effective manner. Especially in the case of insufficient security skills of the team, you can set up automatic execution to perform some trivial system security tasks instead of the team, which is conducive to effectively protect the user's network security. In addition, the integration of machine learning and traditional electronic technology products helps to remove malware from products, thereby achieving the improvement of product safety factor and operational stability [3]. Based on the field of network security, machine learning and deep learning are indispensable components of network security technology, especially deep learning, has become the focus of attention in the field of information security. Moreover, in the field of network information security technology, the entire industry takes deep learning to provide solutions for cyberspace security as an important starting point, applying in areas unknown attacks, real-time detection, response to system vulnerabilities and threats. With the development of information technology, attack behaviors are also constantly upgraded and changed. Therefore, machine learning and deep learning have more advantages and development potential in responding to attacks and maintaining network security, which is also the trend and direction of the development of the entire cyberspace security field. The application of machine learning, neural network and other technologies in the field of network security has a positive significance for improving the security of the network and reducing the maintenance cost of the network. The combination of artificial intelligence and other traditional industries has already yielded many fruitful results. The application of machine learning technology to the field of network security will surely bring new development directions to network security technology and lead network security to a new stage. In addition, the means of using artificial intelligence technology to conduct cyber attacks has also appeared In the future, cyber attacks and defenses will be a competition between the two parties' artificial intelligence technology. Therefore, it is beneficial and necessary to apply machine learning to network security, and machine learning will inevitably make great progress in network security technology [4]. IV. APPLICATION PROCESS OF MACHINE LEARNING IN NETWORK SECURITY RESEARCH Machine learning obtains known attributes from large amounts of data to solve problems such as classification, clustering, and dimensionality reduction. As shown in Figure 1, the general application process of machine learning in cyberspace security research mainly includes six stages of security problem abstraction, data collection, data preprocessing and security feature extraction, model construction, model verification, and model effect evaluation. In the entire application process, each stage camot exist independently, and there is a certain relationship between them. According to the logical sequence, the first step is to abstract the security problems in cyberspace into category objects that machine learning can handle through mapping. Such category objects usually refer to classification, clustering, and dimensionality reduction. The second step is to use Wireshark, Netflow, log collection tools and other means to collect a large amount of effective data needed for machine learning algorithms from the three levels of system, network and application. In the third step, after cleaning and processing the collected original data, the preprocessing optimization of the missing, abnormal, unbalanced and undivided data set data is completed. Machine learning is used to prepare the data set, and the training set, validation set and test set are sorted out. Finally, the attributes with the most security problems in cyberspace are extracted to complete the extraction of security features. The fourth step is the central link in the entire process. This link selects the most appropriate machine learning algorithm based on the data set after data pre processing, and completes the adjustment and optimization of parameters. The fifth step isto verify the trained model through multiple verification methods such as k-fold cross-validation to confirm whether the model is stable and effective enough. The sixth step is to test the learning effect and generalization ability of the machine learning model by evaluating the test set. Of these, different evaluation indicators are usually used for the evaluation of the effects of different categories and different fields. Seourite data collection Data Model building ing Data reason Model GBUB evaluati No Yes Figure 1 Biometric template protection algorithm V. APPLICATION OF MACHINE LEARNING IN PRIVACY PROTECTION 4. Introduction In view of the large number of digital footprints we leave every day, privacy is gaining more and more attention in today's world. The form of privacy may be the information we registered in the forum, photos or videos uploaded to social media sites, or even our historical browsing records. Some parts of these footprints have a positive nature. It is information that users willingly provide to third parties, but surprisingly, a large part of our digital footprint belongs to our digital shadow. The digital shadow is essentially a subset of the digital footprint that we may inadvertently leave behind. A large part of this information is sensitive, and some people worry that it may fall into the wrong hands. Modem encryption technology does a good job of maintaining certain forms of sensitive information, but some data types and applications are still difficult to protect. B. Sensitive information Sensitive information usually refers to data that must be protected from unauthorized access in order to protect the privacy of individuals or organizations. These can be personal information related to individuals, business information related to organizations, or confidential information related to government agencies. From the perspective of the general public, the focus should be on personal information in the form of personally identifiable information (PII), because this information can be used to track individuals and may cause harm to the safety of the person's personal property. (PII) information in the form of detailed information of social security accounts and bank accounts is generally locked behind the password wall using symmetric encryption technology, but media data such as images and videos are not that simple. In general, the complexity of protecting media privacy information stems from the fact that data storage entities need to access information to provide certain characteristics. For example, user images are automatically tagged on social media sites, or the biometric authentication system needs to access the user's biometrics for future matching. In fact, the biometric data is in full compliance with the data categories that we believe are safe and highly sensitive but difficult to obtain 6 Biometrics Biometrics technology is a technology that uses computers to utilize the inherent physiological or behavioral characteristics of the human body for identity authentication Behavior recognition technology is expressed through our long-term habits, such as our signature, gait, or the way we hit the keyboard. Generally, physiological biometrics are considered "hard" biometrics and are more suitable for use alone, while behavioral biometrics are considered 'soft" biometrics and are often used in conjunction with other forms of authentication Fingerprints, irises, human faces, veins, and other biological characteristics are inherent in human beings, and they are unique and can be used to accurately identify a person s identity. At the same time, compared with the password input, biometrics has the characteristics of convenient verification. However, these biometri are few in number and cannot be changed, and there is a security risk if they are leaked With the application of biometric authentication technologies such as fingerprint recognition, face recognition, and speech recognition, attack pattems against such technologies will continue to emerge. D. Protection of feature templates The goal of biometric template protection is to protect biometric data in a manner similar to protecting text passwords. Since the original www biometric data cannot be changed like a text password, its security is critical. The ideal biometric template protection algorithm should have the following characteristics. Given a protected template, it should not be feasible to extract its original biometric data. If the old template is damaged, a new protection template can be generated from the original biometrics. 3. Performance. The template protection algorithm should not cause the loss of matching performance of the biometric system. It is worth noting that the template protection algorithm usually results in a loss of matching accuracy compared with the algorithm using template unprotected. This leads to a trade-off between template security and matching accuracy of biometric authentication algorithms. From a security point of view, the cryptographic hash function is an ideal choice for biometric template protection algorithms, but certain attributes of biometric data make the security based on hashing methods a great challenge. Hash-based security requires precise matching between templates extracted by reading different data from biometrics. Because biometric data shows significant intrinsic differences between readings, it is very difficult to extract a template that matches exactly. Even a single bit change will cause the extracted hash value to be very different. In addition, since the biometric data camot be changed when the hash value is destroyed, it needs to be cancelled in some way. Although there are difficulties in achieving the security of hash-based biometric data templates, several methods have been proposed. As shown in Figure 2, biological template protection algorithms can be roughly divided into two categories: biometric password systems and basic feature conversion. Template protection Basic feature conversion Biometric cryptosystem Salting out Key binding Irreversible transformation Key generation Figure 2 Biometric template protection algorithm a) Local area hash (LR) The principle behind the local area hashing algorithm is to evaluate the suitability of the feature extractor for the protection of facial biometric templates based on cryptographic hashing. It is assumed that even if the template protection algorithm based on password hashing camot accurately match the features extracted from the entire face, it is much easier for the features extracted from the local area of the face. By comparing the features of local binary pattem, directed gradient histogram and self-encoder stack learning, it is found that artificial feature extraction information such as LBP and HOG is even more than the simplest representation learning Oops, this has facilitated the study of representation learning with both matching accuracy and template security. The advantage of this algorithm is that no user data is stored, no external key is used, and the features used are not user-specific. The disadvantage is that due to the higher template security, the loss of matching accuracy and the unevemess of the feature space are caused In short, the algorithm is a preliminary study of the problem, which lays the foundation for a better solution to the problem. b) Deep Secwity Coding The main purpose of the deep security coding algorithm is to directly solve the uniformity problem. It is designed with an ideal template security function and uses deep convolutional neural network to learn its mapping, and then complete the predetermined goal. The ideal feature is a randomly generated user code, which we call the maximum entropy binary code. Since these are randomly generated bit by bit, they have the maximum entropy, which makes the search space for brute force attacks on hash codes very large. Once CNN is trained to learn the mapping from user's registered samples to MEB codes, its performance will be generalized to generate the same code for other samples of registered users. The algorithm has high template security, and achieves the most advanced matching performance when the fault tolerance rate (FAR) 95%. The advantages of this algorithm include: the uniform feature space of the hash, the most advanced template security and matching accuracy, and the increase in the theoretical relationship between the template security and matching accuracy in the same direction. The main disadvantage of this algorithm is that CNN only trains for registered users, and cannot be extended to new users. Therefore, the new user needs to retrain the neural network when registering. c) Deep Random Hashing The deep random hashing algorithm was bom to solve the problem of retraining when new users register in the deep secure coding algorithm The idea is to learn to use deep CNN's class-independent binary encoder, and then use a cryptographic hash function to hash this code to produce a protected template. This algorithm enables people to determine the required attributes from the learned binary representations, and focuses on the ability of neural network generation to be extended to new class representations without retraining. The idea of deep security coding is to design a three part loss function that takes into account each ideal attribute of representation, namely class separation, uniformity, and resistance to mountain-climbing attacks. Its three parts include the traditional "TripletLoss" loss function, as well as norruniform and random edge parts. The experiments conducted compared the performance of deep random hashing and deep secure coding, and the representation form learned between the various components of the three part loss function in deep random hashing, and found that the proposed loss function can indeed be extended to Category, and learned the representation form suitable for our purpose. However, the improvement of evaluation indicators is insignificant, which indicates that a larger data pool may need to be further tested. This work, as a preliminary study in the future, laid the foundation for privacy protection matching in the relatively new representation learning field. E. Practical significance In summary, the advantages of the latest progress in the field of deep learning are introduced into the field of biometric template protection, which opens up a relatively new field of representation learning for privacy protection matching. The proposed algorithm "Deep Security Coding" has achieved the most advanced matching performance and facial template security, and highlights the declared validity. These discoveries use other forms of deep learning algorithms to extend algorithm ideas to new biometric recognition technologies, and bring new opportunities to the development of biometric template protection. Such basic research emphasizes the protection of user data, and at the same time minimizes the overhead related to reducing the matching accuracy, which is beneficial to many aspects. While providing protection for templates, the decline in matching accuracy is one of the main reasons that prevent applications for biometric authentication such as face or fingerprint unlocking from accepting higher standards of template protection. The development of algorithms that implement verifiable template security standards whileminimizing the loss of matching accuracy not only encourages data storage entities to accept higher protection standards of user privacy, but also does not reduce the quality of service they provide. In addition, the above algorithm does not require any secret key or hidden information about the intemal work of the algorithm, so it can be made public. Doing so can increase the user's trust in these technologies, because they can effectively protect their data, making the technology that matches the user's sensitive data more widely accepted. Finally, the application of the algorithm goes beyond the field of biometric authentication, and the concepts introduced above can be used to protect various privacy matches. Perhaps this research can arouse people's interest in new areas that have not considered privacy protection but may have important significance in the near future, such as automatic facial tagging, picture passwords, web browsing pattem analysis, sentiment analysis, etc. VI. CONCLUSION This article takes privacy protection as an example to explain in depth the application of machine learning in network security. First it introduced the relevant concepts and basic principles of machine learning, secondly it stated the importance of the application of machine learning in network security, then it combed the application process of machine learning in network security research, and finally it analyzed in detail in the field of privacy protection Overcoming the problem of mutual constraint between security and matching accuracy in biometric recognition through machine learning, and summarized three algorithms to solve such problems. What is certain is that in the field of network security other than privacy protection, machine learning can also help solve related problems. In the future, machine learning will be able to play a bigger role in the field of network security, and even the mainstay of the role! REFERENCES: [1] Zhou Zhihua. Machine leaming LM. Beijing: Tsinghua University Press, 2016: 1. [2)Peng Yanxin, Machine learning, deep teaming and network security technology Lj]. Computer Products and Circulation, 2018 (4): 66. [3] Liu Jinpeng. Network security protection based on machine learning technology 1). Cyberspace Security, 2018, y (9): 46-102. [4] Zhang Lei, Cui Yong, Li Jing, Jiang Yong, Yu JiapingApplication of machine leaming in cyberspace security research Lj. Joumal of Computer Science, 2018, 41 (V): 1943-1975. 9Step by Step Solution
There are 3 Steps involved in it
Step: 1
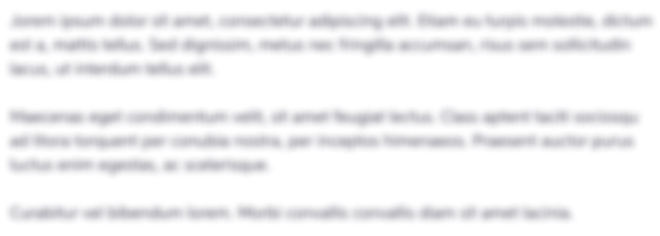
Get Instant Access to Expert-Tailored Solutions
See step-by-step solutions with expert insights and AI powered tools for academic success
Step: 2

Step: 3

Ace Your Homework with AI
Get the answers you need in no time with our AI-driven, step-by-step assistance
Get Started